Abstract
Hydropower reservoirs are artificial water systems and comprise a small proportion of the Earth's continental territory. However, they play an important role in the aquatic biogeochemistry and may affect the environment negatively. Since the 90s, as a result of research on organic matter decay in manmade flooded areas, some reports have associated greenhouse gas emissions with dam construction. Pioneering work carried out in the early period challenged the view that hydroelectric plants generate completely clean energy. Those estimates suggested that GHG emissions into the atmosphere from some hydroelectric dams may be significant when measured per unit of energy generated and should be compared to GHG emissions from fossil fuels used for power generation. The contribution to global warming of greenhouse gases emitted by hydropower reservoirs is currently the subject of various international discussions and debates. One of the most controversial issues is the extrapolation of data from different sites. In this study, the extrapolation from a site sample where measurements were made to the complete set of 251 reservoirs in Brazil, comprising a total flooded area of 32 485 square kilometers, was derived from the theory of self-organized criticality. We employed a power law for its statistical representation. The present article reviews the data generated at that time in order to demonstrate how, with the help of mathematical tools, we can extrapolate values from one reservoir to another without compromising the reliability of the results.
Export citation and abstract BibTeX RIS

Original content from this work may be used under the terms of the Creative Commons Attribution 3.0 licence. Any further distribution of this work must maintain attribution to the author(s) and the title of the work, journal citation and DOI.
1. Introduction
Studies on greenhouse gases emissions from manmade reservoirs began in the 1990s after the publication of Kelly et al [14], followed by other research [12, 13, 18–20], and went on to analyze the spatial variations in reservoirs, the sources of organic matter and the processes involved [1, 5, 10, 15, 21, 24, 25].
The knowledge on the subject has advanced greatly since the late 90s. Two important supporting manuals for estimating emissions have become a standard reference for the international community [8, 9].
Gases in reservoirs are produced biologically by the aquatic metabolism of organic matter in flooded biomass and soils from the watershed. CH4 (methane), N2 (nitrogen) and, to a lesser extent, CO2 (carbon dioxide) are produced under anaerobic conditions, while CO2, N2O (nitrous oxide) and N2 are produced under aerobic conditions. In general the emissions are determined by a set of connected factors.
Some important factors affecting the emissions are presented below:
- the mode of organic mass decomposition (oxic or anoxic), which produces relatively more CO2 or CH4 (respectively);
- the respiration rates of biological activities in the reservoir, which introduce CO2 dissolved in the water and raise the partial pressure of this gas, increasing, consequently, its flux from the water to the atmosphere;
- the photosynthesis rate, which raises the content of oxygen dissolved in the water and may curtail the total content of CH4 therein, because the oxygen reacts (oxidizes) the methane molecules, diminishing its partial pressure and its flux to the air; conversely, the consumption of oxygen in the water favors the CH4 concentration.
- the carbon in the watershed soils (organic and inorganic) feeds the biogenic gas production (mainly DIC—dissolved inorganic carbon and DOC—dissolved organic carbon);
- CO2 and CH4 are influenced by the levels of productivity in the aquatic systems (input of nutrients to the system).
It must be stressed that there is no single factor in isolation that is responsible for the totality of the emissions. Rather, it is the conjunction of factors that produces significant effects.
The levels of greenhouse gas (GHG) emissions from dams are a strategic concern of vital importance, and comparisons with other power generation options such as thermal power, for instance, are helpful in clarifying the issues and implications.
The findings of the studies made to date are highly sensitive to the values of the input variables and the methodologies chosen, requiring careful analysis and interpretation. The data extrapolation method appears to be crucial in this regard.
It has been conjectured that the concept of self-organized criticality and power laws can be applied to the statistical study of GHGs in hydroelectric reservoirs [23]. The power law that is typical of the phenomenon called 'self-organized criticality (SOC)'—a term used in physics to describe (classes of) dynamic systems which have a critical point as an attractor—implies generally a fractal profile for the intensity of the phenomenon over time. The power law gives the number of events N of intensity I (expressed, for our purposes, in mass of carbon or gas emitted per unit of area and time), according to equation (1):

A and λ are fixed parameters, to be estimated.
This statistical model was chosen for the following reasons:
- In terms of the mass of carbon per area in a given time period (t C m−2 y−1), low intensity emissions predominate in most cases, but some higher intensity ones do occur, including a few very high intensity emissions.
