Abstract
The contribution of synthetic pesticides to closing yield gaps around the world is undeniable; however, their use is also a classic double-edged sword. Beyond the well-recognized social costs (e.g., pollution to soil and water, and health effects both on consumers and other species) there are also private costs on farmers beyond the direct costs of inputs, associated with elevated risks of both acute and chronic damage to farmers' health, and with the destruction of populations of beneficial organisms. Managing agricultural land use to enhance natural pest control services (also called mobile agent-based ecosystem services or MABES) holds promise to reduce this growing reliance on pesticides, though it too carries costs. In particular, uncertainty in crop yield due to pest damages, as well as the need to coordinate pesticide use with neighboring farms, can be important obstacles to establishing the longer-term public good of natural pest regulation. Current thinking on promoting ecosystem services suggests that payments or other economic incentives are a good fit for the promotion of public good ecosystem services such as MABES. We undertook a framed field experiment to examine the role of subsidies for non-crop habitat in improving insect-based ecosystem services in two separate samples in Southeast Asia—Cambodia and Vietnam. Our central finding is that these two contexts are not poised equally to benefit from incentives promoting MABES, and in fact may be left worse off by payments schemes. As the study and practice of payments for ecosystem services programs grows, this finding provides an important qualifier on recent theory supporting the use of payments to promote public good ecosystem services—where the nature of the coordination problem is complex and nonlinear, farm systems can be made worse off by being encouraged to attempt it.
Export citation and abstract BibTeX RIS

Original content from this work may be used under the terms of the Creative Commons Attribution 3.0 licence. Any further distribution of this work must maintain attribution to the author(s) and the title of the work, journal citation and DOI.
Introduction
The contribution of synthetic pesticides to maintaining crop yields and closing yield gaps around the world is undeniable. However, their use (let alone misuse or overuse, which are not uncommon—e.g., Dasgupta et al 2007, Xu et al 2008, Laary 2012, Ikpesu and Ariyo 2013) is also a classic double-edged sword. Looking to Southeast Asia, which is the focus of this study, beyond the well-recognized social and environmental costs—e.g., pollution to soil and water (Nishina et al 2010, Toan et al 2013), and health effects both on consumers (e.g., Na et al 2006) and other species (e.g., Klemick and Lichtenberg 2008)—there are also private costs on farmers beyond the costs of purchase and labor for application. They include costs associated with elevated risks of both acute and chronic damage to farmers' health (Jensen et al 2011, Pham et al 2011), and costs associated with the destruction of populations of beneficial organisms (e.g., pollinators, natural enemy arthropods or birds). The latter means generally a loss of biodiversity (Tscharntke et al 2005), and more specifically the collapse of natural defense systems that keep pest populations in check (Bianchi et al 2006, Gardiner et al 2009).
Managing agricultural land use to enhance natural pest control services (also called mobile agent-based ecosystem services or, hereon, MABES, Kremen et al 2007) holds promise to reduce this growing reliance on pesticides while preserving farm livelihoods and food security (Barbosa 1998, Landis et al 2000) though it too carries costs. In particular, the potential for pest-related crop damage, as well as the need to coordinate pesticide use with neighboring farms, can be important obstacles to establishing the longer-term public good of natural pest regulation. Given this potential for costs to potentially outweigh benefits in the short term, the question of appropriate policy to overcome such early hurdles (Bell et al 2016a) and promote ecosystem-compatible management approaches in agriculture becomes important. Current thinking on promoting ecosystem services suggests that payments or other economic incentives are a good fit for the promotion of public good ecosystem services (Kemkes et al 2010), and might play a role in improving MABES provision, but here effectiveness varies with context, including the institutional environment. For example, national organic standards have been successful in Thailand and Laos (Schreinemachers et al 2015), yet a lack of regulatory framework in Cambodia or Vietnam means that local efforts at providing standards—such as Vietnam's 'safe vegetable' standard (Van Hoi et al 2009)—do not necessarily garner a market premium.
As a potential alternative encouragement, we develop a framed field experiment to consider a subsidy on the provision of non-crop habitat—land within the agricultural landscape set aside and left undisturbed to promote natural insect enemy populations (Landis et al 2000)—as an example of an economic incentive tied to an easily observed action and thus potentially feasible as an instrument to promote ecosystem-based management in smallholder landscapes. Framed field experiments can extend more traditional survey approaches by eliciting hypothetical responses to a carefully controlled decision environment, representing conditions that (i) may not yet have analogs in past experience and (ii) would be difficult to construct via pilot study or impact evaluation due to costs or confounding effects such as environmental variation (e.g., Alpizar et al 2011). Specifically, we applied a coordination game framed around the land-use decisions that promote or discourage MABES, as part of a larger examination of the potential for MABES provision in the neighboring Southeast Asian nations of Cambodia and Vietnam. These two contexts share a similar reliance on rice production across both wet and dry seasons, but differ greatly in their usage and access to pesticides and pesticide knowledge. Per-hectare pesticide usage in Vietnam rose from 6 to 16 kg ha–1 over the period 2003 2012, with most imported from China, Cambodian pesticide use was close to nil as late as 2008 but rose quickly to 3 kg ha–1 by 2012, with most imports from Thailand or Vietnam (Schreinemachers et al 2015) and labeling rarely translated to Khmer script legible to Cambodian farmers (Jensen et al 2011). Our central finding is that these two contexts are not poised equally to benefit from incentives promoting MABES, and in fact may be left worse off by payments schemes. The findings have broader implications on policies on sustainable agricultural intensification, and highlight how policy outcomes can be significantly shaped by context.
Methods
NonCropShare—a coordination game for insect-based ecosystem services
We applied a version of the published game NonCropShare (Bell et al 2013, 2016b), a symmetric, turn-based coordination game for the provision of insect-based ecosystem services played on tablet computers linked via a mobile hotspot. In NonCropShare, each of the four players takes responsibility for land use decisions on a 3 × 3 grid-cell section (farm) of a 6 × 6 grid-cell agricultural landscape (figure 1). There are four different land-use choices available to players (planting crop without spraying, 'noSpray'; planting crop + light spray, 'lightSpray'; planting crop + heavy spray, 'heavySpray'; adopting non-crop habitat, 'nonCrop'), each with a different distribution of costs and benefits (table 1). In each game turn, players select land use by cycling through land options for each cell (by tapping repeatedly on the cell), and end their turn by 'confirming' their choices when they are ready. Once all players have confirmed their choices, the turn ends, and the 'score' (i.e., the total points earned) is calculated for each cell based on what choices were made in and around the cell, and the process is repeated in the next round. The coordination problem emerges from the interaction of nonCrop and heavySpray: nonCrop cells provide a bonus of +2 to the yield of cells in a Moore neighborhood (vertical, horizontal, and diagonal directions) of 2 cells around them, including across player boundaries (i.e., up to 24 cells potentially affected, depending on the location); heavySpray cells cancel out any benefits from nonCrop in a Moore neighborhood of 1 cell around them (up to 8 cells potentially affected). Thus, nonCrop cells placed along shared boundaries will also have maximally shared benefits (affecting up to 16 cells in neighboring farms if placed at a farm's inner corner), but heavySpray cells placed along shared boundaries can eliminate many of those benefits.
Figure 1. NonCropShare game screen (english version). Upper left corner of landscape is active player; actions taken by other players in previous turns are visible.
Download figure:
Standard image High-resolution imageTable 1. NonCropShare calibration of cost and yield incurred in the cell, and neighborhood effects.
