Abstract
Spring phenology is a sensitive indicator of climate change and has substantial impacts on the carbon cycle. The global N cycle has been greatly disturbed by anthropogenic activities resulting in altered atmospheric N deposition worldwide. Research has been focused on the changes in the spring phenology and its covariations with climatic factors. However, the influences of N deposition on spring phenology have not been well documented to date. Herein, we investigated the effects of N deposition on the start of growing season (SOS) in continental United States (CONUS) during the years 1986–2015 using the normalized difference vegetation index (NDVI) datasets derived from both the third generation NDVI dataset and the Moderate Resolution Imaging Spectroradiometer (MODIS). We observed that N deposition could only explain approximately 5% of temporal variation in SOS in CONUS. However, the sensitivities of SOS in response to unit change in both temperature (ST) and precipitation (SP) showed clear decreasing spatial patterns with increasing N deposition. The ST generally decreased from −6 d/°C in low N deposition regions (<2 kg ha−1) to −4 d/°C in areas with N deposition >4 kg ha−1. Furthermore, the positive SP also showed a continuously decreasing pattern with the increase in N deposition, but the negative SP was gradually weakened when N deposition was >1.0 kg ha−1. The results have important implications as it reveals the role of N deposition on spring plant phenology, and strongly suggest the consideration of N deposition effects when analyzing or predicting spring phenology in response to future climate change.
Export citation and abstract BibTeX RIS

Original content from this work may be used under the terms of the Creative Commons Attribution 4.0 license. Any further distribution of this work must maintain attribution to the author(s) and the title of the work, journal citation and DOI.
1. Introduction
Vegetation phenology, especially spring green-up date, is one of the key sources of information for mapping, managing, and monitoring the terrestrial ecosystem from local to global scales (Cleland et al 2007). Shifts in spring phenology not only reflect the biosphere's dynamic response to climate change (Richardson et al 2018), but also affect the energy exchange, hydrological cycle, and carbon uptake of the ecosystem (Piao et al 2007, Bonan 2008, Sitch et al 2008, Peñuelas et al 2009, Richardson et al 2013). Thus, accurate understanding of the changes in spring phenology and its influencing factors is critical for the prediction of ecosystem responses to climate change.
Previous studies have widely examined the effects of climatic factors, especially temperature and precipitation, on spring phenology using both ground and satellite data. Temperature has been recognized as the major factor in triggering spring phenology, which was previously occurring worldwide due to global warming (Menzel et al 2006, Piao et al 2007, 2015, Ge et al 2015). Additionally, precipitation affects spring phenology through altering heat requirements and temperature sensitivity for vegetation green-up (Fu et al 2014, Shen et al 2015). However, ground observations indicate that other environmental factors such as N input via deposition additionally trigger the variations in spring phenology (Schulte-Uebbing and de Vries 2018). During the past few decades, the N deposition has considerably increased at global scale due to anthropogenic activities (Gruber and Galloway 2008, Liu et al 2013, Kanakidou et al 2016, Yu et al 2019). Increased N deposition alters nutrient accumulation and allocation patterns in the soil, and consequently affects vegetation growth (Vitousek and Howarth 1991). Previous studies have shown that N deposition has the potential to alter flowering phenology but the degree could vary considerably with species. For example, based on field experiments, Cleland et al (2006) found that N addition delayed the flowering date in grasses but accelerated the flowering date in forbs. Another study from Liu et al (2017) similarly observed that among different species in a Tibetan alpine meadow, flowering times were advanced, delayed, or showed no response to N additions. Furthermore, flowering phenology occurred later in alpine tundra with exclusive addition of N, but was unchanged when both snowpack and N were increased (Smith et al 2012). Addition of N could dampen the acceleration of greening caused by increasing temperature (Cleland et al 2006), and even offset the effects of climate warming on spring phenology in certain biomes (Wang and Tang 2019), ultimately weakening the temperature sensitivity of plant phenology. These studies suggest that increased N deposition could impact spring phenology and its sensitivity to climate change. However, these previous studies on the relationships between phenology and N deposition are often based on short-term (only two or three years) manipulated experiments, and consequently it remains unclear whether and how atmospheric N deposition will affect large-scale spring phenology over a long time.