- Great variations in the intensity of emissions in the same time period were observed from one area to another in each reservoir and also within each reservoir area over time. This is compatible with a fractal profile.
- There is a complex conjunction of various factors that influence the emissions in a reservoir.
- It would be difficult and costly to carry out onsite experimental measurements, collecting data and obtaining reliable statistics for each one in the whole set of 251 dams operating in Brazil, especially considering the variability of emissions over time.
SOC's macroscopic behavior thus displays the spatial and/or temporal scale-invariance characteristic of the critical point of a phase transition, but, unlike the latter, in SOC these features obtain without the need for control parameters to reach a given value. The phenomenon was first identified in 1987 as one of the mechanisms through which complexity arises in nature. The concept has been eagerly applied in fields as diverse as geophysics, cosmology, evolutionary biology and ecology, economics, sociology, solar physics and others [16].
Considering all these factors and their great uncertainty, the ultimate goal would be to establish a clear and quantifiable cause-effect connection between the multiple intervening variables and the observed emissions, something which, however, is beyond the scope of this paper.
There are a number of gaps in the literature on the subject that are worth mentioning such as the issue of extrapolating data for a set of hydroelectric reservoirs as per the works of Saint Louis et al [22] and Barros et al [2].
The objective of this paper is to employ GHGs measurement data from tropical reservoirs together with a mathematical model to extrapolate the reservoir averages to a larger set of reservoirs.
The data extrapolation from a set of sampling points to the entire reservoir uses an average value of the diffusion fluxes obtained and a weighted average of the depth points for the ebullitive fluxes.
The originality of the work is the use of self-organized criticality theory and of the power law of physics to create a mathematical model for data extrapolation applicable to a large group of hydropower reservoirs.
2. Sites and methods
Emissions of CO2 and CH4 in each of the reservoirs selected, whether through bubbles or diffusive exchanges between water and the atmosphere, were assessed by sampling, with subsequent extrapolation of the results in order to obtain a total value for the whole reservoir. Experimental measurements of gas emissions from reservoirs were made to determine precisely the emissions of CH4 and CO2, both in the form of bubbles formed in the bottom of the lake from the decomposition of organic matter and as gases that are transported vertically in the gradient of the lake by molecular diffusion. To arrive at an average value for the total of each reservoir, based on the results of experimental observations made only at certain points of the reservoir and in the span of a few days during the year, a criterion for extrapolation had to be adopted.
In fact, only two series of measurements, separated by an interval of 6 months, were feasible, and each sampling campaign took an average of 5 to 7 days. Gas fluxes were measured during the day and the night so as to be able to account for the variation in insolation conditions. Each reservoir had 10 to 15 sampling points of diffusive fluxes and 8 to 12 sampling points of fluxes from ebullition. Table 1 shows a summary of the data collected.
Table 1. Summary of data collection.
Reservoir | Sampling period | Number of sampling trips | Number of ebullitive sampling stations | Number of diffusive sampling stations |
---|---|---|---|---|
Miranda | April 1998 and December 1998 | 2 | 9 | 9 |
Três Marias | April 1998 and March 1999 | 2 | 12 | 13 |
Barra Bonita | April 1998 and November 1998 | 2 | 6 | 12 |
Segredo | May 1998 and November 1998 | 2 | 12 | 18 |
Xingó | May 1998 and March 1999 | 2 | 7 | 11 |
Samuel | June 1998 | 2 | 7 | 16 |
Tucuruí | June 1998 and June 1999 | 2 | 10 | 10 |
Serra da Mesa | March 1997 and November 1997 | 2 | 19 | 19 |
Itaipú | March 1998 and March 1999 | 2 | 12 | 15 |
Because of the field conditions, the measurements were limited, not only in spatial terms but also in time. This relative scarcity increased the uncertainty, but, for practical reasons, it was not possible to intensify, prolong or repeat the sampling campaigns.
For the emissions through bubbles, which do not occur at greater depths, a weighted average was computed for the reservoir as a whole. For the diffusive emissions, which are independent of the depth, a simple average of the measured values was used.