Action | Cost (points) | Yield (points) | Neighborhood effects | |
---|---|---|---|---|
![]() |
Planting crop without spraying (noSpray) | 0 | 5 | None |
![]() |
Planting crop + light spray (lightSpray) | 1 | 5 + 2 | None |
![]() |
Planting crop + heavy spray (heavySpray) | 2 | 5 + 7 | Cancels all non-crop habitat bonuses in a neighborhood of 1 |
![]() |
Non-crop habitat (nonCrop) | 0 | 0 + Xa | Adds 2 points to all squares in a neighborhood of 2 around it. |
aIn some game treatments in this study, a subsidy of X points is awarded for each square of nonCrop, where X is an integer ranging from 1 to 10.
This structure is a stylized representation of real-world processes (natural enemy services, and pesticide drift) meant to capture meaningful decisions without overwhelming participants with detail. As calibrated in table 1, NonCropShare assumes a net benefit to the provision of MABES (i.e., via nonCrop land use), without explicitly addressing potentially offsetting effects (e.g., nonCrop cells harboring pests) or modeling risk or stochasticity in yields. Player scores may range from 45 points (all land in noSpray with no other benefits) or less (if some land is unproductively committed to nonCrop), up to well over 100 points (if players benefit greatly from nonCrop use by others). There are two important equilibria that may emerge from playing NonCropShare in this calibration, in games played without any subsidy for nonCrop. In the first, a Nash equilibrium (where no player stands to do any better by changing strategies, assuming no change by any other player), players can earn a net of 90 points by choosing heavySpray in all grid cells under their control (figure 2(a)). No action taken by any other player on their grid cells can impact this score of 90. In the second, cooperative equilibrium, players can earn a net of 90 points by coordinating the shared benefits of nonCrop, and the use of lightSpray to top off yields without compromising nonCrop benefits (figure 2(b)). Should any player choose to defect from this coordination and choose heavySpray in any cells, it may adversely affect the score achieved by their neighbors (figure 2(c)). Several other cooperative equilibria exist in which scores higher than 90 are possible (illustrated in Bell et al 2016b), though in our experience only graduate students with time and intent have been able to find them.
Figure 2. Key equilibria in NonCropShare.
Download figure:
Standard image High-resolution imageExperimental design
We undertook game sessions of NonCropShare with 512 farmers in 128 game sessions in each of two sites—one in rural Cambodia and one in rural Northern Vietnam. Two enumerators facilitated the experiment in each country, trained by the authors following the same training program. The games are presented in Khmer and Vietnamese on the tablets. Site selection and the sampling of villages across the sites followed a sampling framework established for an accompanying ecological field experiment that aimed to study insect activities in rice systems across a range of landscapes. The site selection captured variation in landscape complexity along a transect of approximately four hours drive leading away from a city. In Cambodia, this transect began in Siem Reap city, while in Vietnam the transect began outside of Ha Noi. A large sample of villages along the transect were visually classified by the level of agricultural landscape complexity (presence of non-managed features and cropping diversity) into categories of low, medium, and high. We obtained a random sample of 32 households from each of the 16 villages along the transect (5–6 villages from each category of low, medium or high). Thus our sample is not representative at any administrative level, but could be considered a quota sample of farms with access to different levels of available ecosystem service associated with land use diversity in the landscape. Variation in ecological complexity is controlled for via village-level dummies in our analysis, but is not examined explicitly in the current study.
Participants joined us for two consecutive half days. In the first day, they completed a detailed household survey, individually. In the second day, they returned to play the NonCropShare game session in groups of 4. An exit poll was conducted after the game session when members of each group rated their familiarity with each other (from 1 to 5, with 1 indicating no familiarity, and 5 indicating a very close relationship) to provide a proxy for the strength of the relationship among the group members. While it is common to undertake a game session first and follow up with a survey, we undertook our game sessions as one of many objectives, including a knowledge assessment of insect-based services in the household survey. We judged the risk of contamination from game to survey to be greater than the other way around and thus administered activities in this order. Participants were compensated approximately equivalent to one day of local labor wage (USD8) for their time, plus a bonus of approximately USD0.50 per 50 points earned in one of the games played during their session (drawn randomly at the end of the session to encourage participants to play each game seriously), all paid out in full upon completion of the game session.
Game sessions began with a short practice session of 3 rounds, followed by four different game treatments, played in random order in each game session. These four game treatments formed a 2 × 2 design of (i) subsidy and (ii) information.
The motivation for the subsidy treatments was to investigate any role a subsidy might play in encouraging better coordination in the provision and utilization of MABES, besides to encourage adoption of nonCrop land use. The calibration of NonCropShare in this study allows players to do equivalently well either by playing selfishly (equilibrium 1), or coordinating (equilibrium 2); the addition of a subsidy allows an evaluation of how much more valuable the coordination equilibrium must be in order to encourage farmers to adopt nonCrop land and the coordination strategy. In games 1 and 2, no subsidies were offered to participants for the adoption of nonCrop; in games 3 and 4, a subsidy from 1 to 10 points (drawn randomly at the beginning of the game and held constant during the game) was offered to participants for each cell of nonCrop.
The interest in information was to verify whether (beyond what kind of long-term interactions are found in a repeated game) leadership helped improve cooperative outcomes. That is, did the ability to observe others' choices in advance of making one's own decisions increase the level of coordination? In games 1 and 3, NonCropShare did not reveal the choices of any player during a round until all players had completed their turn (clicked 'Confirm'). In games 2 and 4, NonCropShare revealed players' choices to other players immediately upon those choices being confirmed by the player.
Players were able to communicate freely regarding any topic throughout the entire game session, including strategy for the game. Players did not receive any strategic advice from the game session leader, with all questions being answered via restatement of the relevant game rules. A complete game protocol is included as appendix
Data analysis
We examined four main game outcomes measured at the group (or 'landscape') level (i.e., the four neighboring 'farms'): (i) total net production (i.e., yields minus spray cost minus subsidy spending) in the landscape, (ii) total production attributable to nonCrop in the landscape (as an indicator of ES provision), (iii) the fraction of nonCrop-attributable production accruing to properties neighboring the property in which nonCrop was adopted (as an indicator of shared benefits), and (iv) the potential nonCrop-attributable production that was canceled by heavySpray squares (as an indicator of issues in coordinating). To explore possible subsidy effects, we looked at other game outcome indicators including counts of heavySpray and nonCrop cells, and diversity in land use strategies. To disentangle within-player from between-player dissimilarities, and following Jost (2006), we measure three different diversities: Alpha diversity (average diversity in choices made by individual players), Beta diversity (diversity between players) and Gamma diversity (diversity across landscape), based on the mix of heavySpray and nonCrop choices. Because these two land uses are direct indicators of the 'Nash' and 'coordination' strategies, respectively, and ought not appear together, these calculations reflect diversity in strategies, rather than simply diversity in land use3 . Thusly, they provide a lens into the scale (player, landscape) at which coherent land-use approaches are appearing; Alpha and Gamma diversities D are calculated directly from land use choices by:
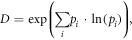
where pi is the proportion of cells in land use i (nonCrop or heavySpray) across the farm or landscape. Beta diversity is then available by the relationship:

Definitions and statistical summary for variables used in the regressions are reported in table 2. All response and explanatory variables are standardized (expressed as z-scores), with the exception of dummy variables to enable the comparison of magnitudes of estimated coefficients within models.
Table 2. Definition and statistical summary for the response (round-level) and explanatory variables.
