Abstract
This study investigated the changes in the toxicity of chemical emissions from the US industrial sector over the 1998–2009 period. Specifically, we employed a multiregional input–output analysis framework and integrated a supply-side index decomposition analysis (IDA) with a demand-side structural decomposition analysis (SDA) to clarify the main drivers of changes in the toxicity of production- and consumption-based chemical emissions. The results showed that toxic emissions from the US industrial sector decreased by 83% over the studied period because of pollution abatement efforts adopted by US industries. A variety of pollution abatement efforts were used by different industries, and cleaner production in the mining sector and the use of alternative materials in the manufacture of transportation equipment represented the most important efforts.
Export citation and abstract BibTeX RIS

Original content from this work may be used under the terms of the Creative Commons Attribution 3.0 licence.
Any further distribution of this work must maintain attribution to the author(s) and the title of the work, journal citation and DOI.
1. Introduction
Toxic chemical management is vital to environmental protection and economic development in industrialized countries (United Nations Environment Programme 2013a) because it reduces the abatement costs associated with the use of toxic chemicals (United Nations Environment Programme 2013b) and minimizes the risk of accidental pollution (Organisation for Economic Co-Operation and Development 2010). Consequently, the management of toxic chemicals has attracted the attention of environmental managers in developing countries, such as China and India, and those in developed countries (Fujii et al 2013, Sharma et al 2014, Sengupta et al 2016). Some studies have examined the factors affecting chemical emission changes in the US (Tang 2015), Japan (Fujii et al 2011), Taiwan (Koh et al 2012) and China (Dou and Sarkis 2013). Most of these studies have examined innovations in the production process (e.g. porter hypothesis) (Ribeiro and Kruglianskas 2015). However, aspects related to toxic chemical emissions that are generated by the product supply chains that connect consumers to producers have not been widely studied (Koh et al 2012).
Figure 1. Research framework of this study.
Download figure:
Standard image High-resolution imageTherefore, a demand-side analysis is important for understanding the countries or sectors that are primarily responsible for the toxic emissions associated with product supply chains and determining why emissions have changed over time. Input–output analyses (i.e. 'demand-driven' input–output analyses) (Leontief 1970) are useful for identifying environmentally responsible countries/industries with higher consumption-based emissions (Hertwich and Peters 2009, Peters et al 2011) and examining the sources underlying changes in environmental loads over time (Lenzen 2016). Numerous studies have been conducted on consumption-based emissions (e.g. CO2 emissions (Kagawa et al 2015, Lan et al 2016), rare metal consumption (Nansai et al 2014, Shigetomi et al 2015) and nitrogen circulation (Oita et al 2016)).
Koh et al (2016) analysed the consumption-based emission amount of chemical substances, although they did not clearly consider the toxicity of the chemical substances. Policy makers typically focus on the toxicity of chemical substance emissions but not on the emission amount itself (Chakraborty and Green 2014) because chemical toxicity directly affects human health and wildlife biodiversity (Romanelli et al 2015). Horvath et al (1995) noted that the chemical toxicity of the most harmful substances affecting humans is more than a hundred times greater than that of the least harmful substances. Thus, simple rankings and time trends regarding the facilities and industries responsible for toxic releases can be misleading because they may neglect relevant toxicological data. Additionally, industrial companies seek chemical substances with low toxicity (e.g. green chemistry) to decrease toxic emissions (Fujii 2016).
Therefore, toxicity reduction, rather than emissions reduction, is a more consistent target due to the toxic chemical management priority of government and industry. In this sense, to develop environmental policies, information about the factors that affect toxicity is more important than information about factors that change the emissions amount.
The objective of this study is to develop a research framework to identify the determinants that change the amount of toxic emissions by focusing on the supply and demand sides. To explain the proposed research framework, we introduce an empirical study using data from US industries. The US industrial sector successfully decreased toxic chemical emissions from 7 trillion tons in 1998 to 1.55 trillion tons in 2009, even though the industrial value added did not decline (see figure S1). Thus, the US industrial sector reduced toxic emissions without a financial sacrifice, which is favourable for sustainable development. Therefore, clarifying the main driver of toxic emissions reduction by the US industrial sector would be helpful in developing toxic chemical management policies in other countries.
The novelty of this study was two-fold: first; it developed a consumption-based accounting framework based on the World multi-regional input–output database (Dietzenbacher et al 2013, Timmer et al 2015) with a focus on toxic chemical emissions; and second, it proposed a new integrated decomposition technique based on a supply-side 'index' decomposition analysis (Ang et al 2003, Ang 2004) and a demand-side 'structural' decomposition analysis (Dietzenbacher and Los 1998, Kagawa and Inamura 2001) to identify the major drivers underlying changes in toxic emissions in the US industrial sector within the context of changes in technology and demand. The consumption-based chemical accounting framework was then further transformed into a 'structural' decomposition analysis (Chang and Lahr 2016). Using these analytical methods developed in this study, we assessed how toxic chemical emissions in the US have been affected by changes in (1) the final demand scale; (2) the production structure, including international trade patterns; and (3) the industrial toxic emission intensities.