The method was aimed to assess the emissions of gases in nine reservoirs of different ages and located at various latitudes. To capture possible variations in the analytical parameters, appropriate criteria were chosen to account for the different environmental conditions of the reservoirs.
The hydroelectric reservoirs were selected so as to be representative of their different types in Brazil, in terms of the range of latitudes; the time since the initial filling of the large and the small reservoirs; reservoirs in areas where human activity had been previously present or where the natural conditions were preserved; and reservoirs with a wide range of the average water residence time.
Methane and carbon dioxide emissions were measured in nine hydroelectric reservoirs, namely: Itaipú, Serra da Mesa, Miranda, Três Marias, Barra Bonita, Segredo, Xingó, Samuel and Tucuruí. These reservoirs range from latitudes of 2° S to 25° S and the vegetation types include the major Brazilian ecosystems such as: the equatorial rainforests, the sub temperate forests, the Atlantic forest, the cerrado (savannah) and the caatinga (semi-arid). The time since the flooding of the reservoirs ranges from one to twenty years, providing the study with a good temporal representation. Fourteen sampling surveys were carried out between 1998 and 1999.
Table 2 provides a brief description and figure 1 provides the geographical location of the reservoirs studied.
Table 2. Technical description of the reservoirs studied.
Reservoir | Latitude | Biome | Years after flooding | Power (MW) | Area of reservoir (maximum operational level) (km2) | Energy density (W m−2) |
---|---|---|---|---|---|---|
Miranda | 18°55´S | Cerrado | 1 | 390 | 50.6 | 7.71 |
Três Marias | 18°13´S | Cerrado | 37 | 396 | 1040 | 0.38 |
Barra Bonita | 22°31´S | Atlantic Forest | 36 | 140.76 | 312 | 0.45 |
Segredo | 25°47´S | Atlantic Forest | 6 | 1260 | 82 | 15.37 |
Xingó | 9°37´S | Caatinga | 4 | 3000 | 60 | 50 |
Samuel | 8°45´S | Amazon Forest | 9.6 | 216 | 559 | 0.39 |
Tucuruí | 3°45´S | Amazon Forest | 14 | 8000 | 3000 | 2.66 |
Serra da Mesa | 14° S | Cerrado | 18 | 1275 | 1784 | 0.71 |
Itaipú | 24° 43' S | Atlantic Forest | 4 | 12 600 | 1.546 | 8.15 |
Figure 1. Geographical localization of the reservoirs studied.
Download figure:
Standard image High-resolution image2.1. Emission rates of gases in the form of bubbles
The emissions of GHG in the form of bubbles have a random behavior. Samples of the bubbles were captured, after their spontaneous liberation from the bottom of the reservoir, using a set of 16 bubble collector funnels (cones of a synthetic sheet on an aluminum framework, 75 cm in diameter and coupled to gas collecting bottles). The funnels were deployed under the surface of the water, at a maximum depth of 20 meters, coupled to plastic bottles that served as buoys and were anchored by stones of around 10 kg each, attached with a rope.
The choice of the sampling sites and the arrangement of the funnels were determined by parameters such as the year the reservoir was filled, the depth, the presence of semi-submerged vegetation, and the geographic region of the reservoir. The funnels were positioned in different areas of the reservoirs so as to cover both the sheltered areas (sheltered bays and arms) and the open areas of the reservoir body. They were placed at different depths in the following ranges: 0–5 meters, 5–10 meters, 10–15 meters and 15–20 meters. The funnels remained at the site for 24 h, the period during which bubbles released from the bottom were captured. Then the collecting bottles were hermetically sealed underwater and kept for later laboratory analysis.
2.2. Rates of gases emitted by diffusion using 'diffusion chambers'
Diffusion chambers are mechanisms that resemble inverted small cups, with a total volume of 75 ml and able to contain a submerged 'bubble' of atmospheric air at a shallow depth (of around 20 cm below the surface) with a contact surface of 22 cm2, through which there is an exchange of gases, here called diffusion.
The initial gas concentration was assessed at zero minute and measured again after 3, 6, and 12 min of contact. In each occasion, a 3 ml aliquot was withdrawn from the chamber, which reduces slightly the total volume of the headspace. These 'bubble' samples were taken to the laboratory and the change in the concentration of each gas was estimated chromatographically, to be able to calculate the emission or absorption rate, as the case may be. 800 chromatographic analyzes of the gas samples from the reservoir were made in the chromatography laboratory installed in one of the buildings close to the reservoir.
