Abstract
The objective of the study is to find the best method to construct a model that could predict the future failure as a function of variables obtained from the customer profile. Decision Tree and Logistic Regression are classification algorithm. One of Decision Tree algorithm is Classification and Regression Tree (CART). It can used to analyze numeric and categorical data. Logistic Regression is more accurate than Decision Tree. In fact, there is some missing value in datasets. Amelia II is the best method to estimate missing value for numeric and categorical data. This study combines Amelia II to estimate missing value, Decision Tree to screening and re-categorization variable and Logistic Regression to classifying debtor into 'good' and 'bad' risk classes. We found that the accuracy of this combined method constant until 40% missing value. The Correct Classification Rate (CCR) value for 10% - 40% same as the CCR value for dataset without missing value. Otherwise, the accuracy decreased for missing value above 40%. This method is effective if missing value of the dataset below 40%. We recommend the bank to apply this method for classify risk of debtor if the missing value is below 40%.
Export citation and abstract BibTeX RIS
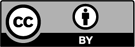
Content from this work may be used under the terms of the Creative Commons Attribution 3.0 licence. Any further distribution of this work must maintain attribution to the author(s) and the title of the work, journal citation and DOI.