We then applied the index decomposition approach (Ang et al 2003, Ang 2004) to clarify the factors affecting the changes in toxic emission intensities in US industrial sectors. Moreover, the toxic emission intensity effects can be decomposed into the following three supply-side factors: cleaner production effects, end-of-pipe effects and chemical toxicity effects. Using this decomposition, we examined how toxic chemical substance management at an industrial level affected industrial toxicity intensities during production. The research framework used in this study is depicted in figure 1.
Notably, the analysis developed in this study includes two types of uncertainties. The first uncertainty comes from which multi-regional input–output database was selected from a wide variety of such databases (e.g. Inomata and Owen 2014). This uncertainty can be called 'wide' uncertainty among the input–output databases used in the analysis.
The second uncertainty comes from how the multi-regional input–output database was constructed by survey and non-survey methods. As a result, the estimated input–output database in question includes statistical errors (e.g. Dietzenbacher 2006). This uncertainty can be called 'narrow' uncertainty in the sense that we here focus on only errors included in a specific database.
Although the global uncertainty analysis considering the two uncertainties is important in evaluating the validity of the estimated consumption-based toxic chemical emissions during the study period, this study reports only the result of the second type of the uncertainty analysis using the World multi-regional input–output database (Dietzenbacher et al 2013, Timmer et al 2015) because we could not obtain a comparable set of world multi-regional input–output databases and conduct the first type of uncertainty analysis. This is a limitation of this study.
The remainder of this paper is organized as follows: section 2 provides the method; section 3 explains the data used in this study; section 4 presents and discusses the results; section 5 provides the result of the uncertainty analysis; and finally, section 6 concludes the paper.
2. Method
2.1. Choice of decomposition analysis model
Decomposition analysis is widely applied in energy and environmental research fields to clarify the driving forces or determinants underlying these changes (Wang et al 2017). According to Hoekstra and van den Bergh (2003), two major techniques that can identify changes in decomposition indicators at the sector level are structural decomposition analysis (SDA) and index decomposition analysis (IDA).
SDA was originally developed as an input–output analysis for understanding the change in consumption-based emissions (e.g. carbon footprint). One of the main advantages of SDA is that it clearly considers the economic system, including trade patterns, the combination of intermediate material inputs, and the scale of final demand.
IDA was developed in energy and environmental research fields to understand the intensity change caused by technological development, such as that of emission intensity due to resource efficiency (Wang et al 2017). IDA has several estimation options, including the Laspeyres index and logarithmic mean Divisia index (LMDI) (Ang 2015).
Notably, our decomposition analysis is divided into two steps: SDA is used for demand-side effects analysis (the first step), and IDA is used for supply-side effects analysis (the second step). We primarily use two different methods to distinguish the change in toxic intensity (the technology factor) and demand-side effects (the economic system factor).
In addition, IDA is advantageous in terms of decomposing emission intensity change. According to Wang et al (2017), SDA is beneficial when analysing demand-side effects and trade-related issues. By contrast, IDA is preferred for analysing the change in emission intensity because it is more flexible in modelling and easier to implement and understand compared with SDA.
2.2. Structural decomposition of toxic emissions in the US
The toxicity of emitted chemical substances in the US induced by the final demand of country k in year t, Qt,kUS, is expressed in equation (1):

where Tt is the row vector consisting of the toxic emission intensity (i.e. toxicity of chemical emissions (unit: tons) per industrial output (unit: one thousand dollars) in the US), Lt = (I − At)−1 is the Leontief inverse matrix, At is the input coefficient matrix in the multiregional input–output table for year t, I is the identity matrix, and ft,k is the column vector consisting of the final demand (unit: one thousand dollars) of country k in year t (Miller and Blair 2009). Based on the number of industries M and countries N, Tt,At and ft,k can be expressed as follows:

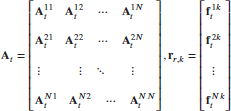
where Ti,tUS(i = 1, ⋯ ,M) is the toxic chemical emissions per industrial gross output in each industrial sector i in the US in year t, Atrs is the input coefficient matrix from country r(r = 1, ⋯ , N) to country s(s = 1, ⋯,N) in year t, and ftrk(r = 1, ⋯, N) is the vector of final demand from country r to country k.
Equation (1) calculates the toxicity of emitted chemical substances in the US through the global supply chains induced by the final demand of country k. It should be noted that Tt is negatively affected by management efforts focused on reducing toxic chemical substances in US industrial sectors only, whereas At and ft,k are affected as entire supply-chain networks by changes in production technology and lifestyles of the different countries, respectively.
