Abstract
With the continuous development of the wind industry, wind turbine blade failure causes wind turbine shutdown, which damages the revenue of wind power. In order to more effectively detect the damage on the surface of wind turbine blade. By comparing ResNet-18 /50, VGG-19 and GoogLeNet models, ResNet-18 has the advantages of short training time and high accuracy. Therefore, ResNet-18 convolutional neural network is selected as the basic network, and a deep learning training and detection model is built based on MATLAB. In order to further optimize the model, multiple convolution kernel is selected for the main branch of residual structure of ResNet-18 to extract features, and add 3×3 pool layer, it is used to improve the learning ability of the model. In addition, Aadm, Sgdm and Rmsprop optimization algorithms are added to further improve the robustness of the model. Experiments show that the classification and recognition accuracy of the improved ResNet-18 model for fan blades is 94.17%, which is 2.5% higher than that of the original model. The improved ResNet-18 model has good recognition effect,it improves a faster and more accurate solution for fan blade surface damage identification in complex weather environment.
Export citation and abstract BibTeX RIS
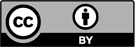
Content from this work may be used under the terms of the Creative Commons Attribution 3.0 licence. Any further distribution of this work must maintain attribution to the author(s) and the title of the work, journal citation and DOI.