Abstract
We present our experience with training and validation of a commercially available deep learning algorithm for organs at risk(OAR) auto-contouring. Computed tomography(CTs) with OARs from a cohort of 213 head and neck(H&N) patients were used for training the deep learning model. A separate cohort of 85 CTs and structure sets was used for validation. All OARs (13) were contoured by a single physician. Metrics such as the DICE similarity coefficient (DSC), Jaccard similarity coefficient (JSC), and volumetric difference (VD) were used to analyze contouring variation. Mean DSC and JSC values ranged 0.48-0.89 and 0.32-0.8, respectively, depending on OAR. A DSC value ≥0.7 indicated low inter-observer variability. In our study, all but one of the contours were above this threshold. DSC for the middle pharyngeal constrictor had the lowest value of all the contours. This may be due to the small volume of this structure. Qualitative assessment of auto-segmented structure samples confirmed the reliability of DSC by demonstrating the compatibility between the expert's evaluation and DSC values. Overall, we found that deep learning auto contouring is a useful tool to speed up the process of contouring in radiotherapy treatment planning.
Export citation and abstract BibTeX RIS
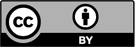
Content from this work may be used under the terms of the Creative Commons Attribution 3.0 licence. Any further distribution of this work must maintain attribution to the author(s) and the title of the work, journal citation and DOI.