We set the change in the consumption-based emissions of chemical substances during year t and year t+1 as ΔQkUS = Qt+1,kUS−Qt,kUS, and these factors were decomposed into the three effects shown in equation (S1) in supplementary method 1 available at stacks.iop.org/ERL/12/124008/mmedia: the changes in toxic emission intensity (i.e. ΔIntensity), in production structure (ΔSTR), and in the final demand scale (ΔScale) (Sun 1998).
Changes in the toxic emissions intensity effects (ΔIntensity) are attributed to changes in the toxic emissions per industrial gross output in the US. The structural change effects (ΔSTR) are attributed to changes in the structure of industrial production at a national scale. Notably, this structural effect includes the changes in the international trade patterns of intermediate products or national production technologies. Change in the final demand effects (ΔScale) are directly affected by changes in the scale of the final demand in each country.
The final demand effects and the production structural effects can be considered demand-side effects, whereas the toxic emissions intensity effects can be considered supply-side effects. To further clarify the effect of toxic chemical management by US industries, the changes in toxic emissions intensities are decomposed further in the following section.
2.3. Index decomposition of toxic emissions intensities in the US
A decomposition framework for the chemical emissions in industrial production processes has been proposed by Fujii and Managi (2013). By referring to their framework, the change in the toxic emissions intensity of industry i in the US, Ti,tUS, can be further decomposed into the following three factors: (1) end-of-pipe (EOP) treatment, (2) cleaner production (CP) and (3) the toxicity of emitted chemicals (TEC).
To apply this decomposition approach to the US, this study used two variables for the toxic chemicals: total release by industry i in the US in year t (Ei,tUS) and total waste management by industry i in the US in year t (Mi,tUS). These data are obtained from the US toxic release inventory. Using these two variables, we calculated the total amount of toxic chemical substances generated by industry i in the US in year t (Gi,tUS), and we herein define Gi,tUS = Ei,tUS + Mi,tUS. Yi,tUS as the gross output in industry i of the US in year t deflated to the 1995 price.
First, the industrial CP indicator in the US, which is defined as , represents the generation of toxic chemicals per industrial gross output. This indicator can be decreased by reducing the generation of toxic chemical substances while maintaining industrial production. The reduction in CP can be achieved by improved production processes and product designs that reduce the input of intermediate chemical materials.
Second, the industrial EOP indicator in the US, which is defined as , represents the share of emissions out of the total amount of toxic chemical substances generated. This indicator can be reduced by increasing the waste management of total toxic chemicals. Finally, the industrial TEC indicator in the US, defined as
represents the toxicity intensity of emitted chemical substances. Zi,tUS is the toxic emissions in industry i of the US in year t. Here, the toxicity per gross output of industry i in the US (
) was decomposed in equation (2).
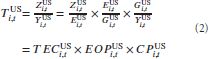
Next, we define the change in the toxic emissions intensity as ΔTiUS(= Ti,t+1US−Ti,tUS) and obtain equation (3).
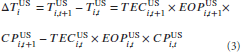
Similar to the decomposition approach to the induced effects in equation (1), we apply the Laspeyres decomposition analysis to equation (3) and decompose ΔTiUS into three effects: the changes in EOP treatment, the changes in CP, and the changes in TEC (see equation (S4) in supplementary method 2).
2.4. Uncertainty analysis for consumption-based toxic emissions
The World input-output database is conventionally compiled through non-survey methods; therefore, the obtained input coefficient matrix tends to contain measurement error problems in the observed transaction tables (Roland-Holst 1989).
As in Roland-Holst (1989) and Dietzenbacher (2006), we conducted a Monte Carlo experiment to examine whether the measurement error in the input coefficient matrix yields a significant input-output multiplier bias and whether such a multiplier bias consequently leads to substantial uncertainty regarding consumption-based chemical toxicity. Monte Carlo analysis has the advantage of applicability to simulations based on a variety of actual transaction tables (Roland-Holst 1989).
As in Dietzenbacher (2006), we see that the 'observed' intermediate input matrix D = (dij), the 'observed' row vector of toxic emissions Z = (ZjUS), and the 'observed' column vector of final demand f = (fi) are assumed to be biased as follows:

with

where k = 1,2,..., K. Here, k is the sample size of the Monte Carlo method, and we set K = 100 and ρ = 0.1, as in Dietzenbacher (2006). Notably, the sample size of the Monte Carlo method must be set at the relatively small number of 100 due to the larger data size of the World Input–Output Database. The error terms of εijk,δjk, and εik follow normal distributions. In this multi-regional input–output table including data noise, the gross outputs include data noise as and therefore the obtained intermediate input coefficient matrix also includes it as Ak = (aijk) = (dijk/Yjk).
Then, the Leontief inverse matrix (i.e. direct and indirect input requirement coefficients) and the row vector of toxic emission intensity, including data noise, are calculated as Lk = (lijk) = (I − Ak)−1 and Tk = (TjUS,k = (ZjUS,k/YjUS,k), respectively where YjUS,k is the gross industrial outputs of the US including data noise.