Remote sensing techniques have been extensively used to continuously observe phenological changes in the vegetated land surface at regional to global scales during the past three decades (Shen et al 2014, Piao et al 2015, Gonsamo and Chen 2016, Wu et al 2016). To distinguish these metrics from ground observation data, remote sensing-retrieved phenology is usually referred to as land surface phenology (LSP) (de Beurs and Henebry 2004, Zhang et al 2017). To date, several algorithms have been developed to derive LSP at regional and global scales from various satellite data, including the Advanced Very High Resolution Radiometer (AVHRR), SPOT-VEGETATION, and Moderate Resolution Imaging Spectroradiometer (MODIS). These algorithms are generally based on time series vegetation indices (VI). The VI time series are first smoothed through Fourier analysis (Roerink et al 2000), piecewise logistic functions (Zhang et al 2003), or a Savitzy-Golay (SG) filter (Chen et al 2004) to eliminate noise interference. The LSP metrics are then identified using various criteria, including threshold methods (White et al 1997, Piao et al 2006), inflection point detection (Julien and Sobrino 2009), and the maximum of change rate of curvature (Zhang et al 2003). However, there exist large discrepancies among different phenological detection methods (White et al 2009, Shen et al 2014). Therefore, caution should be exercised when using a single method exclusively in interpreting spring phenology.
In this study, we applied three widely-used approaches to extract the start of growing season (SOS) from two different satellite normalized difference vegetation index (NDVI) datasets, including the third generation NDVI (NDVI3g) dataset produced by Global Inventory Modeling and Mapping Studies (GIMMS) (1986–2015) and MODIS (2001–2015). Afterwards, for each dataset, we calculated the mean of the SOS obtained using those three methods. By combining SOS estimates with N deposition data of the continental United States (CONUS), we aimed to investigate whether the changes in N deposition can affect SOS directly or indirectly by altering its responses to climatic factors.
2. Data and methods
2.1. Datasets
2.1.1. Satellite observed NDVI datasets.
NDVI has been widely used in retrieving land surface phenology. In this study, we used two datasets in SOS determination to reduce the uncertainties from the use of a single data source. For each dataset, the average SOS, which was obtained using three separate methods, was used in our analysis. The first dataset is the third generation NDVI (NDVI3g), which is derived from the AVHRR and produced by GIMMS. The GIMMS NDVI3g dataset has a spatial resolution of 1/12°, a 15-day interval, and covers 1982–2015; however, data from 1986 to 2015 were only used to match the N deposition data. The NDVI3g dataset has been corrected to reduce the effects of atmosphere, scan angle, cloud cover, and other factors unrelated to vegetation dynamics (Pinzon and Tucker 2014). The second NDVI dataset is from the MODIS 16-day composite product MOD13C1 (Collection 6) with a 0.05° spatial resolution, and covers 2001–2015. It is generated with strict quality control (Justice et al 1998) and has also been widely used to detect LSP at global scales (Piao et al 2015, Gonsamo et al 2018, Wu et al 2018).
2.1.2. Gridded nitrogen deposition data.
The annual gridded N deposition data for 1986–2015 was obtained from the National Atmospheric Deposition Program (NADP, http://nadp.slh.wisc.edu/), and its spatial pattern is shown in figure 1. This data was produced through a modeled, spatial interpolation of quality-controlled point observation data from National Trends Network (NTN) sites across CONUS having a spatial resolution of 2 km. This data has been used in previous studies (Zhang et al 2018, Gilliam et al 2019).
Figure 1. Spatial distribution of averaged nitrogen deposition in CONUS during the year 1986–2015.
Download figure:
Standard image High-resolution image2.1.3. Climate data.
Monthly temperature and precipitation data were obtained from the Climatic Research Unit Timeseries (CRU-TS) 4.00 dataset with a spatial resolution of 0.5° × 0.5° for 1985–2015 (Harris et al 2014). This dataset has been widely used in previous research on climate change and phenology (Piao et al 2015, Gonsamo et al 2016, Wu et al 2018).
2.2. Determination of land surface phenology
We first excluded the pixels with annual mean NDVI of 0.1 and less to eliminate sparse vegetation areas. To exclude the pixels with low seasonality, we exclusively used the pixels that resulted in the average summer (June–August) NDVI values greater than 1.2 times the average winter (November–January) NDVI values. In addition, cropland was excluded based on the International Geosphere-Biosphere Programme (IGBP) classification in the MODIS land cover product (MCD12C1), because its phenology is largely controlled by human activities. As snow cover affects the accuracy of NDVI-based SOS (Shen et al 2013, Zhang et al 2013), we determined the background NDVI value to eliminate snow cover contamination. To determine the background value, we used the median value of the uncontaminated NDVI values during the non-growing season (from November to March in next year) from multi-year observations. If there was no uncontaminated value during the non-growing season, the mean value from the two nearest uncontaminated pixels was used (Cao et al 2018). The quality layer in the MODIS product and the flag value calculated from the quality layer in GIMMS NDVI3g can provide the information on whether a pixel was covered by snow. This procedure has been successfully applied in previous studies (Zhang et al 2006, Shen et al 2014). The SG filter was then used to smooth the time series of NDVI. Afterwards, we applied three methods to determine SOS and used the mean value of those three dates in the following analyses to reduce the uncertainty of SOS values obtained through a single method.