Raw data | Z-scores | |||||||
---|---|---|---|---|---|---|---|---|
Cambodia | Vietnam | Cambodia | Vietnam | |||||
Description | Mean | SD | Mean | SD | Mean | SD | Mean | SD |
Net production (yield less costs less subsidies) for the current round | 286.51 | 42.52 | 296.07 | 41.44 | −0.12 | 1.01 | 0.11 | 0.98 |
Production attributable to benefits from nonCrop for the current round | 30.25 | 53.05 | 102.45 | 76.95 | −0.5 | 0.7 | 0.46 | 1.02 |
Share of nonCrop-attributable production on properties other than the property nonCrop was planted on for the current round | 0.19 | 0.27 | 0.35 | 0.21 | −0.34 | 1.06 | 0.31 | 0.83 |
Potential nonCrop-attributable production that was canceled in the turn due to HeavySpray squares for the current round | 37.78 | 46.49 | 33.15 | 44.41 | 0.05 | 1.02 | −0.05 | 0.98 |
Number of heavySpray cells | 16.56 | 11.61 | 5.84 | 9.38 | 0.48 | 0.98 | −0.43 | 0.80 |
Number of nonCrop cells | 2.88 | 3.63 | 5.61 | 3.36 | −0.38 | 0.97 | 0.35 | 0.90 |
Beta diversity | 1.20 | 0.27 | 1.19 | 0.25 | 0.03 | 1.03 | −0.02 | 0 .97 |
Alpha diversity | 1.12 | 0.17 | 1.13 | 0.18 | −0.01 | 0.96 | 0.02 | 1.03 |
Gamma diversity | 1.35 | 0.37 | 1.35 | 0.38 | 0.004 | 0.99 | −0.004 | 1.01 |
Average fraction of farms owned across group | 0.42 | 0.24 | 0.91 | 0.12 | −0.84 | 0.77 | 0.76 | 0.39 |
Average farm holding size across group | 2.58 | 3.1 | 13.22 | 24.15 | −0.3 | 0.17 | 0.28 | 1.31 |
Average fraction of crops sold (by mass) across group | 30.84 | 18.33 | 27.29 | 20.6 | 0.09 | 0.93 | −0.09 | 1.05 |
Fraction of group using chemical fertilizers | 0.69 | 0.33 | 0.998 | 0.02 | −0.59 | 1.19 | 0.54 | 0.08 |
Fraction of group using pesticides | 0.29 | 0.28 | 0.85 | 0.27 | −0.74 | 0.72 | 0.68 | 0.69 |
Fraction of the group that is female | 0.46 | 0.29 | 0.45 | 0.26 | 0.02 | 1.05 | −0.01 | 0.96 |
Average age across group | 43.22 | 6.69 | 49.24 | 7.16 | −0.42 | 0.88 | 0.38 | 0.95 |
Average years of formal education across group | 2.19 | 0.66 | 3.94 | 0.57 | −0.86 | 0.61 | 0.78 | 0.54 |
Fraction of group received instruction on pesticides | 0.21 | 0.23 | 0.83 | 0.21 | −0.85 | 0.6 | 0.78 | 0.56 |
Fraction of group aware of pesticide hazards | 0.63 | 0.24 | 0.97 | 0.08 | −0.73 | 0.99 | 0.66 | 0.31 |
Average (self-reported, scale of 1–5) relationship across group | 3.75 | 0.74 | 4.17 | 0.69 | −0.29 | 0.99 | 0.27 | 0.93 |
We conducted two complementary regression analyses. First, we ran a panel (longitudinal) data analysis with both Tobit random-effect (RE) and linear fixed-effect (FE) estimators (Woolridge 2010) to identify the causal effects of exogenous treatment variables, both with and without interacting the treatments of subsidy and information. Variables represent game-level data summed over 8 rounds within each game. The experimental dataset is panel data in nature in which the behavior of groups are observed across rounds (with treatment conditions the same within a game) and games, across which only the randomly assigned treatment changes (and not observable or unobservable player and group characteristics). The information treatment is represented by a binary variable for whether or not information is revealed upon confirmation of choices. For the subsidy treatment, the analysis considers both binary (whether or not a subsidy is given) and discrete random variables (subsidy rate per nonCrop cell, ranging from zero to ten), as participants may respond differently going from zero to non-zero subsidy as compared to getting one unit increase in subsidy rate. We also controlled for rounds (in the entire game session) to capture any learning effect.
Second, we turn to Tobit regressions on the pooled round-level data for group-averaged variables for each country to explore the associations between the observed factors (drawn from the household survey) and the experiment outcomes. The nonlinear regressions are censored on both sides and allow for intragroup correlation. Variables represent group-level data averaged across four participants per group and pooled across all rounds in the entire game session. Comparing regression estimates across countries, we are able to identify clear differences and what factors may have contributed to those differences.
Results
We introduce our findings through two birds-eye comparisons of the datasets from the two countries. First, figure 3 plots the outcome of net production (yields minus pesticide spending minus subsidies) against the number of heavySpray cells in the landscape in a given turn, pooling across all turns from all games in each country (see appendix
Figure 3. Pooled landscape level outcomes across all turns in all games in (a) Vietnam and (b) Cambodia. Mean net levels for the landscape and heavySpray squares in the landscape indicated by the larger red dot. Mean values for net production as well as the number of heavySpray squares differ between Cambodia and Vietnam at p < 0.001 by Kolmogorov–Smirnov and Mann–Whitney tests.
Download figure:
Standard image High-resolution imageSecond, figure 4 plots the landscape net production against total subsidy spending in each round, pooling again across rounds from all games for each of the two countries. These plots illustrate the relationship between landscape net production and total spending on subsidy. If farmers' objectives are to maximize their incomes, then there ought to be a degree of subsidy spending that maximizes landscape net production under an nonCrop approach to land management, above which additional subsidy spending leads to productivity losses, as nonCrop crowds out production in the landscape. This curvature emerges clearly through noisy data for the Vietnam case (figure 4(a)). Landscape net production seems to be maximized, on average, when subsidy spending is around 56. In contrast, landscape net production seems to decline with any non-zero spending on subsidies in the Cambodia case (figure 4(b)).
Figure 4. (a) Net landscape production as function of total subsidy spending in Vietnam. Significance of regression coefficients—***: p < 0.01; **: p < 0.05; *: p < 0.1.(b) Net landscape production as function of total subsidy spending in Cambodia. Significance of regression coefficients—***: p < 0.01; **: p < 0.05; *: p < 0.1.
Download figure:
Standard image High-resolution imageTo try to explain the differences in observed outcomes across the two different samples, we turn to regression analysis of key game outcomes on game structure and characteristics of game players.
The presence of a subsidy has distinct effects on total net production in each of the two countries, significantly raising total net production in Vietnam while reducing it in the Cambodia sample (table 3). This key result is robust to estimation methods and alternative model specifications (e.g., modeling subsidy as a discrete random variable and including the interaction between the subsidy and information treatments) (appendix
Table 3. Game-level total net production in the landscape, modeling subsidy as a binary random variable.
Cambodia | Vietnam | |||
---|---|---|---|---|
(1) | (2) | (3) | (4) | |
Subsidy on non-crop cell (dummy) | −0.147** | −0.152** | 0.133** | 0.128*** |
(0.060) | (0.066) | (0.052) | (0.049) | |
Share info when confirm | −0.015 | −0.011 | 0.058 | 0.058 |
(0.042) | (0.044) | (0.039) | (0.039) | |
Rounds into session | 0.169*** | 0.172*** | 0.206*** | 0.208*** |
(0.027) | (0.029) | (0.030) | (0.025) | |
Constant | −0.054 | −0.048 | 0.028 | 0.033 |
(0.037) | (0.093) | (0.032) | (0.090) | |
Observations (number of games) | 468 | 468 | 512 | 512 |
R-squared | 0.140 | 0.219 | ||
Number of sessions | 117 | 117 | 128 | 128 |
Estimation method | Linear, fixed effect | Tobit, random effect | Linear, fixed effect | Tobit, random effect |
*** p < 0.01, ** p < 0.05, * p < 0.1. Standard errors (clustered at the group level in the linear fixed-effect estimation and bootstrapped in the nonlinear Tobit random-effect estimation) in parentheses. Results on village dummy variables are not reported here. Chow test results are is F(3, 244) = 5.59 (Prob > F = 0.0010) for the Linear, Fixed Effect model and chi2(4) = 20.02 (Prob > chi2 = 0.0005) for the Tobit, Random Effect model.