When we set the unknown 'true' values of lij, TjUS, and fi*, their t-statistics are obtained as follows:

respectively, where we have

with
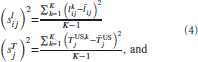
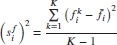
The t-statistics above can provide 95% confidence intervals for the direct and indirect input requirement coefficients, toxic emission intensities, and final demand; therefore, these upper values can be used to estimate the upper value of consumption-based toxic emissions with equation (1), whereas the lower values provide the lower value of consumption-based toxic emissions.
3. Data
3.1. Toxic emissions data
Toxic chemical emissions data from the US industrial sector are obtained from the toxic release inventory (TRI) database published by the US Environmental Protection Agency (US EPA 2017). The TRI was created by Section 313 of the Emergency Planning and Community Right-to-Know Act in 1986. It is a publicly available database that contains information on toxic chemical releases and waste management activities, which is reported annually by certain industries and federal facilities.
In addition, chemical data on seven industries, which were added to the TRI programme in 1997, only became available starting in 1998. These newly added sectors included the mining sector and the electric power sector, which produce large amounts of chemical emissions. Therefore, we set the research periods from 1998–2009 to ensure that we considered these sectors as well.
We used US emissions data for 588 chemical substances with risk–screening environmental indicators (RSEI) as proxies for the toxicities of chemical substances (US EPA 2015). The emissions data cover toxic chemical releases to all media. Horvath et al (1995) introduced the TRI as the database with the most comprehensive and widely reported information on hazardous discharges into the environment in the United States.
Additionally, Toffel and Marshall (2004) investigated the comparative analysis of toxic weighting methods for the TRI database. They compared 13 methods including RSEI, human toxicity potential, the Indiana Relative Chemical Hazard Score, and so on. Toffel and Marshall (2004) concluded that RSEI is the recommended weighting method for integration of TRI data because of greater coverage of TRI chemical substances. Many previous studies have used the TRI and RSEI to evaluate toxic chemical substances in environmental indicators (Velagapudi et al 2017) and their human health impact (Lewis and Bennett 2013) and as parts of accounting research (Cong et al 2014).
To integrate the toxicities of different chemical substances, we applied the RSEI published by the US EPA (2015). The integrated toxicity score (Zi,tUS) for chemical substances generated by a specific sector i in the US in year t was estimated by equation (5):

where j denotes a specific chemical substance. The toxic emissions intensity of 16 sectors between 1998 and 2009 in the US were estimated by dividing the sectoral integrated toxicity score by the sectoral gross output (table S1 for the sector classification). According to the US EPA (2015), the toxicities estimated by equation (5) are hazard-based results, which differ from toxic 'risks' to human health that clearly consider the population density of the region of emissions. Therefore, the toxicity scores in this study focus on the 'toxicity hazard emission' or 'toxicity hazard embedded in production processes'. Using the hazard-based toxic emissions data, we can evaluate how the US sector applied toxic chemical management in their production processes considering the toxicity of chemicals.
The list of toxic chemical substances in the TRI changed with time. To analyse the change in toxicity using time-series data, we applied the toxic chemical data listed in the 1998 core chemical substances published by the US EPA (see table S2). Therefore, the toxic scores in different years are estimated using the same coverage of chemical substances. Thus, we can compare the toxicity data directly between different years.
It should be noted that TRI data are subject to limitations and uncertainty. The US EPA (2009) investigated the uncertainty involved with seven major environmental indicators, including TRI. The US EPA (2009) noted that 'TRI data reflect only 'reported' chemicals, not all chemicals with the potential to affect human health and the environment. TRI does not cover all toxic chemicals or all industry sectors. The following are not included in this indicator: (1) toxic chemicals that are not on the list of approximately 650 toxic chemicals and toxic chemical categories, (2) wastes from facilities within industrial categories that are not required to report to TRI, and (3) releases from small facilities with fewer than 10 employees or that manufactured or processed less than the threshold amounts of chemicals.'
In addition to these limitations, TRI data are subject to uncertainty due to facility-level errors caused by the diverse arrays of methods and information sources used by facilities to prepare their TRI reports (US EPA 2009). The uncertainty involved with self-reported environmental data has also been noted by de Marchi and Hamilton (2006). They find that large decreases in air emissions reported by firms in the TRI are not always matched by similar reductions in measured concentrations by EPA monitors.
3.2. World input-output data
We used the World Input–Output Database (WIOD), which covers 41 countries and regions and 17 industries between 1998 and 2009 (see table S1) (Dietzenbacher et al 2013, Timmer et al 2015). Following Temurshoev et al (2013) and Los et al (2014), the World input–output tables for 1998–2009 were deflated to the tables based on 1995 US dollars using price deflators and the generalized RAS method.
