Abstract
Safe self-driving vehicles require precise 3D Object Identification. LiDAR sensors are key in accomplishing such a task, as LiDARs produce high-definition point clouds. Such point clouds are then processed by 3D Object Detection models to finally detect objects.
Most Object Detection models require massive amounts of data to be trained. Gathering and processing this data is an expensive and time-consuming task, which is why the information taken from each sample must be fully harnessed. Such can be done through Data Augmentation. Data Augmentation contributes significantly for improving performance, being at least as relevant as the advances in the Object Detection models themselves.
A few studies have been reported regarding the effectiveness of Data Augmentation. However, the role played by original and augmented samples has been neglected. This work reports the first-ever detailed quantification of the impact that the inclusion of original and augmented samples in a dataset has in 3D Object Detection in the context of autonomous driving. The obtained results show that although a good augmentation strategy is crucial to the model's performance, it is only as good as the quality of the original samples allows it to be.
Export citation and abstract BibTeX RIS
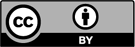
Content from this work may be used under the terms of the Creative Commons Attribution 3.0 licence. Any further distribution of this work must maintain attribution to the author(s) and the title of the work, journal citation and DOI.