We observe at the game level a significant learning effect for all 4 outcomes (measured by regressing against the round number associated with each game, where we again emphasize that the order of the four 8-round games was randomized across participants) (tables 3 and 4). As participants played more rounds into the entire game session, they improved overall landscape net production while simultaneously reducing losses due to coordination problems (through reduced cancelled nonCrop points) in both countries. Sharing of nonCrop benefits also decreased overall as the session progressed for both Cambodia and Vietnam. The key difference in learning appears to be that in Vietnam, players tended toward higher benefits attributable to nonCrop in the landscape (see appendix
Table 4. Game-level outcome variables (1) total production attributable to nonCrop in the landscape, (2) fraction of nonCrop-attributable production accruing to properties neighboring the property in which nonCrop was adopted, and (3) the potential NonCrop-attributable production that was canceled by HeavySpray squares, modeling subsidy as a binary random variable and estimated with Tobit random-effect estimator.
Total production attributable to nonCrop in the landscape | Fraction of nonCrop-attributable production accruing to properties neighboring the property in which NCH was adopted | The potential nonCrop-attributable production that was canceled by HeavySpray squares | ||||
---|---|---|---|---|---|---|
Cambodia | Vietnam | Cambodia | Vietnam | Cambodia | Vietnam | |
(1) | (2) | (3) | (4) | (5) | (6) | |
Subsidy on non-crop cell (dummy) | 0.260*** | 0.280*** | 0.537*** | 0.099** | 0.810*** | 0.233*** |
(0.041) | (0.043) | (0.091) | (0.045) | (0.099) | (0.070) | |
Share info when confirm | 0.018 | 0.063** | 0.087 | 0.037 | −0.030 | −0.071 |
(0.028) | (0.032) | (0.061) | (0.037) | (0.060) | (0.051) | |
Rounds into session | −0.053*** | 0.043* | −0.263*** | −0.063* | −0.178*** | −0.184*** |
(0.019) | (0.024) | (0.047) | (0.035) | (0.044) | (0.030) | |
Constant | −0.670*** | 0.314*** | −1.130*** | 0.294*** | −0.426*** | −0.216*** |
(0.056) | (0.079) | (0.150) | (0.091) | (0.101) | (0.083) | |
Observations (number of games) | 468 | 512 | 468 | 512 | 468 | 512 |
Number of sessions | 117 | 128 | 117 | 128 | 117 | 128 |
Chow-test | chi2(4) = 143.33 (Prob > chi2 = 0.0000) | chi2(4) = 77.30 (Prob > chi2 = 0.0000) | chi2(4) = 23.01 (Prob > chi2 = 0.0001) |
*** p < 0.01, ** p < 0.05, * p < 0.1. Bootstrapped standard errors in parentheses. Results on village dummy variables are not reported here.
To understand how the provision of subsidy on nonCrop cells resulted in lower total net production for the Cambodia sample while doing the opposite in Vietnam, we first examine how subsidy affected game outcomes that capture key sources of gains and losses (table 4). Providing a subsidy raised benefits attributable to nonCrop in the landscape in both countries by similar degrees (i.e., 0.26–0.28 standard deviations, on average). Further, the subsidy encourages both (i) more sharing of nonCrop benefits (i.e., more benefits from one farmer's nonCrop accruing to neighboring farms) as well as (ii) more cancellation of potential nonCrop benefits (from heavySpray squares, reflecting a failure in coordination) in both countries. However, the average effect sizes are much greater for Cambodia than for Vietnam—greater levels of sharing, but also greater coordination failure accruing to the presence of a subsidy (columns 3–6 in table 4). These results are generally robust to estimation methods and model specifications (appendix
Pooled round-wise Tobit regression results show that, while subsidy indeed reduced the number of heavySpray cells and increased the number of nonCrop cells in both countries (columns 3–6 in table 5) as expected, the subsidy failed to provide sufficient incentives to turn around the dynamics in land use choices for Cambodia. Moreover, by encouraging more nonCrop cells, inefficiency increased (as captured by a large increase in cancelled potential nonCrop benefits (appendix
Table 5. Round-level outcome variables (1) total net production for the current round, (2) number of heavySpray cells, (3) number of nonCrop cells, (4) diversity within players, (5) diversity between players, and (6) diversity across landscape, modeling subsidy as a binary random variable and estimated with Tobit regressions on pooled data.
Total net production for the current round | Number of heavy-spray cells | Number of nonCrop cells | Diversity within players | Diversity between players | Diversity across landscape | |||||||
---|---|---|---|---|---|---|---|---|---|---|---|---|
Cambodia | Vietnam | Cambodia | Vietnam | Cambodia | Vietnam | Cambodia | Vietnam | Cambodia | Vietnam | Cambodia | Vietnam | |
(1) | (2) | (3) | (4) | (5) | (6) | (7) | (8) | (9) | (10) | (11) | (12) | |
Subsidy on non-crop cell (dummy) | −0.137** | 0.137*** | −0.172*** | −0.098* | 0.889*** | 0.392*** | 0.822*** | 0.114 | 0.205*** | −0.044 | 0.658*** | 0.015 |
(0.055) | (0.045) | (0.037) | (0.059) | (0.100) | (0.053) | (0.119) | (0.095) | (0.063) | (0.042) | (0.108) | (0.087) | |
Enumerator | 0.208*** | −0.061 | 0.179** | 0.097 | −0.015 | −0.278*** | −0.030 | 0.029 | −0.100** | 0.037 | −0.128 | 0.068 |
(0.066) | (0.050) | (0.083) | (0.068) | (0.087) | (0.045) | (0.098) | (0.102) | (0.050) | (0.046) | (0.091) | (0.102) | |
Rounds in game | 0.060*** | 0.026** | 0.028*** | 0.010 | −0.018 | −0.008 | −0.060* | −0.041 | −0.019 | −0.025** | −0.051** | −0.057** |
(0.010) | (0.010) | (0.006) | (0.009) | (0.017) | (0.009) | (0.031) | (0.026) | (0.015) | (0.012) | (0.024) | (0.023) | |
Rounds into session | 0.165*** | 0.184*** | 0.171*** | 0.021 | −0.180*** | −0.064** | −0.247*** | −0.343*** | −0.131*** | −0.072*** | −0.277*** | −0.287*** |
(0.026) | (0.027) | (0.021) | (0.033) | (0.040) | (0.028) | (0.054) | (0.057) | (0.031) | (0.027) | (0.047) | (0.056) | |
Share info when confirm | −0.003 | 0.060* | −0.023 | −0.027 | −0.009 | 0.026 | −0.021 | −0.054 | 0.032 | −0.065* | 0.017 | −0.112 |
(0.040) | (0.034) | (0.033) | (0.039) | (0.067) | (0.039) | (0.075) | (0.087) | (0.041) | (0.036) | (0.069) | (0.074) | |
Average fraction of home property owned across group | −0.027 | 0.128 | 0.032 | 0.272 | 0.055 | −0.355** | 0.104 | −0.755** | 0.102 | 0.069 | 0.183 | −0.351 |
