Abstract
The application of password usage in databased user authentication method is unsafe, due to higher chance of off-line guessing attacks. Hackers are able to save our personal information and can detect them until the perfect match is acquired. Thus, the aim of this research is to examine EEG signals during typing task from three engineering students (right handed) with age of between 19 to 23 years old to identify the regular human typing pattern to differentiate between one user to another. All the subjects were asked to perform three times typing tasks for 5 minutes with rest in between for 30 seconds. The subjects were required to rest before and after performing the typing tasks for one minute. Truscan EEG device (Deymed Diagnostic, Alien Technic, Czech Republic) with frequency sampling of 1024 Hz and 19 channels was used. This study applied Infinite Impulse Response (IIR) notch filter to remove 50Hz powerline artefacts and proposed the implementation of nonlinear features such as Distribution Entropy (DistEn) and Approximate Entropy (ApEn). The features were extracted from channels F3 and F4. The extracted entropies features vector are used as an input to k-Nearest Neighbour (kNN) classifier. As a conclusion, the kNN and LDA classifier giving promising accuracy which are 82.22% (F3), 88.52% (F4), 94.81% (F3 and F4) and 80.37% (F3), 81.48% (F4), 89.63% (F3 and F4) respectively.
Export citation and abstract BibTeX RIS
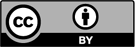
Content from this work may be used under the terms of the Creative Commons Attribution 3.0 licence. Any further distribution of this work must maintain attribution to the author(s) and the title of the work, journal citation and DOI.