Abstract
Feed Forward Neural Network (FFNN) model is the best model to forecast the time series data. In this research, The Fuzzy Feed Forward Neural Network (FFFNN) with backward propagation method is used to predict the Jakarta Islamic Index (JII) data time series in 2018. Fuzzy is used as input to the FFNN model because it is overcome the weaknesses of the inaccurate results of the FFNN when the data is unclear or incomplete. The purposes of this research are to explain the procedure to generate the FFFNN model. The steps of the FFFNN model prepare the input data to become a fuzzy number using Growth-S Curve fuzzification; the second is divide the data into two training and testing data, the third is determining the best neural network architecture with different neurons and hidden layers to get the best weights that used for the forecasting model. In this research, the best FFFNN model is built by 19 neurons and one hidden layer with 90% and 10% training and testing data, respectively. Therefore with the model obtained, forecasting produces the value of MSE 0.0018 in training and 0.0004 in testing. From the MSE values obtained, it can be concluded that the forecasting using FFFNN model is reasonable to predict.
Export citation and abstract BibTeX RIS
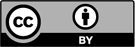
Content from this work may be used under the terms of the Creative Commons Attribution 3.0 licence. Any further distribution of this work must maintain attribution to the author(s) and the title of the work, journal citation and DOI.