2.3. Emission rates
To obtain emission rates by the bubbles, the average values were used for different depth ranges and approximate mathematical functions were established that describe the emission rates as a function of depth. One of the functions used was a straight line and the others were exponential functions.
Normally the total area of the reservoirs is known, but not necessarily the 'area which emits bubbles' because hydrostatic pressure maintains in place some bubbles at the bottom of the reservoir at depths greater than 20 meters. Therefore, the emission rates referring to the total area were determined, along with the rates for the 'area which emits bubbles'.
For each reservoir, the area which emits was estimated based on first degree experimental equations, which describe the bubble emissions y of the gas as a function of the depth x of the sampled site. For each reservoir, the equation y(x) was obtained, and on this basis the value of xo was calculated, such that:

In this model, each reservoir emits in the belt between the shore and the geometric site of depth xo. The 'bubble emitting area' is thus defined.
Multiplying the pairs corresponding to each area and the respective associated rate, the total mass of the gas emitted from each depth range of the reservoir was obtained. The sum of these values represents the total mass of the gas emitted by the whole reservoir. From the total masses, the reservoir's average emission rate for each gas was calculated.
Calculating the emissions from each of the selected reservoirs involved sampling each one at various locations so as to later extrapolate from these measurements a figure for the entire reservoir. The measurements and their extrapolations were made according to a well-established routine.
The field sampling trips lasted five to six days. Each day the sampling team would go to the reservoir to start the experimental measurements for diffusive and ebullitive sampling fluxes. The team would spend the entire day at the reservoir and come back to the laboratory at night. The gasometers with gas samples were brought to the laboratory for analysis. After the analysis, the concentration data were converted into mass figures for each gas. For details on the field and extrapolation procedures see [7].
By contrast, the second type of extrapolation for the set of all reservoirs requires special attention not to introduce extra uncertainty, since there are no well-established routines readily available. The emission rate was calculated based on the data obtained from the experimental measurements, yielding an average value for the two surveys of each reservoir.
This was then used to extrapolate a value for a one-year period.
2.4. The main sources of uncertainty
The results obtained come from two data collection surveys, and the extrapolation of the values that was adopted implies a simplified hypothesis for the emissions from hydroelectric reservoirs, since the emissions were projected as constant over the period of time covered.
This simplified hypothesis can introduce some uncertainty in the data extrapolation estimations.
For example, a recent Curtarelli et al [4] work showed that at diel and daily time scales the CO2 emissions show a significant spatial and temporal variability which can introduce high uncertainty in estimates based on a constant value over time. Other sources of uncertainty are fluctuations in the water level of the studied reservoirs that can change the total area of the reservoir. Curtarelli et al [4] indicated that including a variable reservoir surface area can lead, in the course of a year, to obtaining estimates around 18% lower than those resulting from a fixed water level.
3. Data extrapolation for the whole hydropower system
When primary data is not directly available, the mathematical and statistical methodology deployed for extrapolation, as in [18], relies on the grouping of data according to arbitrarily chosen ranges. Such an approach requires the postulation of several hypotheses about the distribution of the emissions in order to derive the aggregate results. The choice of range boundaries and averages inevitably affects the resulting total values. There is no established consensus on what constitutes the best criterion for such a selection.
By contrast, our study is based on original field survey data, using a reliable methodology for extrapolation. In the early stages of our study, the methodology allowed us to dispense with the grouping of data in ranges. Thus we avoided the arbitrariness in defining range limits and the consequent uncertainties in the total values inherent in the alternative approach [7].
Since its initial study, COPPE (Coordination of Post Graduate Courses in Engineering) has used a power law as one of two possible alternatives for extrapolating the total emissions from all hydroelectric plants, based on measurements taken from a sample set of reservoirs specified by the study. The power law is useful in accounting for the presence of extreme events—in this case, corresponding to extremely high CH4 and CO2 emissions—even though they are rare. Such events, if they did not occur during the short emissions sampling period, could be under-represented and thus affect the computed averages. Hence an appropriate probability density function (a Pareto distribution) that included such extreme events and the power law were employed to address such methodological issues [16].