We should note our limitation related to data availability. The WIOD released data from its 2016 version, which covers 2000 to 2014. However, the WIOD did not release the socio-economic data, including price index data. Thus, we find translating the database into real terms, which is needed for time-series analysis, difficult. Additionally, the WIOD released data from its 2013 version, which covers 1995 to 2011. Moreover, the WIOD only releases price index data from 1995 to 2009 from the 2013 version of its socio-economic accounting database. Based on these available data, we create an input–output table data in real terms for 1995 to 2009.
Although the WIOD includes the time-series data from 1995 to 2009, we used the data from 1998 because of the consistency of the data for toxic chemical substances in the TRI in the US. As a result, we compiled the environmentally extended world input–output tables from 1998–2009. Because industries are clarified differently between the TRI and WIOD, we integrated the TRI industry using North American Industry Classification System (NAICS) codes (see table S3).
4. Results
4.1. Consumption-based chemical toxicity in the US
Table 1 shows the toxic emissions from the five largest industries associated with the final demand of countries around the World in 1998 and 2009 (table 1). First, we examined the magnitude of chemical pollution in 1998 and 2009. In 1998, the total amount of toxic chemicals generated by the five industrial sectors in the US accounted for 5743 billion tons (table 1). Interestingly, the induced chemical toxicity (i.e. consumption-based chemical toxicity) decreased by 4779 billion tons during the decade from 1998–2009, implying that US industries have contributed to the rapid decrease in chemical toxicity observed in the US.
Table 1. Induced toxic emissions of US industrial sectors associated with final demand by country.
World | US | Canada | Mexico | EU | Japan | BRICs | Others | |||||||||
---|---|---|---|---|---|---|---|---|---|---|---|---|---|---|---|---|
Year | Industry | billion | billion | % | billion | % | billion | % | billion | % | billion | % | billion | % | billion | % |
tons | tons | tons | tons | tons | tons | tons | tons | |||||||||
1998 | TOTAL | 5743.586 | 4896.027 | 85.243% | 126.780 | 2.207% | 56.150 | 0.978% | 213.115 | 3.710% | 93.417 | 1.626% | 67.453 | 1.174% | 290.645 | 5.060% |
MINING | 4600.025 | 3990.320 | 86.746% | 89.241 | 1.940% | 37.176 | 0.808% | 150.248 | 3.266% | 73.081 | 1.589% | 49.477 | 1.076% | 210.483 | 4.576% | |
CHEMICALS | 249.838 | 191.418 | 76.617% | 7.172 | 2.871% | 5.039 | 2.017% | 18.202 | 7.286% | 5.629 | 2.253% | 6.264 | 2.507% | 16.114 | 6.450% | |
METALS | 304.626 | 234.268 | 76.903% | 12.029 | 3.949% | 7.263 | 2.384% | 16.750 | 5.499% | 6.083 | 1.997% | 5.220 | 1.714% | 22.968 | 7.540% | |
TRANSPORT | 316.110 | 235.494 | 74.497% | 14.072 | 4.452% | 4.085 | 1.292% | 20.199 | 6.390% | 5.583 | 1.766% | 4.267 | 1.350% | 32.410 | 10.253% | |
UTILITIES | 137.265 | 129.458 | 94.312% | 1.044 | 0.761% | 0.590 | 0.430% | 2.179 | 1.587% | 0.862 | 0.628% | 0.635 | 0.463% | 2.497 | 1.819% | |
2009 | TOTAL | 964.171 | 839.117 | 87.030% | 13.754 | 1.427% | 7.989 | 0.829% | 36.813 | 3.818% | 10.667 | 1.106% | 19.201 | 1.991% | 36.586 | 3.795% |
MINING | 648.425 | 589.597 | 90.928% | 6.809 | 1.050% | 2.633 | 0.406% | 19.155 | 2.954% | 6.264 | 0.966% | 7.535 | 1.162% | 16.432 | 2.534% | |
CHEMICALS | 103.539 | 71.946 | 69.487% | 2.814 | 2.718% | 2.542 | 2.455% | 10.077 | 9.733% | 2.270 | 2.192% | 5.674 | 5.480% | 8.171 | 7.892% | |
METALS | 71.084 | 50.884 | 71.583% | 2.406 | 3.385% | 1.861 | 2.618% | 4.085 | 5.747% | 1.135 | 1.597% | 3.904 | 5.492% | 6.809 | 9.579% | |
TRANSPORT | 12.574 | 8.897 | 70.757% | 0.545 | 4.334% | 0.227 | 1.805% | 0.953 | 7.579% | 0.182 | 1.447% | 0.454 | 3.611% | 1.362 | 10.832% | |
UTILITIES | 99.726 | 93.916 | 94.174% | 0.590 | 0.592% | 0.363 | 0.364% | 1.453 | 1.457% | 0.454 | 0.455% | 0.953 | 0.956% | 1.952 | 1.957% |
Note: BRICs includes Brazil, Russia, India, and China.
