Abstract
Identification of rare states in complex dynamical systems remains challenging due to data incompleteness and limited success in adopting standard machine learning algorithms for such problems. Although alternatives such as single-example learning (SEL) can learn novel classes from just a few training examples using similarities to reference classes for which large data sets are available, SEL performance quickly deteriorates with decreasing number of available reference classes. Previously, we have shown that with availability of just two broad classes, vector of boosting-ensemble components, discovered in supervised classification learning, provides universal state representation. Analytical models and other domain-expert knowledge can be directly incorporated within such ensemble decomposition learning (EDL) framework reducing requirements for data size. Alternatively, auto-encoders (AE), components of deep learning (DL) frameworks for automatic feature selection and dimensionality reduction, offer unlimited flexibility in discovering robust latent representations. However, AEs require large data sets and unlike boosting are less effective in utilization of existing models. We argue that using EDL vectors as inputs in standard AE architectures allows synergetic combination of the two approaches and discovery of robust state representations even with limited data. The framework is illustrated in the context of personalized medicine application using cardio data from www.physionet.org.
Export citation and abstract BibTeX RIS
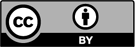
Content from this work may be used under the terms of the Creative Commons Attribution 3.0 licence. Any further distribution of this work must maintain attribution to the author(s) and the title of the work, journal citation and DOI.