Abstract
3D object detection is widely used in the field of autonomous driving. The embedded devices have few resources and low power consumption, so the existing 3D object detection algorithms are difficult to complete the detection task in the required time under some conditions. In this paper, the Complex-YOLOv4-Trans model is proposed to use point cloud data for 3D object detection. Firstly, a transformer encoder block is introduced to improve the structure of Backbone, which makes full use of its context information to improve detection accuracy. Secondly, the general convolution in Backbone is replaced with a depth-wise separable convolution, which effectively reduces the computational load of the model. Finally, Complete-IoU (CIoU) is used as the loss function, and then 8-bit model quantization is performed to speed up the inference. We select the KITTI dataset and deploy the model on NVIDIA Jetson Xavier NX by using TensorRT acceleration tools to test the methods. The mAP of Bird's Eye View for Car is 71.79%, the mAP for 3D Detection is 15.82%, and the FPS on the NX device is 42 frames. Experimental results show that our Complex-YOLOv4-Trans can perform real-time 3D object detection tasks on low-energy embedded devices.
Export citation and abstract BibTeX RIS
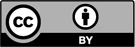
Content from this work may be used under the terms of the Creative Commons Attribution 3.0 licence. Any further distribution of this work must maintain attribution to the author(s) and the title of the work, journal citation and DOI.