The first method is the dynamic threshold approach, which uses an annually-defined threshold for each pixel based on the NDVIratio.

where NDVI is the daily NDVI; NDVImax and NDVImin are the annual maximum and minimum of NDVI, respectively. The NDVIratio ranges from 0 to 1. SOS is defined as the day of the year when NDVIratio reaches 0.2 in spring.
The second method is based on a series of piecewise logistic functions. The annual NDVI time series were firstly divided into two parts, i.e. before and after the annual maximum NDVI in each year. Then a four-parameter logistic function (equation (2)) was applied to fit each part.

where y(t) is the NDVI value at day of year (DOY) t, a and b are fitting parameters, c + d is the maximum NDVI value, and d is the initial background NDVI value (Zhang et al 2003). Then the second derivative of the fitted curve was calculated and the date corresponding to its first maximum point was extracted as a date of SOS.
The third method is similar to the second one, but the fitting function was replaced by a double logistic function (equation (4)), which was developed by Elmore et al (2012)
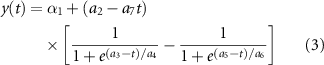
where y(t) is the NDVI at day of year t and a1–a7 are fitting parameters. SOS was also defined as the time when the second derivative of the fitted curve first reaches its maximum value.
2.3. Analyses
We analyzed the satellite time series for three different time periods, for NDVI3g 1986–2015 and 2001–2015, and for MODIS 2001–2015. To match the spatial resolution of MODIS NDVI and GIMMS NDVI3g data, the N deposition data and climate data were resampled using the bilinear interpolation method into the spatial resolution of each satellite data.
While investigating the impact of N deposition on SOS, we used a partial correlation analysis to remove the influence of climatic factors. We calculated the partial correlation coefficient between SOS and annual N deposition of the previous year by keeping the spring (March–May) mean temperature and total precipitation as the control variables.
Moreover, to investigate whether N deposition would indirectly affect SOS by altering its responses to climatic variables, we further analyzed the variations in the sensitivities of SOS to temperature (ST, day/°C) and precipitation (SP, day/mm) along the spatial variations of long-term average N deposition. We first calculated the partial correlation coefficient between SOS and spring mean temperature, with spring total precipitation as the control variable. Similarly, the partial correlation coefficient between SOS and spring total precipitation was calculated, controlling for spring mean temperature. Subsequently, the two-tailed Student's t-test was conducted to identify the significance, and only the pixels with significant level at p < 0.05 were used in the following analyses. In addition, we detected the temporal changes in the relationship between N deposition and ST/SP with a 15-year moving window from 1985 to 2015. The ST and SP were measured as the slope of linear regression between SOS and climatic factors. To investigate whether N deposition would affect the responses of SOS to climatic variables, we calculated average ST and SP in each 0.2 kg ha−1 interval of long-term average N deposition. Considering the simultaneous increase in the temperature, precipitation, and N deposition rate during the past three decades, we conducted spatial partial correlation analysis to avoid pseudo relationships between ST or SP and N deposition. The partial correlation coefficients were calculated between ST or SP and N deposition, while setting spring mean temperature and total precipitation as the control variables (Shen et al 2015). Additionally, hydrothermal conditions (measured by annual mean temperature and total precipitation) may affect the sensitivities of SOS to spring temperature and precipitation. Therefore, we analyzed the variations of ST and SP in different N deposition and hydrothermal gradients, with a temperature interval of 1 °C and a precipitation interval of 50 mm.
3. Results
3.1. Relationship between SOS and N deposition
We found that SOS was significantly correlated (p < 0.05) with N deposition of the previous year in about 5.9% of the pixels across CONUS, based on NDVI3g data during 1986–2015 (figure 2(a)). The significant positive correlations were mainly observed in northern CONUS, accounting for only 1.9% of all vegetated areas. Certain areas in the eastern and western parts of CONUS exhibited significant negative correlations, comprising approximately 3.9% of the pixels. During 2001–2015, the percentage of pixels with significant correlations decreased to approximately 5.0%, and the positive correlations were mainly observed in the central and southern parts of CONUS, whereas the negative correlations were distributed in northeastern and western regions (figures 2(b) and (c)).
