Abstract
Previous studies discovered a spatially heterogeneous expansion of Siberian larch into the tundra of the Polar Urals (Russia). This study reveals that the spatial pattern of encroachment of tree stands is related to environmental factors including topography and snow cover. Structural and allometric characteristics of trees, along with terrain elevation and snow depth were collected along a transect 860 m long and 80 m wide. Terrain curvature indices, as representative properties, were derived across a range of scales in order to characterize microtopography. A density-based clustering method was used here to analyze the spatial and temporal patterns of tree stems distribution. Results of the topographic analysis suggest that trees tend to cluster in areas with convex surfaces. The clustering analysis also indicates that the patterns of tree locations are linked to snow distribution. Records from the earliest campaign in 1960 show that trees lived mainly at the middle and bottom of the transect across the areas of high snow depth. As trees expanded uphill following a warming climate trend in recent decades, the high snow depth areas also shifted upward creating favorable conditions for recent tree growth at locations that were previously covered with heavy snow. The identified landscape signatures of increasing tall vegetation, and the effects of microtopography and snow may facilitate the understanding of treeline dynamics at larger scales.
Export citation and abstract BibTeX RIS
A correction was made to this article on 6 Jan 2022. The author list was re-ordered as it was not amended pre-publication.
1. Introduction
The Arctic terrestrial ecosystems have been changing in response to the global warming (Hinzman et al 2005) over the past decades. Numerous studies have reported shrubification and tree expansion into tundra ecosystems towards higher latitudes and areas of higher elevation (Sturm et al 2001, Tape et al 2006, Forbes et al 2010, Myers-smith et al 2011, Elmendorf et al 2012, Frost and Epstein 2014). The process has been proliferating and is well documented for many places in the Arctic from both direct in situ observations, dendrochronological analyses (Lloyd and Fastie 2002, Shiyatov et al 2007, Devi et al 2008, Van Bogaert et al 2011, Martin et al 2017) and satellite-based observations (Lloyd et al 2011, Frost et al 2014, Mathisen et al 2014). With a warming trend projected for the coming decades, the change of tundra plants at the circumpolar scale is expected to continue in the future (Bjorkman et al 2018), including both structural shifts from low to high shrubs or trees (Forbes et al 2010, Macias-fauria et al 2012) and migration-based treeline advances (Macdonald et al 2008, Vavrus et al 2012, Koenigk et al 2013).
The observed replacement of short tundra vegetation (graminoids, forbs, and non-vascular vegetation) to tall erect woody plants (shrubs and trees) are thought to be induced mainly by increasing temperature (Walker et al 2006, Elmendorf et al 2012), along with the impacts of other important drivers like precipitation, soil moisture, snow dynamics, and herbivory (Frost and Epstein 2014, Martin et al 2017). This process will likely have positive feedback to climate and enhance warming through changes of albedo, evapotranspiration, and carbon cycle (Lafleur et al 2001, Foley et al 2003, Chapin et al 2005, Pearson et al 2013, Zhang et al 2013, Lafleur and Humphreys 2018). Furthermore, this process can potentially alter wildlife habitat (Tape et al 2016). Forest-tundra boundaries have been found to be temperature-sensitive transitional zones (Esper and Schweingruber 2004, Harsch et al 2009). While treeline location is mainly controlled by summer temperatures and growing season length (Macdonald et al 2008, Hoch and Korner 2009), treeline advances have also been found to be associated with winter warming (Kullman 2007, Hagedorn et al 2014). Although tree growth is considered to be mainly limited by low temperatures (Körner and Paulsen 2004), other environmental conditions also affect tree distribution patterns (Holtmeier and Broll 2005) leading to spatial heterogeneity in treeline advances (Lloyd et al 2002, Wilmking et al 2004).
