Abstract
Electrical load forecasting is an essential foundation for power reliable and economical operation of the power grid. Most forecasting models regard the prediction results as deterministic variables, which ignores the randomness and volatility of the power load. At the same time, insufficient historical load data often lead to undertrained models, which affects the accuracy of capturing uncertain information. Therefore, we proposed an optimized transfer learning-based method for short-term load-interval prediction. A deep learning quantile regression model would be constructed by source domain data in the method, and the weights of the source model would be optimized to avoid negative transfer. Then, the target model is constructed by parameter transfer based on key layers and is tuned with hyperparameters by target domain data. From the experimental discussion, it is known that the model with an optimized transfer learning strategy can accurately quantify the fluctuation range of future power load.
Export citation and abstract BibTeX RIS
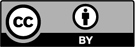
Content from this work may be used under the terms of the Creative Commons Attribution 3.0 licence. Any further distribution of this work must maintain attribution to the author(s) and the title of the work, journal citation and DOI.