Figure 2. Accumulated changes in toxic emissions by driving factors. Note: Each acronym represents the following: ΔScale is the change in the final demand effects, ΔSTR is the changes in the structure of industrial production, ΔTEC is the change in the toxicity intensity of emitted chemical substances, ΔEOP is the changes in end-of-pipe treatment effects, and ΔCP is the changes in cleaner production effects.
Download figure:
Standard image High-resolution imageNext, we discuss the regional distribution of consumption-based toxic emissions by examining several countries and regions that induce significant toxic emissions in the US. Table 1 shows that most of the toxic emissions in the US industrial sector were triggered by US domestic final demand. The share of US final demand increased slightly from 85.2%–87.0% from 1998–2009. Thus, 13.0% of the toxic emissions from the US industrial sector were triggered by the final demand of other countries outside the US in 2009.
The contribution of the final demand of BRIC countries (Brazil, Russia, India and China) to US toxic emissions increased from 1.2%–2.0% from 1998–2009, whereas that of other countries decreased or remained steady over the same period (see figures S2 and S3 in the supplementary information for the geographical distribution).
The data in table 1 indicate that the contribution of each industry to the final demand differed among countries and regions because different countries have their own relative industrial strengths in the global market as well as different objectives and characteristics that drive the importation of US products as intermediate goods or final products.
4.2. Factor decomposition analysis of the change in toxic emissions in the US
The driving forces underlying changes in consumption-based chemical toxicity and chemical toxicity transferral can be captured using economic factors, such as the industrial structure, international trade structure, final demand structure, and production technology.
Figure 2 shows the trends for consumption-based toxicity in the US. The plotted line shows the changes in the consumption-based toxicity of the US as a ratio of that in the base year 1998, and the bar chart shows the effects of economic factors on the consumption-based toxicity of the US. The summation of the bar chart score is equivalent to the value of the plotted line.
From figure 2, consumption-based toxic emissions in the US decreased by approximately 83% from 1998–2009, and an 80% reduction was achieved by 2004. The main drivers for this rapid reduction were the observed decreases in TEC and EOP treatment, which together accounted for a 66% reduction. Thus, pollution abatement technologies and initiatives in the US industrial sector have markedly contributed to the reduction in toxic emissions in the US. In addition, changes in the production structure (STR) and CP methods contributed to decreasing the toxicity by 24% and 17%, respectively.
Next, we consider the changes in induced toxicity in each industrial sector. Table 2 shows the changes in the induced toxic emissions in the US industrial sector related to increased product demand in 41 countries and regions. The trading-factor results were estimated by the environmentally extended multi-region input–output analysis model, and the breakdown of toxic emission intensity change (ΔT) was calculated by a further decomposition analysis using the Laspeyras index decomposition approach. The LMDI is an alternative approach for further decomposition analysis (see supplementary method 3) (Lan et al 2016). To confirm the robustness of the results obtained by further decomposition, we compared the estimation scores obtained using the Laspeyres index and LMDI approaches. Table S4 in the SI describes the results of these further decomposition approaches, and the results appear to corroborate the obtained results.
Table 2 shows that the induced toxic emissions of the US industrial sector decreased by 83% from 1998–2009. The main driver of this dramatic reduction is a decrease in the toxic emissions intensity (i.e. toxic emissions per industrial gross output). These findings imply that the reduction in induced toxic emissions was achieved by decreasing the toxic emission intensity in the US industrial sector. Compared with the demand-side effects, we found that the supply-side effects exert a markedly stronger influence on the changes in induced toxic emissions in most industries and countries (see table 2 and table S5 in SI). This trend differs from that reported in previous studies that focused on consumption-based increases in CO2 emissions and concluded that consumption scale is the main driver of changes in emissions (Kagawa et al 2015). One possible reason for the observed disparity is that toxic chemicals can be managed by EOP, which is a difficult approach to inducing reductions in CO2 emissions. Another reason is the marked difference in toxicity among chemicals, which means that substituting a highly toxic chemical with a less toxic chemical can cause a marked reduction in toxic emissions. The finding that supply-side effects lead to a greater reduction in induced toxic emissions relative to demand-side effects is explained by the simultaneous application of supply-side and demand-side approaches.
Table 2. Decomposition of changes in US chemical toxicity from 1998–2009.