Figure 2. Spatial patterns of partial correlation coefficients between SOS and N deposition (a)–(c), and their corresponding frequency distributions (d)–(f). (a) and (d) represent the results from NDVI3g during 1986–2015. (b) and (e) are also from NDVI3g but during the periods 2001–2015. (c) and (f) represent the result from MODIS during 2001–2015. The gray pixels indicate correlations are not significant (p < 0.05). The red and blue bars in (d)–(f) represent positive and negative correlations, respectively, and the solid bars represent the significant (p < 0.05) correlations.
Download figure:
Standard image High-resolution image3.2. Variations in SOS sensitivity to temperature and precipitation with nitrogen deposition
The ST and SP of SOS in CONUS were calculated from two NDVI datasets and their spatial distributions are shown in figure 3. The temperature sensitivity of SOS was significantly negative (p < 0.05) in approximately 52.3% of the pixels during the period 1986–2015. The ST was much higher (exceeded −6 d/°C) in western and northeastern CONUS, indicating that an increase in temperature of 1 °C corresponded to an SOS advance of at least six days in these areas. The central regions were less sensitive to temperature, with approximately −4 to −2 d/°C. Although the majority of pixels are showing the advance of SOS with warming, only negligible amount significantly positive ST were observed (0.4%). The SP of SOS exhibited an evidently different spatial pattern. The regions with significantly positive sensitivity (higher than 0.2 d mm−1) accounted for approximately 10.4% of CONUS and were mainly observed in western regions. In addition, certain areas in the Midwest showed positive sensitivity but had relatively lower values (less than 0.1 d mm−1). However, increased precipitation would advance SOS, which was evident in the negative sensitivity in southwestern parts of CONUS. During 2001–2015, the significant ST was also predominantly negative, but the percentages were reduced to 35.5% and 36.7% for NDVI3g and MODIS datasets, respectively. For the significant SP, both positive and negative sensitivities were observed in those datasets.
Figure 3. Spatial distribution of sensitivity of SOS to spring mean temperature (a), (c), (e) and spring total precipitation (b), (d), (f). (a), (b) represent the results from NDVI3g during 1986–2015. (c) and d are from NDVI3g, but during the periods 2001–2015. (e), (f) represent the result from MODIS during 2001–2015. The gray pixels represent the sensitivities that are not significant at p < 0.05 level.
Download figure:
Standard image High-resolution imageWe then calculated the average correlation coefficient from pixels with significant (p < 0.05) correlations between temperature and SOS in each 0.2 kg ha−1 interval of long-term average N deposition, and found a strong nonlinear relationship between ST and N deposition across space (figures 4(a), (c), (e). SOS was generally more sensitive to temperature in areas with lower N deposition. In regions with N depositions less than 2 kg ha−1, the ST was approximately −6 d/°C. This sensitivity generally decreased to −4 d/°C in areas with N deposition above 4 kg ha−1. Moreover, the significantly (p < 0.05) positive partial correlations between ST and N deposition were also observed when the effects of temperature and precipitation were excluded. Although there were differences among the sensitivity values due to different datasets and time spans, the changes of ST with N deposition were highly consistent.
Figure 4. Spatial variations in sensitivity of SOS to spring mean temperature (a), (c), (e) and spring total precipitation (b), (d), (f) along the gradient of long-term averaged N deposition. (a) and (b) represent the results from NDVI3g during 1986–2015. (c) and (d) are also from NDVI3g but during the periods 2001–2015. (e) and (f) represent the results from MODIS during 2001–2015. The red and blue curves show the values averaged from pixels with significantly positive and negative sensitivities at p < 0.05 level for each N deposition gradient, respectively. The shaded areas represent the mean ± one standard deviation within each N deposition bin. The partial correlation coefficients (Rpartial) were calculated between ST or SP and N deposition, while controlling for temperature and precipitation. ** denotes significance at the p < 0.05 level.