To extrapolate the measured data to a set of reservoirs, the study [7] calculates the average of all the reservoirs using the power law N(I) = AI−λ. An integral is calculated between limits given by the maximum and minimum values, as per equation (3) below:
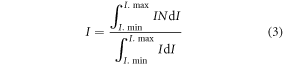
Substituting the terms N and I in equation (3), we have the following result (equation (4)) in terms of the power equation:

It is easy to verify that equation (4) [7], corresponds exactly to the correction proposed by [11]. This formula allows the calculation of the average value of emissions, based only on the parameter λ and on the values Imax and Imin, which correspond, respectively, to the highest and the lowest levels of the emissions measured.
With the sample data from the field measurements, it is possible to calculate the value of λ that best fits the data. This is done based only on the original field observations, without using arbitrary ranges, and seeking the parameter λ that generates the curve N(I) = AI−λ which would be closer to the field observations than any other similar curve.
4. Results obtained
Four different alternatives were tested in order to estimate the parameters associated with the power law, but meaningful results were obtained in only two of them, which will be further detailed here. The first one is the orthodox method of least squares, which basically seeks to minimize the sum of the squares of the deviations between the values observed and those given by the theoretical distribution. In this alternative, a boundary condition was imposed such that the integral of the density function between the minimum intensity value and infinity would be equal to 1 (one), as has to be the case with probability density functions. Thus, the following restriction was included:

Where:
Imin was taken as 99% of the minimum intensity actually observed. The implicit assumption is that there are no occurrences below the chosen minimum intensity.
In the other alternative, the parameters were estimated observing the criterion of least total absolute deviation, aimed at minimizing the sum of the absolute deviations between the observed values and those derived from the theoretical distribution. Thus, the following (non-linear and non-differentiable) problem was solved:

Where:
- n—is the total number of observations;
- (Ii, Yi)—represents the pair of each generic observation i;
the value given by the power law as a function of the independent variable Ii and the parameters λ and A.
In this alternative, as in the first, the restriction in equation (5) was made.
It should be emphasized that this last alternative is, in principle, better, since the field measurements may entail uncertainties that affect the least squares estimators, which are extremely sensitive to outliers, by contrast to the least total deviation estimator. It is, however, more difficult to estimate the parameters in this second alternative since it requires numerical approximation methods. Table 3 summarizes the results obtained by computer algorithms:
Table 3. Computational approximations obtained for different parameters.
CH4 bubbles (mg m−2 d−1) | CH4 diffusion (mg m−2 d−1) | CO2 bubbles (mg m−2 d−1) | CO2 diffusion (mg m−2 d−1) | |||||
---|---|---|---|---|---|---|---|---|
Data | Imax | Imin | Imax | Imin | Imax | Imin | Imax | Imin |
1205 | 0.0001 | 4572 | 0.002 | 23.3 | 0.002 | 142 723 | 0.0001 | |
Alternative | λ | A | λ | A | λ | A | λ | A |
1 | 1.0800 | 0.038 25 | 1.0820 | 0.049 21 | 1.2170 | 0.056 18 | 1.0410 | 0.028 13 |
2 | 1.0780 | 0.037 97 | 1.0840 | 0.049 66 | 1.2110 | 0.056 70 | 1.0410 | 0.028 09 |
Observing equation (5), which involves the integral of the density function and can be solved analytically, as the upper limit gets to be large enough, approaching infinity, the integral would only converge to a finite value if −λ + 1 < 0, or λ > 1. In other words, the value of λ has to be greater than 1, otherwise the equation would not hold. Both alternatives yielded values of λ above 1, as expected.
The two alternatives produced results that are practically equivalent and mutually confirming. This indicates that outliers were not significant where the field measurements are concerned. The results presented below were obtained with the parameters for the first alternative.
The average emissions were calculated with equation (4). For bubble fluxes the average CH4 is 39.0 mg CH4 m−2 d−1 and the correlation attained is 93%. For CO2 it is 1.0 mg CO2 m−2 d−1 with a 98% correlation. For diffusion fluxes the average CH4 is 175.9 mg CH4 m−2 d−1 with an 83% correlation. For CO2 it is 4443.5 mg CO2 m−2d−1 with a 79% correlation.