The northern Siberian region encompasses the largest forest-tundra ecotone in the world (Frost and Epstein 2014) and exhibits large gradients in summer conditions and regional differences in vegetation distribution (Macdonald et al 2008). Previous studies have shown various rates of vegetation expansion across northern Siberia strongly correlated with increased winter precipitation (Frost and Epstein 2014, Devi et al 2020). This can be explained by the impact of winter precipitation on soil hydrological and thermal mechanisms. On the one hand, the increase of winter precipitation may provide extra water supply for seedlings and saplings during the growing season (Grigorieva and Moiseev 2018, Devi et al 2020); on the other hand, snow cover is essential for new trees to survive along the treeline as snowpack increases soil temperature and protects against frost and wind abrasion stresses (Holtmeier and Broll 2007, Kharuk et al 2010a, Hagedorn et al 2014). Landscape features also influence the spatial distribution of forests, thereby mediating their response to ambient warming (Kharuk et al 2010b, Dearborn and Danby 2018). In the areas of complex topography including mountainous regions, tall vegetation would have higher or lower favorability over different microtopographic niches. This preference of vegetation depends on environmental factors such as slope type and snow redistribution, which can lead to spatially varying treeline sensitivity to climate (Holtmeier and Broll 2005). Topography-mediated hydrological conditions such as drought and flooding stresses can also affect the boreal forest response to climate at regional scales (Sato and Kobayashi 2018). Soil nutrient is also a key player in biomass dynamics if the low-temperature limitation of tree growth at the treeline starts weakening in the future (Hagedorn et al 2020). An emerging question is: as the climate is becoming less limiting for the encroachment of tall vegetation, are there other appreciable factors controlling the distribution of trees over the forest-tundra ecotone?
This study aims to analyze spatiotemporal patterns of the larch forest encroachment into tundra and relevant environmental factors with a focus on two features: microtopography and snow cover. We analyze the timberline ecotone in the Polar Urals region where a unique set of records on tree growth dynamics along with environmental features data are available since the 1960s. The 'timberline ecotone' refers to a transitional belt of mountain vegetation between the upper limit of closed forests and the upper limit of single tree growth in the tundra (Körner 2003, Shiyatov et al 2005). We hypothesize that the spatiotemporal pattern of tree distribution of this ecotone is associated with niches of favorability caused by microtopography and snow cover.
2. Materials and methods
2.1. Study site and vegetation
The study region of the Rai-Iz massif is located on the eastern slope of the Polar Urals range (200–350 m asl.), in the Sob' River watershed (ca. 66°46' N, 65°49' E) underlain by the continuous permafrost (figures 1(a) and (c)). The bedrock is mainly comprised of Paleozoic amphibolite and crystal granodiorite (Mazepa et al 2011). The most common soil in the Polar Urals is mountain-tundra gleysol, and rare inclusion of mountain-tundra turf soils. On the ultramafic massif of Rai-Iz, soils are characterized by neutral and near-neutral pH (Kataeva et al 2004). According to data collected at Salekhard meteorological station (55 km southeast of the study area, 35 m asl.) between 1883 and 2005., the mean annual, mean monthly minimum (January) and maximum (July) air temperatures are −6.7°, −24.4°, and 13.8 °C, respectively. The mean annual precipitation is 600 mm with 50% as snow and sleet. The growing season lasts from mid-June to earlyAugust with a mean frost-free period of 94 d. Westerly wind prevails, while northeasterly wind is also present in early summer (see S1 available online at stacks.iop.org/ERL/17/014017/mmedia), with an average wind speed of 1–3 m s−1 and maximum speed observed during the winter season (Mazepa 2005).
Figure 1. Maps of the study area: (a) the permafrost zones (Brown et al 2002) and (b) the bioclimate subzones (Walker et al 2005 in the Polar Urals region. (c) The vicinity of the Rai-Iz massif and the Sob' river watershed, where the studied transect (red polygon) is located. Elevations of hilltops near the transect have been displayed. (d) Outline of the studied transect. The permafrost zones in (a) are classified based on the percentage (as in the legend) of landscape underlain by permafrost. In (d), elevation ranges from 194 (bottom-right) to 257 (top-left) m asl.