Download figure:
Standard image High-resolution imageClear variation in SP along the spatial gradient of N deposition was also detected (figures 4(b), (d), (f). Considering that both positive and negative SP values were observed, we calculated the average values of the two types of sensitivities, respectively, in each N deposition gradient. During the period 1986–2015, the positive SP continuously decreased from approximately 0.2 d mm−1 to 0.08 d mm−1 with the increase in N deposition (figure 4 (b)). The highest negative SP was observed at N deposition level of 1.0 kg ha−1, and then gradually decreased with increasing N deposition. The spatial variations of SP from both NDVI3g and MODIS during 2001–2015 also showed similar decreasing patterns, although the SP values were relatively larger, and the highest negative SP was found in regions with N deposition of 1.2 kg ha−1 (figures 4(d), (f)). In addition, the partial correlations between SP and N deposition were also significant (p < 0.05).
The temporal changes in the relationships between N deposition and ST/SP also showed patterns similar to those of the spatial changes (figure 5). The change in ST well-corresponded with the change in N deposition over time (figure 5(b)). In general, the absolute values of both the positive and negative SP also decreased with the increasing N deposition (figures 5(c), (d)).
Figure 5. Temporal changes of N depositions, ST, and SP with (a) 15-year moving window for 1985–2015 (a). (b) represents the relationship between N deposition and ST. (c) shows the relationship between N deposition and positive SP, whereas (d) shows the relationship between N deposition and negative SP. **indicates the significance at p < 0.05.
Download figure:
Standard image High-resolution imageWe further investigated ST and SP with the combination of N deposition and hydrothermal gradients. Consistent with the above results, we observed that ST was generally lower in the regions with N deposition of greater than 4 kg ha−1, and its distribution was similar among different datasets and periods (figure 6). In detail, higher ST values were mainly observed in warm (annual temperature >12 °C) and humid (annual precipitation >800 mm) regions with N deposition <2 kg ha−1. Overall, in the same temperature or precipitation zones, the ST gradually decreased with increasing N deposition across space.
Figure 6. Spatial variations in sensitivity of SOS to spring mean temperature (ST) along the gradient of long-term averaged nitrogen deposition, temperature and precipitation. (a), (c), and (e) represent the distribution of ST along the gradient of 0.2 kg ha−1 intervals of nitrogen deposition and 1 °C intervals of temperature. (b), (d), and (f) represent the distribution of ST along the gradient of 0.2 kg ha−1 intervals of nitrogen deposition and 50 mm intervals of precipitation. (a) and (b) are the results from NDVI3g during 1986–2015. (c) and (d) are also from NDVI3g, but during the periods 2001–2015. (e) and (f) are from MODIS during 2001–2015.
Download figure:
Standard image High-resolution imageThe distributions of SP in N deposition and climate space are shown in figure 7. From NDVI3g during 1986–2015, we found that both positive (figure 7(a)) and negative (figure 7(c)) SP decreased to near 0 d mm−1 with the increase in N deposition, but varied little among different temperature zones. This was further supported by the results from different datasets during 2001–2015 (figures 7(e), (g), (i), and (k)). However, both N deposition and precipitation could influence SP. SOS was more sensitive to precipitation in areas with N deposition lower than 2 kg ha−1 and annual precipitation less than 400 mm. With the increase in N deposition and precipitation, both positive (figures 7(b), (f), and (j)) and negative (figures7(d), (h), and (l)) SP significantly declined.
Figure 7. Spatial variations in sensitivity of SOS to spring total precipitation (SP) along the gradients of long-term averaged nitrogen deposition, temperature and precipitation. (a)–(d) are the results from NDVI3g during 1986–2015. (e)–(h) are from NDVI3g, but during the periods 2001–2015. (i)–(l) are from MODIS during 2001–2015. The intervals of nitrogen deposition, temperature, and precipitation are 0.2 kg ha−1, 1 °C, and 50 mm, respectively.
Download figure:
Standard image High-resolution image4. Discussion
In this study, we used partial correlation analysis to investigate the effects of N deposition on satellite-derived SOS in CONUS and revealed that N deposition can explain only approximately 5% of the temporal variation in SOS (figure 2) since spring phenology is principally affected by climatic factors, especially temperature (Menzel et al 2006, Cleland et al 2007, Wu et al 2016). In addition, previous studies observed less contribution of N deposition to vegetation growth. For example, Wu et al (2014) found that only 12.8% of accumulated residual growth of forest in Canada was attributed to N deposition. In addition, a recent study observed that N deposition explained only 9% of the global greening trend (Zhu et al 2016).