(0.075) | (0.150) | (0.094) | (0.231) | (0.122) | (0.147) | (0.118) | (0.333) | (0.065) | (0.119) | (0.112) | (0.307) | |
Average farm holding size across group | −0.361 | −0.049 | 0.156 | −0.021 | −0.034 | −0.033 | 0.535 | −0.015 | 0.296 | 0.116*** | 0.531 | 0.134** |
(0.233) | (0.031) | (0.297) | (0.045) | (0.306) | (0.043) | (0.343) | (0.077) | (0.206) | (0.026) | (0.326) | (0.061) | |
Average fraction of crops sold across group | 0.224* | 0.137* | 0.320** | −0.103 | −0.189 | 0.013 | −0.015 | −0.334* | −0.088 | −0.123 | −0.098 | −0.346* |
(0.120) | (0.078) | (0.153) | (0.113) | (0.164) | (0.075) | (0.182) | (0.186) | (0.098) | (0.088) | (0.178) | (0.184) | |
Fraction of group using chemical fertilizers | −0.069 | 0.097 | 0.070 | 1.666*** | −0.056 | −0.873*** | −0.177 | 1.141* | 0.099 | 0.217 | 0.024 | 1.123* |
(0.081) | (0.290) | (0.100) | (0.515) | (0.107) | (0.278) | (0.208) | (0.605) | (0.078) | (0.274) | (0.116) | (0.631) | |
Fraction of group using pesticides | −0.132 | −0.181 | −0.042 | 0.384** | −0.043 | −0.238* | 0.470* | 0.432 | −0.174 | 0.094 | 0.093 | 0.346 |
(0.208) | (0.174) | (0.234) | (0.162) | (0.244) | (0.122) | (0.250) | (0.300) | (0.163) | (0.152) | (0.251) | (0.287) | |
Fraction of the group that is female | −0.150** | 0.085 | −0.150** | 0.106 | 0.125 | 0.063 | 0.030 | −0.027 | 0.123** | −0.082* | 0.171* | −0.115 |
(0.071) | (0.052) | (0.075) | (0.079) | (0.083) | (0.056) | (0.096) | (0.103) | (0.056) | (0.050) | (0.094) | (0.105) | |
Average age across group | −0.231*** | −0.088 | −0.125 | −0.100 | −0.001 | 0.096 | 0.178 | 0.225 | 0.146* | 0.060 | 0.251** | 0.192 |
(0.087) | (0.078) | (0.105) | (0.117) | (0.125) | (0.078) | (0.125) | (0.174) | (0.075) | (0.071) | (0.123) | (0.170) | |
Average years of formal education across group | 0.232* | 0.022 | 0.192 | 0.152 | 0.048 | −0.018 | −0.220 | 0.258 | −0.153 | −0.155 | −0.315* | −0.043 |
(0.120) | (0.120) | (0.144) | (0.179) | (0.162) | (0.115) | (0.166) | (0.225) | (0.106) | (0.114) | (0.163) | (0.240) | |
Fraction of group received instruction on pesticides | 0.000 | 0.111 | −0.154 | −0.155 | 0.115 | 0.123 | −0.191 | −0.523* | 0.190 | −0.051 | 0.054 | −0.344 |
(0.217) | (0.120) | (0.247) | (0.205) | (0.263) | (0.109) | (0.263) | (0.286) | (0.175) | (0.121) | (0.258) | (0.256) | |
Fraction of group aware of pesticide hazards | 0.025 | −0.031 | −0.035 | −0.145 | −0.020 | −0.142 | −0.084 | 0.087 | −0.062 | 0.111 | −0.100 | 0.199 |
(0.077) | (0.133) | (0.087) | (0.182) | (0.098) | (0.120) | (0.116) | (0.269) | (0.068) | (0.162) | (0.109) | (0.279) | |
Average relationship across group | −0.127* | 0.115* | −0.271*** | −0.098 | 0.237** | 0.076 | 0.146 | −0.113 | 0.030 | −0.105* | 0.133 | −0.204* |
(0.073) | (0.060) | (0.092) | (0.084) | (0.093) | (0.057) | (0.132) | (0.122) | (0.054) | (0.057) | (0.109) | (0.124) | |
Constant | −2.884*** | 1.926* | −2.222* | −4.841*** | −0.597 | 7.259*** | −0.707 | −2.787 | 1.474* | −1.479 | 1.160 | −3.847* |
(1.003) | (1.059) | (1.215) | (1.449) | (1.161) | (0.960) | (1.364) | (2.093) | (0.807) | (0.972) | (1.337) | (2.143) | |
Observations | 3.744 | 3.936 | 3.744 | 3.936 | 3.744 | 3.936 | 3.744 | 3.936 | 3.744 | 3.936 | 3.744 | 3.936 |
*** p < 0.01, ** p < 0.05, * p < 0.1. Standard errors (clustered at the group level) in parentheses. Results on village dummy variables are not reported here.
The pooled round-level Tobit regression analysis shows additional explanatory factors for game outcomes beyond the game treatments (table 5), with clear differences in the set of factors explaining outcomes across the two sites. Groups with older participants or more female participants tended to achieve lower total net production in the Cambodia sample, while these factors did not appear to have an effect in Vietnam. The use of pesticides and chemical fertilizers in their own farms reduced investment in nonCrop benefits and shared nonCrop benefits while increasing cancellation of potential nonCrop benefits for the Vietnam sample, but this effect was not observed for the Cambodia sample (where input use is much lower overall) (appendix
Perhaps most interestingly, the strength of relationships in the group (the average measure of how well players in the game know each other) encourages nonCrop benefits in both countries (appendix
Discussion
We find that the subsidy on non-crop cells, while changing land use behavior among the Cambodian participants, failed to provide sufficient incentives for the groups to solve the coordination problem, resulting in lower total net production than the case in which no subsidy is offered. In contrast, the Vietnam sample showed a tendency to coordinate and the presence of a subsidy served as additional incentive to boost coordinated action. A natural next question is, why did the different samples behave so differently? Though our experiment was not designed to fully disentangle behavioral causes, we discuss several possible causes.
One possibility is that the two samples were coached differently by their enumerators. This is unlikely because the same experiment protocol was employed in both sites and all four enumerators (two in each country) were trained by the authors directly. Even after controlling for the enumerator effect in the pooled Tobit regressions, the land use choices made by each sample still differ significantly on average. Nevertheless, it is possible that there are some other influences induced by the enumerators that are unobservable to us, which merits follow up research.
A second possibility is to attribute the findings to the underlying behavioral differences in risk preferences and coordination capacity. The two samples differ in many socio-economic factors, including land ownership, farming holding size, use of modern inputs (e.g., chemical fertilizers and pesticides), education, agricultural advisory support, etc (table 2), which may affect people's attitude toward risk as well as ability to coordinate. However, it should be acknowledged that there may well exist factors that are either unobservable to researchers or beyond what household survey or behavioral experiments were able to capture. A better understanding of the institutional environments in which people operate is also warranted.