Download figure:
Standard image High-resolution imageStarting in the early 1960s, six permanent altitudinal transects of 300–1100 m long and 20–80 m wide were established in the eastern foothills of the mountain range for long-term monitoring of spatiotemporal dynamics of alpine forest-tundra and forest-meadow communities. Transects typically start at the upper timberline boundary, where live trees are not yet present, and extend down into the upper limit of closed forest (Mazepa et al 2011). This study focuses on the earliest ecotone transect within which complete data of tree characteristics, terrain elevation, and snow distribution have been collected for multiple years (figure 1(d)). This transect also exhibits varying topography, as compared to the other transects. The transect is 860 m long and 40–80 m wide (the total area is 5.6 ha, with the upper left corner at 66°48'57'' N, 65°34'09'' E) and oriented along the direction of the predominant westerly wind (Mazepa 2005, Shiyatov and Mazepa 2011).
This transect is located close to the southern boundary of the bioclimate subzone E (Walker et al 2005) as shown in figure 1(b). Tree stand composition within the timberline ecotone is relatively simple: larch (Larix sibirica Ledeb.) forest-tundra communities dominate in the upper part, while open larch forest with Siberian spruce (Picea obovata Ledeb.) and downy birch (Betula tortuosa Ledeb.) occur in the lower part of the area (Shiyatov et al 2005). Dwarf birch (Betula nana L.) is abundant in the lower and middle parts of the transect. Names of plant species correspond to the Integrated Taxonomic Information System (ITIS 2021). Historically, boreal trees grow at the fringe of the forest-tundra ecotones in various growth forms (creeping, prostrate, single-stemmed, and multi-stemmed), which reflect adaptations to environmental changes (Mazepa and Devi 2007, Devi et al 2008). Over 90% of the young trees appearing in the study transect after 1950 are single-stemmed (Mazepa et al 2011). The studied forest stands, and recent vegetation growth have emerged without significant impacts from reindeer grazing, fire, and human activities (such as logging) over at least the last millennium (Mazepa 2005, Devi et al 2020).
2.2. Tree structural and allometric characterization
The records of three census campaigns in 1960, 1999, and 2011 are used in this study to quantitatively assess the changes in the composition, structure, and spatial distribution of the forest-tundra communities. Specifically, detailed mappings of all alive and dead tree locations, measurements of their allometric and geometric characteristics such as height, crown size, branch position, and diameter at multiple heights were produced during these campaigns. All three censuses predominantly recorded larch with the occasional presence of spruce along the study transect. The numbers of trees for the 1960, 1999, and 2011 censuses are 1853, 1700, and 1398, respectively, after pre-screening (see supplementary materials (S2)).
After reconstructing the missing tree diameter data using an allometric function (see S2), we compute the change of tree diameters ΔD between censuses as the proxies for the increase of biomass and tree productivity, and then relate them to environmental features. Specifically, to compare changes of tree diameters ΔD for different periods (i.e. from 1960 to 1999, and from 1999 to 2011), the relative diameter change ΔDrel
= ΔD/D0, where D0 is the tree diameter at the first year of the corresponding period, is computed first. ΔDrel is then normalized by the period of time T (years) to get the annual relative change rate of stem diameter assuming a constant annual change rate for a given period. This rate was computed only for trees recorded both at the start and end year of the time interval between the two censuses. The changes in tree diameter may also be used as a proxy of tree biomass change through its relationship with sapwood (see S2).
2.3. Snow distribution
Snow data were collected along the study transect in 1961, 2006, and 2013 in the spring, when snow depth (ZS) was at its peak by direct measurement, tree marking, and visual assessment (see S3). The relative snow depth, defined as where
and
are the maximum and minimum snow depths within the transect for a given survey year from the three surveys, is shown in figure 2.
Figure 2. The spatial distribution of the relative snow depth in the study transect in 1961 (a), 2006 (b), and 2013 (c). Plot insets show the relative density (bars) and the probability density function of the relative snow depth estimated with kernel density (red curves in the insets) using a Gaussian kernel. The transect was mapped to a relative coordinate frame, where the x and y axes align with the lowermost and leftmost point of the transect, respectively.