Using the self-organized criticality theory, the average emissions rate per area for the Brazilian hydroelectric reservoirs would then be the sum of the average fluxes, in the forms of bubbles and of diffusion for each type of gas:


Based on these two figures, we can extrapolate the total flux for each of the two gases emitted by each of the 251 Brazilian hydroelectric plants, by multiplying these values by the surface area of each hydroelectric reservoir, as obtained from the National Electric Energy Agency—ANEEL, the Federal government agency in charge of regulating the electric power supply.
The same criterion was adopted to compute the total emissions of each gas produced by the whole hydroelectric system, by multiplying the total area of the reservoirs by the respective average emissions per area. The total value for the whole hydroelectric complex of the 251 plants is thus 6980 tons of CH4 and 144 379 tons of CO2 per day. The calculations are summarized in table 4.
Table 4. Extrapolation of GHG emissions for the hydroelectric complex using the power law criterion.
CH4 emission rate | CO2 emission rate | Total area of reservoirs | Daily emissions of CH4 | Daily emissions of CO2 |
---|---|---|---|---|
mg m−2 d−1 | mg m−2 d−1 | km2 | t d−1 | t d−1 |
214.9 | 4444.5 | 32 485 | 6980 | 144 379 |
The correction made in COPPE's 2002 study [7] shows higher average emission values relative to the estimates in our pioneering work [6, 23]. Notwithstanding this increase, the corrected figures clearly confirmed that, in most cases, the emissions from hydroelectric plants remain considerably lower than those from thermoelectric plants, for the same amount of power generated. Table 5 is illustrative in this context.
Table 5. Comparison between emissions from hydroelectric plants and equivalent thermoelectric plants.
Average of the two campaigns | Emissions from equivalent thermoelectric plantsb | Merit index missions from thermoelectric plants/emissions from hydroelectric plants | ||||||||||||||
---|---|---|---|---|---|---|---|---|---|---|---|---|---|---|---|---|
Reservoir area | Power | CH4 emission rRate | CO2 emission rate | Hydro plant's emissionsa | Coalc simple cycle | Oild simple cycle | Diesel oile simple cycle | Gasf simple cycle | Gasg combined cycle | |||||||
Hydroelectric plant | Latitude | km2 | MW | mg m−2 d−1 | mg m−2 d−1 | t C year−1 | t C-CO2 year−1 | t C-CO2 year−1 | t C-CO2 year−1 | t C-CO2 year−1 | t C-CO2 year−1 | Coal simple cycle | Oil simple cycle | Diesel Oil simple cycle | Gas simple cycle | Gas combined cycle |
Tucuruí | 3°45'S | 2430 | 4240 | 109.40 | 8475 | 2791 038.13 | 4661 873 | 4702 228 | 4501 659 | 4330 284 | 2598 170 | 1.67 | 1.68 | 1.61 | 1.55 | 0.93 |
Samuel | 8°45'S | 559 | 216 | 104.00 | 7448 | 576 491.62 | 237 492 | 239 547 | 229 330 | 220 599 | 132 360 | 0.41 | 0.42 | 0.40 | 0.38 | 0.23 |
Xingó | 9°37'S | 60 | 3000 | 40.10 | 6138 | 43 366.78 | 3298 495 | 3327 048 | 3185 136 | 3063 880 | 1838 328 | 76.06 | 76.72 | 73.45 | 70.65 | 42.39 |
Serra da Mesa | 13°50'S | 1784 | 1275 | 51.10 | 3973 | 959 655.93 | 1401 860 | 1413 995 | 1353 683 | 1302 149 | 781 289 | 1.46 | 1.47 | 1.41 | 1.36 | 0.81 |
Três Marias | 18°13'S | 1040 | 396 | 196.30 | 1117 | 684 667.27 | 435 401 | 439 170 | 420 438 | 404 432 | 242 659 | 0.64 | 0.64 | 0.61 | 0.59 | 0.35 |
Miranda | 18°55'S | 51 | 390 | 154.20 | 4388 | 44 196.75 | 428 804 | 432 516 | 414 068 | 398 304 | 238 983 | 9.70 | 9.79 | 9.37 | 9.01 | 5.41 |
Barra Bonita | 22°31'S | 312 | 140.76 | 20.90 | 3985 | 141 942.10 | 154 765 | 156 105 | 149 447 | 143 757 | 86 254 | 1.09 | 1.10 | 1.05 | 1.01 | 0.61 |