The results presented are from a game experiment, rather than pilot study or subsidy program, and it is important to acknowledge that validation from game behaviors to real world decisions has been mixed (Levitt and List 2007). Importantly, games (as with any experiment) are better understood as 'trying to accumulate regularity about how behavior is generally influenced by' factors of interest such as rules or incentives, rather than having specific targets in reality against which they ought to be validated (Camerer 2015). In this vein, we consider our NonCropShare results as they relate to the challenge of encouraging community-level coordination of land-use to improve shared services. Farmers may bring many different strategic approaches to this challenge, and of these NonCropShare captures a particular slice—of responses to a subsidy offered on adoption of a practice. Across our study, whether a subsidy on the practice of maintaining non-crop habitat (nonCrop) can improve performance depends on whether the context is positioned to benefit (on average) from a reduction in spraying (i.e., whether groups are close enough to abstaining from heavySpray to make nonCrop effective). There are some parallels here to the environmental Kuznets curve (EKC) (e.g., Dasgupta et al 2002, Stern 2004), which hypothesizes an inverted U-shaped relationship between environmental degradation and income per capita—initially growing worse with development, and then improving as economies are better able to harness cleaner approaches to do the things they do (figure 5).
Figure 5. Production outcomes as a function of landscape spraying, as implied by game data shown in figure 3. Landscapes to the left of the minimum generate benefits from nonCrop (i.e., MABES-based regulation of damages), while landscapes to the right of the minimum generate benefits by heavySpray (i.e., pesticide-based regulation of damages). A landscape whose average level of spraying is shown by the 'A' is made worse off by a small move to the left (less spraying), while a landscape whose average level of spraying is shown by the 'B' is made better.
Download figure:
Standard image High-resolution imageThe opposing effects of the subsidy in the two countries—encouraging attempts to coordinate (and generate nonCrop benefits) but only successfully boosting total net production in one of them—highlight the very different contexts into which any real subsidy program would be launched. The nature of this particular coordination problem—outlined in the U-shaped curve of both panels of figure 3, with nonCrop benefits dropping off sharply with heavy spraying—means that the same nudge that improves conditions in one region (e.g., landscape B in figure 5) could make things worse in another (e.g., landscape A in figure 5). Production outcomes could decline, dealing a loss either to the subsidy provider (if it is continued) or passed along to the farmer (if it is not).
More broadly, our findings are an example of the challenges of improving provision of a particular ecosystem service (the control of crop damage from pests via natural enemies) while simultaneously discouraging the use of synthetic pesticides via the encouragement of a specific, measurable practice (the maintenance of natural enemy habitat). As in many such situations, more is often but not always better; the balance of nonCrop and cropland that maximizes benefits is not obvious from the outset, nor is the appropriate level of encouragement to achieve it. Rather, this 'appropriate encouragement' appears from our data to be context specific (for our Cambodia sample, it appears not to be appropriate at all). The real-world variability in MABES provision that NonCropShare omits—namely the complicated interplay among pest and predator populations enabled by more- and less-disturbed habitats in agricultural systems—could reasonably obscure this appropriate balance further.
On its own, this finding is not new—in reviewing US experiences with market-based policy instruments including subsidies, Stavins (1998) concluded that which instrument is best in any given situation depends upon a variety of characteristics of the environmental problem, and the social, political, and economic context in which it is being regulated. Similarly, Tscharntke et al (2005) suggested that subsidies and agri-environment incentives should better adapt to landscape type, because management changes do not have the same impact on biodiversity and ecosystem services in different landscapes.
Our study identifies an additional twist to the challenge of developing appropriate market-based instruments when the objective is to improve provision of an ecosystem service whose supply is non-trivially linked to a particular practice. 'More' of something is comparatively easy to measure and incentivize, but in the case of MABES provision, 'more' nonCrop is only helpful to a degree, and then only when coordinated carefully with pesticide practice. Similarly in the problem of managing sediment loading to surface waters in agricultural areas, not all ground cover contributes equally to managing the problem (Jack et al 2008); in the challenge of improving biodiversity, 'more' habitat often matters only if it means greater contiguity (e.g., Parkhurst et al 2002), among other features that support biodiversity. In these latter contexts, there is a body of research examining spatial incentives whose value can change with location or proximity to other land-uses (e.g., Drechsler et al 2007, Parkhurst and Shogren 2007), but to date we are not aware of such approaches being applied at scale. Such programs in practice will suffer just as much as others from the challenge of choosing appropriate incentive levels, with the additional political challenge that accrues when those incentives are heterogeneous (and thus one party might stand to gain more than his or her neighbor).
With global ecosystem services in decline, there is an increasing demand for payments for environmental services (PES) schemes, yet this work has only recently begun to be documented comprehensively (Engel et al 2008). Recent work has suggested that provision of ecosystem services that behave as public goods (such as biodiversity and in our case, MABES) can be improved using payments (Kemkes et al 2010). To the extent that games can provide a lens into real-world choices, our study adds an empirical qualifier to this literature by highlighting a clear case where the PES incentive can have perverse effects—where the nature of the coordination problem is complex and nonlinear, farming systems (and possibly farmers themselves) can be made worse off by being encouraged to attempt it.
Acknowledgements
This work was undertaken with financial support from the CGIAR Research Program on Water, Land, and Ecosystems (WLE) and the CGIAR Research Program on Policies, Institutions, and Markets (PIM). We gratefully acknowledge field support from Mr Iv Phirun, Dr Keosothea Nou, Dr Leang Hak Khun, Dr Mai Van Trinh, and Dr Vu Dinh Tuan; as well as design input from Dr Wopke van der Werf and Dr Felix Bianchi.
Appendix A.: NonCropShare game session protocol
Appendix A is available as online supplementary material at stacks.iop.org/ERL/11/114024/mmedia.
Appendix B.: Game-level data analysis results from alternative specifications and estimation methods
Total net production in the landscape | Total production attributable to nonCrop in the landscape | Fraction of nonCrop-attributable production accruing to properties neighboring the property in which NCH was adopted | The potential NonCrop-attributable production that was canceled by HeavySpray squares | |||||||||||||
---|---|---|---|---|---|---|---|---|---|---|---|---|---|---|---|---|