Download figure:
Standard image High-resolution image2.4. Topographic analysis
Digital terrain models (DTMs) are needed to capture topography at fine scales, but there are limited areas in the Arctic for which high-resolution DTMs are available. There is a range of digital elevation model (DEM) products that can provide high-resolution elevation maps. However, these DEM products are affected by the presence of surface biomass masking the location of actual topography. To reconstruct the DTM for the transect, we combined a resampled 2 × 2 m resolution grid from a 20 × 20 m regularly spaced field terrain measurements of the transect, and a 2 m resolution DEM product called ArcticDEM (Porter et al 2018). After co-registering two ground sources (i.e. field measurement and ArcticDEM), we manually selected bare ground grids from ArcticDEM together with the field measurement to extract a high-resolution DTM for the study transect by natural neighbor interpolation (Tily and Brace 2006).
Terrain curvature is one of the fundamental topographic properties and an important driver of hydrologic processes (Moore et al 1991). Two types of curvature indices, i.e. profile curvature and planform curvature defined by the Environmental Systems Research Institute (ESRI 2021), are used to represent micro-topographic features in this study. Specifically, profile curvature is the curvature of the surface in the direction of the steepest slope, while planform curvature is the curvature in the plane perpendicular to the direction of the steepest slope. For profile curvature index, a negative value indicates an upwardly convex or dome-shaped surface, while a positive value represents a concave surface or depression; for planform curvature index, negative and positive values indicate a laterally concave and convex surface, respectively. Note that the opposite sign is used in the definition of planform curvature concavity/convexity to that of profile curvature. In conventional terrain analysis, the curvature indices are calculated from DTM as the second-order derivative of elevation map using various geomorphometric methods (e.g. Zevenbergen and Thorne 1987). The curvature indices calculated from the resampled DTM with various resolutions are used to capture topographical features at different scales (see S4).
To attribute topographic locations to niches of higher or lower favorability for tree encroachment, we computed the weighted average curvature index using stem diameter at locations where trees are present as a weight:

where is the diameter of tree i,
is the curvature index of a terrain grid cell where the tree is located, and the summation is for all trees along the transect. The index p represents either planform or profile curvature index. Noted that
as well as the number of trees within a given grid cell change with resolutions, so the summation is not carried out over the same set of trees for different grid resolutions. The above formulation emphasizes the contributions of curvature indices of terrain locations with higher D. The rationale is that stem diameter is argued to be an indicator of biomass and long-term productivity (section 2.2). If there is an enhanced tree growth at topographic locations of specific curvature, it can be elucidated by comparing
in (1) with the average curvature of transect topography as the arithmetic mean of curvature over all grid cells along the transect at different grid resolutions.
2.5. Stem density clustering analysis
Spatial point pattern analysis is commonly used to characterize the patterns of the spatial distribution of tree species (e.g. Condit et al 2000). Most of these analyses use a family of statistics derived from the Ripley's K-function (Ripley 1976) to quantify the species clumping characteristics by comparing them with complete spatial randomness (CSR). In this study, following the approach proposed by Plotkin et al (2002), we apply a density-based spatial clustering method with the presence of noise (i.e. points located too far from other points) (Ester et al 1996) to capture spatial pattern of tree distribution. The advantage of this method is that it does not require a priori number of clusters, such as in k-means analysis (Macqueen 1967), and can identify clusters of arbitrary shape. Clusters of tree locations (referred to as 'points') are derived at different spatial resolutions using the records of the three tree censuses (section 2.2). The algorithm requires two parameters: neighborhood search radius (d) and the minimum number (q) of points required to form a cluster located within the search radius. By setting the parameter q to 1 in all analyses, we make the clustering process depend only on the parameter d, which thus represents the maximum distance between any pair of connected trees belonging to the same cluster. Therefore, for any given value of d all stem locations are partitioned into a unique set of clusters.