Itaipú | 25°26'S | 1549 | 12 600 | 2080 | 171 | 116 171.20 | 13 853 680 | 13 973 602 | 13 377 571 | 12 868 296 | 7720 978 | 119.25 | 120.28 | 115.15 | 110.77 | 66.46 |
Segredo | 25°47'S | 82 | 1260 | 8.80 | 2695 | 24 009.85 | 1385 368 | 1397 360 | 1337 757 | 1286 830 | 772 098 | 57.70 | 58.20 | 55.72 | 53.60 | 32.16 |
Sample total | 7.867 | 23 518 | 85.04 | 4491 | 5381 539.62 | 25 857 739 | 26 081 572 | 24 969 088 | 24 018 532 | 14 411 119 | 4.80 | 4.85 | 4.64 | 4.46 | 2.68 |
aIncludes carbon from CH4 (with GWP of 10.1818 expressed in mol according to Myhre et al 2013) and from CO2: (CH4 × 12/16 × 10.1818 + CO2 × 12/44) × 365/1000; bHydroelectric plant's load × 0.5 × 365 * 24 × CO2 emission factor/fuel efficiency. The value of 0.5 corresponds to the average capacity factor of the system (Eletrobras/MCT, 2000). cCO2 emission factor for coal, simple cycle: 0.092 88 tC MWh−1, with 37% efficiency; dCO2 emission factor for fuel oil, simple cycle: 0.075 96 tC MWh−1, with 30% efficiency; eCO2 emission factor for diesel oil, simple cycle: 0.072 72 tC MWh−1, with 30% efficiency; fCO2 emission factor for natural gas, simple cycle: 0.055 08 × 1.27 tC MWh−1, with 30% efficiency; gCO2 emission factor for natural gas, combined cycle: 0.055 08 × 1.27 tC MWh−1, with 50% efficiency; In the case of natural gas, the above-mentioned emission factors include the factor 1.27 related to fugitive losses.
Table 5 shows that among the nine hydroelectric plants (HEPs) analyzed, only in two cases (Samuel, by a larger difference and Três Marias, by a smaller one) would most types of thermoelectric plants (TEPs) be a better option than the HEPs with respect to GHG emissions. Of the seven other cases, only three are outperformed by the most efficient TEPs, namely, those based on a combined natural gas cycle. In the four remaining cases, the HEPs outperform all types of TEPs by a large margin, emitting up to 66 times less GHG than their TEP counterpart. The total GHG emissions from the most efficient TEPs (gas combined cycle), producing the same amount of energy as the HEPs in the sample, would be 2.68 times more than those from the sample HEPs, while less efficient TEPs would emit between 4.46 to 4.85 times more than the HEPs.
When the results were generated, the issue of degassing by the structures through which the water flows at the dam (turbines and spillways) had not yet been investigated. Later on, such measurements were initiated, showing that, in some cases, the degassing is negligible but in others it can be quite significant [3]. With respect to CO2, the share of degassing in the total emissions varies from 0.7% to 47.49%, while in the case of CH4 it varies from 0.02% to 48.77%. The following table 6 provides the proportions obtained for each project.
Table 6. Percent participation of degassing emissions in total emissions of studied hydropower reservoirs.
Hydropower plant | CO2 emissions (%) | CH4 emissions (%) |
---|---|---|
Tucuruí | 0.7 | 0.2 |
Xingó | 47.49 | 1.16 |
Serra da Mesa | 1.5 | 20 |
Três Marias | 2.7 | 0.02 |
Itaipú | 1.8 | 0.19 |
Segredo | 33.1 | 4 |
Funil | 13.5 | 1.6 |
Balbina | 1.3 | 48.77 |
Source: Brasil 2014.
5. Comparison with results of other studies
Even when using the re-estimated values for HEP methane emissions, which are much higher than the calculations of other studies [11, 17], our results support the argument in favor of hydroelectric plants. In the case of Brazil, with respect to GHG emissions, they can be shown to be preferable to thermoelectric plants4 .