Cambodia | Vietnam | Cambodia | Vietnam | Cambodia | Vietnam | Cambodia | Vietnam | |||||||||
Modeling subsidy on non-crop cell as a binary random variable (dummy) | ||||||||||||||||
Subsidy on non-crop cell (dummy) | −0.147** | −0.152** | 0.133** | 0.128*** | 0.260*** | 0.260*** | 0.280*** | 0.280*** | 0.321*** | 0.537*** | 0.090* | 0.099** | 0.709*** | 0.810*** | 0.219*** | 0.233*** |
(0.060) | (0.066) | (0.052) | (0.049) | (0.043) | (0.041) | (0.046) | (0.043) | (0.062) | (0.091) | (0.049) | (0.045) | (0.084) | (0.099) | (0.065) | (0.070) | |
Share info when confirm | −0.015 | −0.011 | 0.058 | 0.058 | 0.018 | 0.018 | 0.063* | 0.063** | 0.051 | 0.087 | 0.043 | 0.037 | −0.023 | −0.030 | −0.079* | −0.071 |
(0.042) | (0.044) | (0.039) | (0.039) | (0.028) | (0.028) | (0.034) | (0.032) | (0.049) | (0.061) | (0.038) | (0.037) | (0.053) | (0.060) | (0.046) | (0.051) | |
Rounds into session | 0.169*** | 0.172*** | 0.206*** | 0.208*** | −0.053*** | −0.053*** | 0.043* | 0.043* | −0.162*** | −0.263*** | −0.049* | −0.063* | −0.146*** | −0.178*** | −0.147*** | −0.184*** |
(0.027) | (0.029) | (0.030) | (0.025) | (0.017) | (0.019) | (0.023) | (0.024) | (0.030) | (0.047) | (0.029) | (0.035) | (0.038) | (0.044) | (0.033) | (0.030) | |
Constant | −0.054 | −0.048 | 0.028 | 0.033 | −0.670*** | −0.670*** | 0.314*** | 0.314*** | −0.613*** | −1.130*** | 0.323*** | 0.294*** | −0.279*** | −0.426*** | −0.129*** | −0.216*** |
(0.037) | (0.093) | (0.032) | (0.090) | (0.026) | (0.056) | (0.030) | (0.079) | (0.036) | (0.150) | (0.030) | (0.091) | (0.050) | (0.101) | (0.037) | (0.083) | |
R−squared | 0.140 | 0.219 | 0.165 | 0.151 | 0.149 | 0.023 | 0.263 | 0.106 | ||||||||
Including interaction of 'subsidy on non−crop cell (dummy)' and 'share info when confirm' | ||||||||||||||||
Subsidy on non−crop cell (dummy) | −0.138* | −0.141* | 0.150** | 0.144** | 0.287*** | 0.287*** | 0.272*** | 0.272*** | 0.393*** | 0.650*** | 0.049 | 0.057 | 0.728*** | 0.807*** | 0.225*** | 0.230** |
(0.078) | (0.085) | (0.065) | (0.064) | (0.051) | (0.058) | (0.056) | (0.042) | (0.077) | (0.110) | (0.064) | (0.070) | (0.104) | (0.120) | (0.084) | (0.096) | |
Share info when confirm | −0.005 | 0.001 | 0.075 | 0.074 | 0.045* | 0.045* | 0.055 | 0.055 | 0.123* | 0.207* | 0.002 | −0.005 | −0.003 | −0.034 | −0.074 | −0.074 |
(0.053) | (0.055) | (0.058) | (0.061) | (0.024) | (0.025) | (0.049) | (0.047) | (0.064) | (0.107) | (0.063) | (0.065) | (0.053) | (0.069) | (0.058) | (0.056) | |
Subsidy (dummy) * Share info when confirm | −0.019 | −0.023 | −0.035 | −0.032 | −0.054 | −0.054 | 0.015 | 0.015 | −0.145 | −0.226 | 0.083 | 0.083 | −0.039 | 0.007 | −0.011 | 0.006 |
(0.078) | (0.098) | (0.082) | (0.092) | (0.052) | (0.056) | (0.061) | (0.048) | (0.110) | (0.156) | (0.088) | (0.093) | (0.116) | (0.142) | (0.090) | (0.102) | |
Rounds into session | 0.169*** | 0.173*** | 0.206*** | 0.208*** | −0.052*** | −0.052*** | 0.043* | 0.043* | −0.160*** | −0.258*** | −0.048* | −0.062** | −0.145*** | −0.178*** | −0.147*** | −0.184*** |
(0.027) | (0.031) | (0.030) | (0.033) | (0.017) | (0.018) | (0.023) | (0.023) | (0.030) | (0.046) | (0.029) | (0.029) | (0.037) | (0.039) | (0.033) | (0.038) | |
Constant | −0.059 | −0.054 | 0.020 | 0.025 | −0.683*** | −0.683*** | 0.318*** | 0.318*** | −0.649*** | −1.190*** | 0.344*** | 0.315*** | −0.288*** | −0.424*** | −0.132*** | −0.214*** |
(0.043) | (0.092) | (0.038) | (0.076) | (0.025) | (0.050) | (0.035) | (0.081) | (0.038) | (0.119) | (0.038) | (0.095) | (0.051) | (0.083) | (0.043) | (0.073) | |
R-squared | 0.140 | 0.219 | 0.167 | 0.151 | 0.154 | 0.025 | 0.264 | 0.106 | ||||||||
Modeling rate of subsidy on non-crop cell as a discrete random variable | ||||||||||||||||
Subsidy on non-crop cell (rate) | −0.094*** | −0.097*** | 0.023 | 0.019 | 0.168*** | 0.168*** | 0.153*** | 0.149*** | 0.212*** | 0.333*** | 0.061** | 0.062* | 0.403*** | 0.459*** | 0.180*** | 0.191*** |
(0.035) | (0.029) | (0.030) | (0.029) | (0.028) | (0.034) | (0.027) | (0.022) | (0.037) | (0.050) | (0.028) | (0.032) | (0.048) | (0.063) | (0.044) | (0.049) | |
Share info when confirm | −0.016 | −0.011 | 0.057 | 0.057* | 0.020 | 0.020 | 0.060* | 0.060 | 0.054 | 0.094 | 0.042 | 0.036 | −0.017 | −0.021 | −0.082* | −0.073 |
(0.041) | (0.047) | (0.039) | (0.034) | (0.025) | (0.030) | (0.033) | (0.037) | (0.048) | (0.078) | (0.038) | (0.037) | (0.051) | (0.060) | (0.046) | (0.057) | |
Rounds into session | 0.171*** | 0.175*** | 0.213*** | 0.216*** | −0.058*** | −0.058*** | 0.050** | 0.050** | −0.168*** | −0.271*** | −0.047 | −0.061* | −0.153*** | −0.189*** | −0.146*** | −0.182*** |
(0.027) | (0.032) | (0.030) | (0.031) | (0.016) | (0.015) | (0.024) | (0.023) | (0.029) | (0.052) | (0.029) | (0.032) | (0.037) | (0.047) | (0.031) | (0.039) | |
Constant | −0.123*** | −0.119 | 0.096*** | 0.099 | −0.550*** | −0.550*** | 0.462*** | 0.462*** | −0.464*** | −0.880*** | 0.372*** | 0.347*** | 0.053** | −0.046 | −0.010 | −0.088 |
(0.021) | (0.079) | (0.020) | (0.065) | (0.013) | (0.061) | (0.017) | (0.094) | (0.024) | (0.147) | (0.019) | (0.078) | (0.026) | (0.079) | (0.023) | (0.077) | |
R-squared | 0.155 | 0.202 | 0.261 | 0.150 | 0.208 | 0.028 | 0.329 | 0.155 | ||||||||
Including interaction of 'subsidy on non-crop cell (rate)' and 'share info when confirm' | ||||||||||||||||
Subsidy on non-crop cell (rate) | −0.088** | −0.090** | 0.041 | 0.036 | 0.178*** | 0.176*** | 0.172*** | 0.168*** | 0.231*** | 0.363*** | 0.057 | 0.062 | 0.397*** | 0.438*** | 0.197*** | 0.207*** |
(0.043) | (0.043) | (0.038) | (0.037) | (0.032) | (0.024) | (0.034) | (0.029) | (0.042) | (0.065) | (0.039) | (0.039) | (0.057) | (0.063) | (0.058) | (0.054) | |
Share info when confirm | −0.004 | 0.002 | 0.085 | 0.084* | 0.038* | 0.033 | 0.090** | 0.089* | 0.088 | 0.151 | 0.036 | 0.036 | −0.029 | −0.062 | −0.055 | −0.047 |
(0.047) | (0.049) | (0.053) | (0.049) | (0.022) | (0.023) | (0.045) | (0.048) | (0.063) | (0.102) | (0.058) | (0.060) | (0.053) | (0.059) | (0.059) | (0.063) | |
Subsidy rate * Share info when confirm | −0.004 | −0.005 | −0.011 | −0.010 | −0.006 | −0.005 | −0.012 | −0.011 | −0.012 | −0.018 | 0.002 | 0.000 | 0.004 | 0.013 | −0.010 | −0.010 |
(0.014) | (0.015) | (0.014) | (0.014) | (0.010) | (0.010) | (0.010) | (0.010) | (0.016) | (0.025) | (0.016) | (0.017) | (0.020) | (0.023) | (0.019) | (0.017) | |
Rounds into session | 0.172*** | 0.176*** | 0.213*** | 0.216*** | −0.057*** | −0.057*** | 0.050** | 0.051* | −0.166*** | −0.269*** | −0.047 | −0.061** | −0.153*** | −0.191*** | −0.146*** | −0.181*** |
(0.027) | (0.027) | (0.030) | (0.030) | (0.016) | (0.019) | (0.024) | (0.026) | (0.029) | (0.053) | (0.029) | (0.028) | (0.036) | (0.039) | (0.031) | (0.032) | |
Constant | −0.123*** | −0.119 | 0.097*** | 0.100 | −0.550*** | −0.550*** | 0.463*** | 0.463*** | −0.465*** | −0.884*** | 0.372*** | 0.347*** | 0.053** | −0.044 | −0.009 | −0.087 |
(0.021) | (0.100) | (0.020) | (0.069) | (0.012) | (0.048) | (0.016) | (0.072) | (0.024) | (0.141) | (0.019) | (0.066) | (0.025) | (0.074) | (0.024) | (0.081) | |
R-squared | 0.155 | 0.203 | 0.262 | 0.152 | 0.209 | 0.029 | 0.329 | 0.156 | ||||||||
Observations (number of games) | 468 | 468 | 512 | 512 | 468 | 468 | 512 | 512 | 468 | 468 | 512 | 512 | 468 | 468 | 512 | 512 |
Number of sessions | 117 | 117 | 128 | 128 | 117 | 117 | 128 | 128 | 117 | 117 | 128 | 128 | 117 | 117 | 128 | 128 |
Estimation method | Linear, fixed effect | Tobit, random effect | Linear, fixed effect | Tobit, random effect | Linear, fixed effect | Tobit, random effect | Linear, fixed effect | Tobit, random effect | Linear, fixed effect | Tobit, random effect | Linear, fixed effect | Tobit, random effect | Linear, fixed effect | Tobit, random effect | Linear, fixed effect | Tobit, random effect |
*** p < 0.01, ** p < 0.05, * p < 0.1. Standard errors in parentheses. Results on village dummy variables are not reported here.
