Abstract
The paper presents the results of the classification of handwritten digits from the MNIST database using the Hopfield network. A strong correlation of training binary patterns does not allow the use of the standard Hebbian learning method. The application of the Storkey learning method increases the capacity of associative memory, and the optimized pattern binarization threshold and pattern size reduce the correlation of patterns. By optimizing these parameters, a network achieved a classification accuracy of 56.2% on a set of validation data used for network training. The selection of the optimal binarization threshold for a separate set of test images increased the classification accuracy to 61.5%.
Export citation and abstract BibTeX RIS
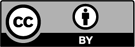
Content from this work may be used under the terms of the Creative Commons Attribution 3.0 licence. Any further distribution of this work must maintain attribution to the author(s) and the title of the work, journal citation and DOI.