Nitrogen is an important element involved in photosynthesis, and thus, a higher N deposition benefits photosynthesis and could advance SOS, which is observed in eastern and western parts of CONUS. However, increasing N deposition would also delay SOS. Previous studies have reported that the budding time and subsequent phenological stages were significantly delayed after N addition (Cleland et al 2006, Wang and Tang 2019), because plant species typically allocate more resources to growth (LeBauer and Treseder 2008) when the available N is increased (Xiang et al 2016). In addition, the low proportion of significant correlation between SOS and N deposition suggests that the effects of N deposition on SOS are complex and may be controlled by human activities, climatic conditions, and vegetation types. Certain previous studies that were based on ground experiments confirmed that the interactive effects of environmental factors (e.g. temperature, precipitation, and snowpack) and N deposition on spring phenology were highly complex and species-specific (Smith et al 2012, Xia and Wan 2013, Liu et al 2017).
Although N deposition showed minimal direct effect on SOS, we observed that it could significantly influence the response of SOS to climatic factors. We observed that both temperature sensitivity (ST) and precipitation sensitivity (SP) of SOS significantly varied across CONUS, but they could be affected by N deposition. Areas with higher N deposition generally had lower temperature sensitivity, suggesting that N deposition will reduce the rate of SOS advancement with climate warming. Similar modeling was also found to occur over time (figure 5). There are two possible explanations for this phenomenon. On one hand, as discussed before, more N addition would delay the development of buds (Cleland et al 2006, Smith et al 2012, De Barba et al 2016, Wang and Tang 2019), whereas increased temperature will advance the growing season. Therefore, the effect of N deposition may be to offset the climate warming effect on SOS, resulting in the decreased sensitivity of SOS to temperature under high N deposition. On the other hand, excessive N deposition would limit the CO2 utilization efficiency of vegetation, causing a reduction in photosynthetic capacity (He et al 2017), thereby suppressing the advancement of spring phenology due to the warming climate. Recent research found that a slowdown of the advancing green-up trend occurred in boreal forests and was largely attributed to the weakening of the warming trends (Park et al 2018). Based on our study, we infer that such a slowdown of SOS may also be due to the increase in N deposition. The SP of SOS showed a similar response to N deposition. Both the positive and negative SP become weaker in the regions with more N deposition. As surplus N deposition may reduce phytohormones (Omarov et al 1999, De Barba et al 2016), the response of SOS to climate change may be weakened accordingly. However, the relationships of these SOS sensitivities to temperature and precipitation with N deposition followed a nonlinear pattern. The sensitivities significantly decreased within the range of approximately 2 to 4 kg ha−1, behaving as a threshold and saturation responses with increasing N deposition. A similar mechanism has also been observed in the responses of carbon sequestration, gross ecosystem productivity, and ecosystem respiration to N addition in previous studies (Fornara and Tilman 2012, Tian et al 2016), indicating that nonlinearity is probably a general feature of ecosystem processes including vegetation phenology. In addition, climatic conditions (e.g. precipitation) would also affect ST and SP (Shen et al 2015). However, in the same temperature or precipitation zones, both the ST and SP still exhibited decreasing trends with the increase in N deposition. A recent study found that the simulated global gross primary productivity increase was overestimated by 85% without N input (He et al 2017). Our study suggests that if the impacts of N deposition on SOS are included in the phenology modules embedded in the terrestrial biosphere models, the accuracy of these models would be further improved.
5. Conclusions
The start of the growing season has been significantly shifted under the background of global warming during the past few decades. Such changes in SOS are triggered not only by temperature and precipitation, but by other environmental factors such as atmospheric N deposition which has been evidently altered due to anthropogenic activities. Using remote sensing data, we derived SOS for the period 1986–2015 in continental United States and investigated its relationship with N deposition. We found that N deposition could only explain the temporal variation in SOS for approximately 5% area of the continental United States. We further analyzed the responses of SOS to temperature and precipitation along different N deposition gradients and found that all these responses became much weaker in regions with higher N deposition. Our results reveal the important role of N deposition variability on spring phenology, which are meaningful for developing the understanding of phenology responses to climate change. Additionally, we suggest that N deposition should be incorporated into ecosystem models that aim to predict carbon cycling that is related to shifting phenology with climate change.
Acknowledgments
We acknowledge National Atmospheric Deposition Program (NADP) for providing the N deposition data. This work was funded by the Strategic Priority Research Program of the Chinese Academy of Sciences (XDA19040103), National Natural Science Foundation of China (41871255, 41901359), and the key Research Program of Frontier Sciences, CAS (QYZDB-SSW-DQC011). A.G. is funded by the NSERC Discovery Grant (Grant No. RGPIN-2020-05708). We are grateful to the anonymous reviewers for their insightful comments and suggestions.
Data availability statement
The data that support the findings of this study are available upon reasonable request from the authors.