Tree clusters were formed at different spatial scales by changing the parameter d from 0 to the maximum distance between locations of any two connected trees in the transect (65 m). For a given d, the total number of obtained clusters is denoted as m and the size of an ith cluster as ci
. The sample size, i.e. the total number of trees in the transect, is denoted as . The unique arrangement of clusters for a specific value of d can be summarized with the normalized mean cluster size:
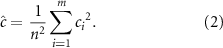
This variable represents the probability that two randomly chosen tree locations belong to the same cluster (Plotkin et al
2002). For example, when
, corresponds to a clustering pattern that each point forms its own cluster;
when d equals the maximum pairwise distance between any two connected tree locations, i.e. all trees form a single cluster. The clustering pattern varies for intermediate values of d and can be obtained by recording the concomitant change of
, known as the mean cluster size curve
. To make the results for censuses with different sample sizes comparable in the mean cluster size curves, we use the normalized distance
defined as

where A is the transect area. Spatial patterns of clustering can then be compared with CSR (see S5).
3. Results
3.1. Stem diameter change
The sampling distribution of the annual relative change rate of stem diameter for the two periods between the three censuses, (1960–1999 and 1999–2011) is shown in figure 3(a). The mode of the distribution increased from 2%/year to 3%/year, indicating accelerated growth in the later period. The right-skewed distribution of the second period implies that the proportion of trees with higher growth rates is larger in the recent period. Figure 3(b) shows the relationship between
and D0 for the two periods between the three field surveys. The maxima of
form a clear upper boundary with respect to D0 for both periods, indicating the upper limit of the annual growth rate and the results illustrate an increase of these growth rates from the earlier to the later period (red and blue lines). The highest growth rate decreasing with D suggests that larger, older trees grow slower than smaller, younger trees. Growth rates can vary significantly, between just above zero and to a few percent of their stem diameter per year.
Figure 3. (a) The sampling probability density function (pdf) of the annual relative rate of stem diameter change, (a proxy for stem growing rate), for the two periods between field surveys: from 1960 to 1999, and 1999–2011. The blue and red curves are the probability density functions estimated using a Gaussian kernel for 1960–1999 and 1999–2011, respectively. (b) A relationship between the
from 1960 to 1999 (cyan dots) and 1999–2011 (orange dots) and the stem diameter at the start year (D0) for each period. The logarithmic scale is used for the horizontal axis. Blue (1960–1999) and red (1999–2011) lines are the upper boundaries of the change rate. To construct the boundaries, we calculated 95% percentiles of
for bins of D0 (plotted as blue squares and red diamonds) and a linear regression with respect to log(D0) at the center of each bin was fitted.
Download figure:
Standard image High-resolution image3.2. Tree locations and terrain curvature indices
Figure 4 compares the average, D-weighted terrain curvature index for grid cells with live trees (equation (1)) and the transect average index (i.e. uses all grid cells), as a function of DTM grid cell resolution. The results indicate scale-dependent differences with respect to the transect average for both planform and profile curvatures, and for all censuses. The difference is significant for DTM grid sizes ranging from 2 to 14 m, with the largest difference occurring at the grid size of 10 m. This implies the preference of tree growth in terrain curvature is characterized most significantly at this scale (i.e. 25–30 m length scale of landforms, which is the size of the moving window used to compute the curvatures, see S4). At locations with live trees, the profile curvature index is more negative, and the planform curvature index is more positive than the overall transect averages, implying that trees tend to cluster at sites with convex topography. The curvatures of the locations with trees and the transect average become indistinguishable for grid cells of sizes greater than 14 m. Since the number of grids along the transect decreases with grid size, this leads to the convergence of the curvature indices to the same value.
Figure 4. Transect curvature indices as a function of the DTM resolution: (a) average profile curvature index and (b) average planform curvature index computed over all locations with live trees, all censuses (colored curves), for different grid cell sizes. Averaged curvature indices estimated using all grids in the transect are shown with black lines; whiskers represent the 95% confidence interval.