Change in toxic emissions | Demand-side effect | Supply-side effect | colspan="3">Breakdown of Δ Intensity | |||||
---|---|---|---|---|---|---|---|---|
Δ Scale | Δ STR | Δ Intensity | Δ TEC | Δ EOP | Δ CP | |||
billion tons | % | % | % | % | % | % | % | |
TOTAL | −4779.424 | −83.213% | 23.334% | −24.153% | −82.395% | −40.699% | −25.066% | −16.630% |
MINING | −3951.573 | −85.904% | 26.763% | −27.068% | −85.598% | −5.322% | −0.702% | −79.574% |
FOOD | 0.517 | 74.001% | 17.146% | −12.001% | 68.857% | 67.454% | −222.802% | 224.204% |
TEXTILES | −0.118 | −57.104% | −31.488% | −56.743% | 31.128% | 95.107% | −109.508% | 45.529% |
LEATHER | −8.161 | −79.285% | −32.688% | −20.189% | −26.408% | 39.277% | −9.997% | −55.689% |
WOOD | −0.522 | −51.124% | −6.221% | −16.933% | −27.969% | 100.694% | −71.127% | −57.537% |
PAPER | −32.024 | −91.408% | 7.380% | −11.104% | −87.683% | −55.944% | −27.448% | −4.291% |
FUEL | −39.337 | −72.987% | 14.936% | −9.993% | −77.930% | −67.360% | 20.508% | −31.078% |
CHEMICALS | −146.321 | −58.566% | 15.620% | −14.106% | −60.080% | −26.148% | −22.999% | −10.933% |
RUBBER | −1.357 | −80.407% | 12.491% | −21.869% | −71.029% | −4.095% | −38.309% | −28.624% |
MINERALS | −5.433 | −55.292% | 5.711% | −23.931% | −37.071% | −13.334% | 68.607% | −92.344% |
METALS | −233.565 | −76.669% | 9.686% | −18.161% | −68.194% | −47.956% | −12.710% | −7.528% |
MACHINES | −7.281 | −79.956% | −0.615% | −8.481% | −70.860% | −20.319% | −33.191% | −17.349% |
ELECTRIC | −0.767 | −56.640% | 33.428% | −4.169% | −85.900% | 197.300% | −5.449% | −277.750% |
TRANSPORT | −303.514 | −96.016% | 4.984% | −3.668% | −97.332% | −81.202% | −3.993% | −12.136% |
MISCELLANEOUS | −12.428 | −98.410% | 6.674% | −4.302% | −100.782% | −94.116% | −2.935% | −3.731% |
UTILITIES | −37.548 | −27.356% | 11.354% | −17.566% | −21.144% | 9.046% | −33.271% | 3.082% |
Figure 3. Major drivers of induced toxic emission changes in the mining and transportation equipment sectors. Note: Each acronym represents the following: ΔScale is the change in the final demand effects, ΔSTR is the changes in the structure of industrial production, ΔTEC is the change in the toxicity intensity of emitted chemical substances, ΔEOP is the changes in end-of-pipe treatment effects, and ΔCP is the changes in cleaner production effects.
Download figure:
Standard image High-resolution image4.3. Major driver of changes in the toxicity of emissions in key industries
Table 2 shows that the toxicity induced by the mining and transportation equipment industries decreased markedly because of a reduction in the toxic emission intensity. However, the main determining factors for the observed change in toxic emission intensity differed between the two industries.
Figure 3 shows a comparison of the main driving factors affecting changes in induced toxic emissions in the mining and transportation equipment sectors. Toxic emissions in the mining sector mainly decreased because of CP factors. In the mining sector, compounds of copper, zinc and arsenic accounted 67% of the total toxic chemical release in 1998. However, the chemical release from these three metal compounds was reduced by 63% from 1998–2009, especially that from copper and arsenic compounds, which decreased by 87% and 83%, respectively. Copper and arsenic compounds rapidly decreased during this period for two main reasons.
First, copper and arsenic compounds were reduced due to scaled-down production in the US copper and gold mining sectors, respectively. The copper mining sector accounted more than 90% of the release from copper compounds in the US mining sector in 1998. Meanwhile, the production in US copper mines decreased from 1.86 million tons to 1.18 million tons from 1998–2009 (US Geological Survey 2017). Similarly, the gold mining sector accounted for more than 95% of the arsenic compound release in the US mining sector in 1998, as arsenic compounds are mainly released through the roasting of arsenious gold ores (Wang and Mulligan 2006). US gold mine production decreased from 366 tons in 1998 to 210 tons in 2009 (US Geological Survey 2017). Thus, the scaling down of copper and gold mine production contributed to the decrease in the total release of copper and arsenic compounds in the US mining sector, respectively.
Second, corporate pollution prevention activities are another contributing factor to toxic chemical release reduction in the mining sector. Especially in large-scale US mining companies, such activities were implemented to prevent environmental pollution in cyanidation practices and to enhance acid mine drainage control (Hilson and Murck 2001). Table S6 shows the corporate activities implemented for pollution prevention in the metal mining industry, as listed on the US EPA homepage (www3.epa.gov/enviro/facts/tri/p2.html). From this information, we can see that corporate pollution prevention activities also effectively contribute to reducing the sources of metal compound releases.