Appendix C.: Land choices by game and country
Participants of the two samples adopted sharply different strategies, judging by the number of cells that adopted heavySpray versus nonCrop (figure C-1). Overall, sessions in Cambodia on average made significantly more heavySpray choices (p < .000) and less nonCrop choices (p < .000) than their Vietnamese counterparts, regardless of the order of games played (figure C-1(a)) or game treatments (figure C-1(b)). Tellingly, participants in Cambodia adopted much higher number of heavySpray cells from the on-set of the session (i.e., games played in order 1 in figure C-1(a)), whereas the Vietnam sample showed a tendency to coordinate from the beginning.
Figure C-1. Number of cells that adopt heavySpray or nonCrop by order of the game played ranging from 1 to 4 (a) and game treatment (b). 'S', 'NS', 'I' and 'NI' stand for subsidy, no-subsidy, information and no-information, respectively, in (b).
Download figure:
Standard image High-resolution imageAppendix D.: Round-level outcome variables (1) production attributable to benefits from nonCrop for the current round, (2) share of nonCrop-attributable production on properties other than the property nonCrop was planted on for the current round, and (3) potential nonCrop-attributable production that was canceled in the turn due to HeavySpray squares for the current round, modeling subsidy as a binary random variable and estimated with Tobit regressions on pooled data
Production attributable to benefits from nonCrop for the current round | Share of nonCrop-attributable production on properties other than the property nonCrop was planted on for the current round | Potential nonCrop-attributable production that was canceled in the turn due to HeavySpray squares for the current round | ||||
---|---|---|---|---|---|---|
Cambodia | Vietnam | Cambodia | Vietnam | Cambodia | Vietnam | |
(1) | (2) | (3) | (4) | (5) | (6) | |
Subsidy on non-crop cell (dummy) | 0.476*** | 0.308*** | 0.732*** | 0.104** | 0.919*** | 0.185** |
(0.067) | (0.051) | (0.139) | (0.049) | (0.116) | (0.080) | |
Enumerator | −0.045 | −0.237*** | −0.226 | −0.064 | −0.001 | −0.037 |
(0.095) | (0.058) | (0.157) | (0.048) | (0.084) | (0.089) | |
Rounds in game | 0.025** | 0.006 | −0.063* | −0.012 | −0.047* | −0.040** |
(0.011) | (0.009) | (0.035) | (0.014) | (0.025) | (0.020) | |
Rounds into session | −0.156*** | 0.028 | −0.379*** | −0.051* | −0.226*** | −0.262*** |
(0.028) | (0.026) | (0.073) | (0.029) | (0.051) | (0.048) | |
Share info when confirm | 0.041 | 0.061* | 0.117 | 0.030 | −0.017 | −0.113* |
(0.047) | (0.037) | (0.096) | (0.036) | (0.073) | (0.065) | |
Average fraction of home property owned across group | −0.021 | −0.306 | 0.037 | −0.296** | 0.147 | −0.359 |
(0.124) | (0.191) | (0.190) | (0.148) | (0.115) | (0.280) | |
Average farm holding size across group | −0.360 | −0.030 | 0.108 | 0.006 | 0.721** | 0.045 |
(0.306) | (0.043) | (0.644) | (0.029) | (0.317) | (0.061) | |
Average fraction of crops sold across group | −0.228 | 0.123 | −0.463 | 0.100 | 0.013 | −0.351** |
(0.167) | (0.096) | (0.311) | (0.071) | (0.159) | (0.160) | |
Fraction of group using chemical fertilizers | −0.107 | −1.000** | −0.027 | −1.022*** | 0.013 | 1.182** |
(0.115) | (0.390) | (0.177) | (0.332) | (0.128) | (0.553) | |
Fraction of group using pesticides | −0.152 | −0.408** | −0.084 | −0.206* | 0.302 | 0.429* |
(0.222) | (0.159) | (0.362) | (0.122) | (0.251) | (0.243) | |
Fraction of the group that is female | 0.118 | 0.016 | 0.117 | −0.079 | 0.126 | 0.011 |
(0.085) | (0.070) | (0.144) | (0.059) | (0.086) | (0.084) | |
Average age across group | −0.043 | 0.082 | 0.141 | 0.165** | 0.187* | 0.144 |
(0.125) | (0.106) | (0.206) | (0.083) | (0.113) | (0.141) | |
Average years of formal education across group | −0.066 | −0.066 | −0.305 | −0.045 | −0.109 | 0.066 |
(0.164) | (0.151) | (0.277) | (0.119) | (0.158) | (0.192) | |
Fraction of group received instruction on pesticides | 0.317 | 0.168 | 0.378 | −0.024 | −0.202 | −0.285 |
(0.259) | (0.165) | (0.414) | (0.143) | (0.242) | (0.233) | |
Fraction of group aware of pesticide hazards | 0.016 | −0.019 | 0.024 | 0.232 | −0.158 | −0.086 |
(0.096) | (0.180) | (0.174) | (0.155) | (0.098) | (0.213) | |
Average relationship across group | 0.286*** | 0.134** | 0.471*** | 0.043 | 0.076 | −0.148 |
(0.103) | (0.066) | (0.172) | (0.055) | (0.106) | (0.108) | |
Constant | −0.054 | 7.029*** | 1.942 | 2.610** | −0.952 | −1.556 |
(1.217) | (1.235) | (2.106) | (1.014) | (1.182) | (1.813) | |
Observations | 3.744 | 3.936 | 3.744 | 3.936 | 3.744 | 3.936 |
Footnotes
- 3
Diversity calculations based on all four land uses (noSpray, lightSpray, heavySpray, and nonCrop) would not unambiguously show differences in strategy.