Download figure:
Standard image High-resolution image3.3. Tree cluster dynamics
The resultant cluster size curves for the three censuses are shown in figure 5. The sharp transitions in the three curves at various distances (equation (3)) correspond to the 'continuum percolation' phenomenon (Meester and Roy 1996, Plotkin et al
2002), i.e. the aggregation of isolated smaller clusters into a larger cluster. The corresponding distance is known as the 'critical distance' or 'percolation threshold'. When
is below the threshold, elements in the system of interest are disconnected and form many smaller-sized clusters; above the percolation threshold, the elements are aggregated to form larger clusters. In theory, for true CSR (i.e. if the sample size is infinitely large) the cluster size curve will show a discontinuous transition corresponding to the step function at a critical distance of
(an abrupt transition for the developed CSR curve in figure 5 is not discontinuous due to the finite sample size and transect edge effects on the computation of
).
Figure 5. Normalized mean cluster size curves for the three censuses (colored lines) and the complete spatial randomness (CSR) ensemble set. The CSR curve (black solid line) shows the curve averaged over 1000 members of the CSR ensemble set; the black dashed curves represent the 95% confidence interval for this set. The normalized mean cluster size, , represents the probability that two randomly chosen tree locations fall in the same cluster. While
is the normalized maximum pairwise distance between two connected tree locations (see text for details). The plateaus of the curves between the abrupt transition in cluster sizes represent the ranges of
within which the change of cluster pattern is insignificant. The
corresponding to the start of plateaus is the critical distance.
Download figure:
Standard image High-resolution imageThe cluster size curves constructed using the actual tree locations from all censuses have three transitions at varying critical distance . Their differences with respect to the CSR curve imply a non-random spatial tree distribution at different spatial scales. The plateaus of the curves between the abrupt transition in cluster sizes represent the ranges of d within which the change of cluster pattern is insignificant. The number of plateaus (two for this case) indicates the number of scales of non-random aggregation (Plotkin et al
2002). Therefore, one may perform a clustering analysis using d corresponding to a plateau region to represent such a stable distribution at a selected scale. Here we choose the values of normalized distance corresponding to the start of plateaus immediately after the occurrence of 'continuum percolation'. The following clustering analysis uses
corresponding to the first plateaus (
) in figure 5: 1.24, 1.4, and 1.51 (i.e. the actual distances are d1 = 6.7, 7.9, and 9.4 m) for the 1960, 1999, and 2011 censuses, respectively. For the second plateau (
) in figure 5, the normalized distances are
= 2.37, 3.63, and 5.58 (d2 = 12.8, 20.5, and 34.7 m). When a cluster pattern corresponding to
is defined as the 'primary cluster set', then the value of
is a representative proxy of the distance among trees within any cluster of this set, while
represents a proxy of the distance between borders of clusters formed by the primary set. As figure 5 shows, over time relatively smaller changes of the distribution pattern occurred within the primary cluster set, as reflected by the comparable magnitudes of
across the three censuses. The distances between the cluster borders however become larger, as illustrated by the increase of
, particularly for the census of 2011 (mainly due to the mortality of trees at cluster borders).
Figure 6 shows the spatial distributions of tree clustering for the two critical distances . Transitions of cluster patterns can be detected by comparing plots in the same row. For example, when the distance changes from d1 = 6.7 m to d2 = 12.8 m (the census of 1960), the number of clusters decreases significantly from 102 to 21 due to cluster aggregation. The same 'continuum percolation' phenomenon is observed for the other census data of 1999 and 2011. Comparing plots in the same column reveals that the number of primary clusters decreases over time. This phenomenon can be explained by the disappearance of cluster 'noise', i.e. those trees that form their own cluster. Notice that for the primary cluster set (the left panel of figure 6), the sum of cluster sizes of the three largest clusters is over 80% of the total number of surveyed trees (not shown).
