Abstract
Liver cancer is one of the highest causes of death in the world. According to IARC data in 2018, the number of cases of liver cancer reached 841,080 cases and 781,631 of them declared dead. One effort that can be done to reduce cases of death from liver cancer is early detection by doing a classification on MicroRNA data. MicroRNA can be used to identify whether a cell is a cancer cell or not at the earliest stages. MicroRNA data studied was obtained from the GDC Data Portal of the National Cancer Institute (NCI). The Deep Neural Network (DNN) method is one of the methods that can be used in classifying cancer. Data normalization in the DNN method brings input values with an unlimited range into a limited range of output values. Data normalization at data pre-processing work more optimally by adding activation functions and Batch Normalization in each hidden layer. Different types of normalization and use of different activation functions can be compared to get the best accuracy value for DNN. This study compares three types of data normalization, namely Min-Max, Sigmoid, and Softmax. Whereas the activation functions being compared are ReLU, Sigmoid, and TanH. The results showed the best accuracy for the classification of liver cancer with MicroRNA data obtained using Min-Max data normalization, ReLU activation function, batch normalization with parameters of 2 hidden layers, 200 epochs, and 0.004 learning rate with an accuracy value reaching 98.33%.
Export citation and abstract BibTeX RIS
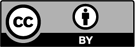
Content from this work may be used under the terms of the Creative Commons Attribution 3.0 licence. Any further distribution of this work must maintain attribution to the author(s) and the title of the work, journal citation and DOI.