Abstract
Predicting stock market movements is a well-known problem of the machine learning field. In general, there are two primary methods used to analyze stocks and make investment decisions: fundamental analysis and technical analysis. But fewer researchers focus on monthly revenue indicators and different time period prediction. We collect and organize financial data extracted from Taiwan and U.S n companies' monthly and quarterly financial reports across a period of 10 years. In addition, we successfully use fundamental and technical indicators as training model's features. Among experiment results, which has good performance. The annualized profitability (annualized rate of return) can reach 2.56%, the Sharpe ratio is 2.01, the maximum amplitude is - 20.8%. Compared with other strategies, our strategy is relatively stable and achieves ideal results. The more important is we used monthly revenue indicators based features to improve model performance.
Export citation and abstract BibTeX RIS
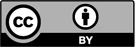
Content from this work may be used under the terms of the Creative Commons Attribution 3.0 licence. Any further distribution of this work must maintain attribution to the author(s) and the title of the work, journal citation and DOI.