Figure 6. Spatial representation of tree clusters corresponding to the two critical distances (left panel, the first critical distance in figure 5, the actual distance is d1) and
(right panel, the second critical distance in figure 5, the actual distance is d2); m is the corresponding number of clusters. The first, second, and third row corresponds to the censuses in 1960, 1999, and 2011, respectively. Circles represent tree locations and each cluster is plotted with a unique color.
Download figure:
Standard image High-resolution imageFigure 7 illustrates the temporal change of the distribution of the largest cluster from 1960 to 2011. In 1960, there were many small trees scattered in this cluster and larger trees were mainly located in the middle part. A large fraction of small trees previously located in the lower part disappeared in later years, while those in the upper part grew bigger and likely seeded new trees that appeared in the transect in 2011.
Figure 7. Change of spatial pattern of the largest tree cluster (blue circles) identified for the first 'percolation threshold' in figure 6 (left panel) in (a) 1960 and (b) 2011. Circle sizes are scaled by the tree diameters. The color map indicates the distribution of relative snow depth in (a) 1961 and (b) 2013. Letter 'c') in the panels denotes the cluster size, i.e. the number of trees within this cluster.
Download figure:
Standard image High-resolution imageConcurrently with the change of the tree distribution pattern, the area with high snow depth had shifted from the lower part to the middle part of this cluster. Similar transitions of areas with high snow depth are also apparent in the regions outside of this cluster (not shown). The area of high snow accumulation shifted from the lower part to the middle-upper part of the transect, coinciding with the growth and densification of trees in clusters at the top.
4. Discussions
Forest expansion in the Polar Urals region is thought to be induced by the increased summer temperature and winter precipitation (Shiyatov et al 2005, Devi et al 2008, 2020). Analyses of treeline dynamics are usually based on repeated landscape photographs and remotely sensed imagery (Kharuk et al 2006, Beck and Goetz 2011, Frost and Epstein 2014), dendrometric survey on tree remnants (Briffa et al 2008, Shiyatov and Mazepa 2011, 2015), and treeline models (Kaplan and New 2006, Paulsen and Körner 2014). However, long-term records of allometric characteristics of living trees, as well as snow distribution back to the beginning of the satellite era are rare at tree stand level. This study analyzed spatiotemporal characteristics of the encroachment process of Siberian larch into timberline ecotone of Polar Urals between 1960 and 2011. The unique data sets spanning over 50 years allowed us to carry out tree-level characterizations of landscape favorability for the encroachment process and other features of tree expansion.
Various factors influence the spatial distribution of trees in the timberline ecotone. Considering the small scale of this study that spans a ∼1 km-long gentle mountain hillslope, climatic factors such as temperature and precipitation as well as topographic features such as elevation and slope aspect are relatively uniform along the transect. In spite of the spatially homogeneous climate, our analysis reveals a consistent signature of terrain favorability with respect to the curvature indices derived from the data for all censuses. Trees tend to grow on convex or 'bulged' surfaces along the study transect, indicating the preference of trees to be located at sites with divergent surface characteristics. Considering topographic features as proxies for hydrologic dynamics, these locations correspond to well-drained sites. Convex topography may also have negative effects on tree growth, such as extreme windswept and the likelihood of summer drying, impacting seedling establishment (Holtmeier and Broll 2005). The preference of terrain locations with convex curvature is an overall outcome of local-scale hydrologic dynamics combined with more favorable climate conditions (e.g. increasing winter precipitation), dominating over concomitant disadvantages. The subsurface conditions are also important factors affecting tree distribution. Grigorieva and Moiseev (2018) have pointed out the importance of soil moisture on the survival and growth of larch seedlings. Shiryaev et al (2019) presented a strong correlation between larch forest advance and increase of soil thaw depth and soil microbiota activities. However, such data are currently unavailable and extremely laborious to collect in the field. Future work augmenting terrain data with soil textural analysis and characterization of the subsurface thermal state is needed.