Our work contends that the most relevant comparison of emissions to be made is between different sources for power generation, such as those from fossil-fuel fed thermoelectric plants and hydroelectricity. An arbitrary comparison, such as the one offered by [11] between the total emissions from some large city (São Paulo, in this case) and those from hydroelectric plants, does little to clarify the actual choices for energy production that governments face. The city's emissions are obviously unrelated to the problem of emissions from the electric power sector, since they arise from a variety of sources and are all related to the consumption of energy rather than to its production in a usable form.
The article quoted above also suggests that our own work has been consistently biased in favor of hydroelectric plants and thus we have tended to underestimate their GHG emissions. This, however, is incorrect, since our second work of 2002 [7], employing a more refined methodology, arrived at total emissions results which were significantly higher than those obtained by Pueyo & Fearnside themselves in 2011, i.e., nine years later. Our values in 2002 are above 200 mg CH4 m−2 d−1 [7], while [17] estimate 81.3 mg CH4 · m−2d−15 .
Ignoring our findings and, contrary to an even cursory review of our work, Pueyo and Fearnside [11] have insisted that we have stuck to our initial underestimated figures of 20006 . Thus, they argue that Brazil has adopted an incorrect position with respect to energy planning, showing a preference for hydroelectricity generation, an option that they claim is based on flawed scientific grounding. However, our work from 2002 [7] shows clearly that this is not the case and it is important to stress that the higher values did not challenge the final conclusion that hydroelectricity tends, for the most part, to produce weaker GHG emissions than thermoelectricity.
6. Final comments
The Fearnside and Pueyo's [11] comparison between hydroelectric plants' emissions and those of the city of São Paulo on the basis of which the core of the criticism for Brazil's preference for electricity generation is made is, patently, misleading. There is no such strategic choice to be made—on the one hand, there are the figures for the city's emissions from disparate sources and, on the other, there are the calculations for GHG hydroelectricity emissions which must bear on the assessment of feasible alternatives for power generation. The meaningful decision can and must be between real available options such as hydroelectric plants, thermoelectric plants (based on fossil fuels, nuclear power or biomass), and/or other energy sources such as renewables—wind, solar, ocean power, etc.
The technology for power generation from renewables other than hydroelectricity is of relatively recent origin. At the current technological level, these sources cannot, as yet, produce energy on the scale and with the steadiness necessary for the ongoing development of the country or at costs that are competitive with existing alternatives. Despite its very low GHG emissions, nuclear energy, for instance, is an option that presents its own particular environmental problems and high energy costs, as is well established in the scientific literature, though beyond the scope of our present discussion. The main choice for expanding the supply of electric power, at least within the horizon of the next few years, is between fossil-fuel run thermoelectric plants and hydroelectric plants.
It is true that there is a certain degree of complementarity between these two sources for electricity generation, given the seasonal—and, therefore, not totally predictable—nature of hydroelectricity production, as compared to the predictability and rapid start-up time of thermoelectric plants. Hence, in the Brazilian grid system's operation model, largely based on hydroelectricity, thermoelectric plants are justified to be activated when hydroelectric power runs low. However, the ideal situation, from the perspective of lower GHG emissions, is that thermoelectric plants be kept idle for most of the time, because they are the higher GHG emitters. To what extent such complementarity is feasible involves considerations beyond the scope of our article.
To conclude, present day Brazil's energy planners cannot ignore the overall superiority7 of hydroelectric plants over thermoelectric ones in terms of GHG emissions and their impact on climate change.
Footnotes
- 4
Indeed, the results published by Fearnside and Pueyo [11] show emissions that are greater than the original ones found in Santos (2000) [23] and reflected in the Eletrobras (2000) publication [6]. It should be emphasized that Santos' PhD thesis, written in 2000, was a pioneering study on the subject, and Eletrobras made it available on its webpage that same year because it was the only document, at the time, measuring the effective emissions from Brazilian reservoirs (see [23]). But, since 2002, our work shows much higher re-estimated emissions' values.
- 5
Fearnside and Pueyo (2012) [11] uses the unit mg m−2 d−1, which is equivalent to kg km−2 day−1.
- 6
Fearnside and Pueyo (2012) [11] quotes erroneously an article that we wrote in 2008 in support of the argument that we continue to underestimate the emissions, although that article does not even mention those estimates, in any passage.
- 7
There are a few exceptions which have already been pointed out in our work and in the PhD thesis itself (Santos 2000) [23].