An increase in the maximum stem growth rate during the most recent period has been observed in this study. Such an increase is expected as the climate warms and the environment becomes more favorable for tree growth, as had been inferred from long-term dendrochronology studies (Briffa et al 2008, 2013), field measurements (Devi et al 2008), as well as remotely sensed Normalized Difference Vegetation Index analysis (Zeng et al 2013, Berner et al 2020). The variation in growth rates can be explained by the influence of multiple environmental factors such as soil moisture (Myers-smith et al 2015, 2020), soil nutrient availability and non-homogeneity of subsurface freeze-thaw processes (e.g. Sullivan et al 2015) for trees located at different locations. Quantitative assessment of their contributions is the topic of future research.
This study shows a clear interaction between changes in spatial patterns of tree locations and the snow distribution shifts. Many small trees and saplings of the largest cluster died between 1960 and 1999 (section 3.2) likely caused by high snow accumulation due to the wind drift—a phenomenon well pronounced at the field transect. Specifically, when snow is redistributed by wind, high roughness obstacles such as trees reduce wind speed at their lee sides leading to leeward snow accumulation (Hiemstra et al 2002). In 1960, this accumulation was observed at the bottom of the largest cluster (figure 7(a)). Although snow cover corresponds to a favorable microclimate (Hagedorn et al 2014), excessive snow accumulation results in a longer snowmelt period and a shorter growing season reducing total photosynthetic carbon gain and survival chances for small trees. As trees grew and became larger at locations with snow depths that did not limit the duration of the growing season (e.g. the upper part of the largest cluster), the leeward snow accumulation moved towards upslope (figure 7(b)). The increased tree presence in the upper areas in 2011 resulted in a new area of high snow accumulation in the middle part of the cluster. Such a change in surface roughness condition likely made the lower part of the transect even more favorable for trees to grow as indicated by a large number of new stems growing in the lower part. Such growth patterns at different locations with a phase shift signal the effect of spatial teleconnection. This effect has been displayed within and among clusters through interactions between new tree growth and snow distribution changes, which partially explain the heterogeneity of trees encroaching upslope areas along this mountain transect. Since snow distribution can change drastically between consecutive years, one obvious limitation is the incompleteness of the snow record. Improved understanding of the spatial interactions of snow with the tree encroachment process requires a more continuous record of snow distribution at fine-scale resolutions (i.e. at ~1 m).
Treeline is considered a critical indicator of climate warming (Harsch and Bader 2011). Forest extent is predicted to increase 55% in the Arctic with a 42% decrease in tundra area under projected warming in the next few decades (Kaplan and New 2006). The spatial variability of tree expansion, however, indicates the importance of other drivers of vegetation shifts besides summer warming (Elmendorf et al 2012). The results presented in this study imply the necessity to take microtopography and snow conditions into account when predicting future timberline ecotone dynamics. Specifically, two thresholds of snow depth need to be determined as a prior: the lower limit, above which trees can survive winter stresses such as wind abrasion and frost, and the upper limit, under which the growing season is long enough for trees to grow. Admittedly, however, these challenges are difficult to overcome as snowpack modeling can be a major source of error in treeline dynamics models due to the lack of precipitation data in mountain or remote regions (Paulsen and Körner 2014). The complexities displayed in the interaction between trees and snow distribution implies that until this dynamic process approaches a steady state, prediction of treeline dynamics at regional scales will remain difficult and subject to uncertainties.
Acknowledgments
This research is sponsored by the National Science Foundation Office of Polar Programs grants 1725654 (University of Michigan), 1724868 (Kansas State University), 1724633 (Georgia Tech), and 1724786 (Ohio State University), respectively. V Ivanov and V Mazepa acknowledge the support from project RUB1-7032-EK-11 funded by the U.S. Civilian Research & Development Foundation. V Mazepa acknowledges the partial support from grant RFBR-19-05-00756 from the Russian Foundation for Basic Research. Assistance of Yuriy Trubnikov with data collection and developing allometric relationship is greatly appreciated. The authors thank the two anonymous reviewers for their valuable comments that greatly improved this manuscript.
Data availability statement
The data that support the findings of this study are available upon request from the authors.