In the transportation equipment industry, ΔTEC strongly contributed to reducing the induced toxicity, whereas ΔCP did not have much of an effect on driving toxicity compared with that observed in the mining sector. These results imply that the transportation equipment industry improved its production processes using less toxic substances in its manufactured goods and these improvements manifested as a decrease in TEC substances, whereas the amount of chemical substances emission per industrial gross output did not change markedly. Specifically, we found that the reduction in induced toxicity in the transportation equipment industry was achieved by decreasing the release of asbestos. In the US transportation equipment sector, asbestos was responsible for 97% of the toxicity in terms of chemical releases in 1998. However, asbestos emissions were reduced from 1843 tons in 1998 to 54 tons in 2009, which decreased the share of asbestos in total chemical toxicity to 28.5% in 2009.
Asbestos was widely used in the production of high friction automotive parts (e.g. brake pads, clutch pads, etc.) (Cowan et al 2015). However, advances in materials have enabled the production of asbestos-free products (e.g. antimony compounds) (Iijima et al 2007). In the same period, the emissions of antimony compounds have increased three-fold (31 tons to 93 tons) in the transportation equipment industry. This increase implies that the transportation industry increasingly uses antimony compounds in its production processes. This substitution of chemicals and materials was the main reason for the reduction in the toxicity of chemical emissions induced by the transportation equipment sector from 1998–2009.
Here, we investigate how our estimation results are different from those in previous studies focusing on toxic chemical substance management but not on chemical toxicity. Fujii and Managi (2013) and Koh et al (2016) used decomposition analysis to analyse toxic chemical substance management in various industrial sectors in the US.
Both studies indicate that CP is a major contributor to emission intensity reduction in many industries. In this study, we reveal that TEC is a more important factor in reducing chemical toxicity in industrial sectors, especially the paper, fuel, metal, and transportation equipment sectors (see table 2). This information is useful when evaluating toxicity management activities by substituting the reduction of chemical substances or the input amount of chemical materials.
5. Uncertainty of consumption-based toxic emissions in the US
As we reported in the previous section, consumption-based toxic emissions in the US decreased by 83% from 1998–2009 (figure 2). However, an important problem is that this result may be seriously statistically biased due to data errors. To validate the statistical bias, we conducted a Monte Carlo analysis for the consumption-based accounting described in section 2.4. table S7 in the supplementary information shows the results for 95% confidence intervals of the induced toxic emissions of US industrial sectors associated with final demand by country.
Table S7 illustrates that for 1998, the consumption-based toxic emissions in the US associated with the final demand of countries (i.e. world final demand) ranged from a low of 5654 billion tons to a high of 5906 billion tons (see 'TOTAL' in 1998 of table S7 in the supplementary material). For 2009, we observed that the consumption-based toxic emissions in the US associated with the final demand of countries ranged from a low of 926 billion tons to a high of 973 billion tons (see 'TOTAL' in 2009 of table S7).
These results imply that the induced toxic emissions in the US in 1998 and 2009 are not significantly affected by data errors. Using the 95% confidence intervals for the induced emissions in 1998 and 2009, we can easily calculate the 95% confidence intervals for the change in the induced emissions from 1998–2009 as [926−5906, 973−5654]=[−4980, −4681]; therefore, we ultimately verified a sharp 82%−84% decline in consumption-based toxic emissions in the US during the study period. Similarly, we see that the toxic emissions in the US associated with the final demand of a specific country are also not significantly biased due to data errors (see table S7).
6. Conclusions
This article examined how the final demands of different countries induced changes in the toxic emissions in US industrial sectors. We focused on three factors–changes in the demand scale, production structure, and the toxic emission intensity–to identify the major drivers responsible for inducing changes in toxic chemical emissions in the US. In addition, a further decomposition approach was proposed to clarify the reason why the toxic chemical intensity changed. This approach used three factors that describe pollution abatement in industrial production processes. Our findings revealed the following three key results.
First, pollution abatement efforts by US industries have resulted in a decrease of 83% in toxic emissions induced by the US industrial sector from 1998–2009. In 2009, 87.0% of the toxic emissions in the US were induced by final US domestic demand and 13.0% were induced by foreign final demand.
Second, the largest contributors towards pollution abatement differed among industries. In particular, we found that CP methods had a significant effect on reducing toxic emissions in the mining sector from 1998–2009. Similarly, decreasing toxicity per chemical emission was a key factor in reducing the induced toxic emissions in the transportation equipment industry.
Finally, the changes in induced toxic emissions differed among importing countries and industries in exporting countries. These results imply that combinations of demand-side effects and supply-side effects are diverse. These different trends provide useful information for discussing the toxic chemical management policy that considers the product supply chain.
The integrated decomposition analysis framework developed in this study is considered useful for understanding why industrial toxic emissions have changed over time by focusing on demand-side effects and supply-side effects. In addition, the chemical toxicity accounting framework proposed in this study can be used to estimate the changes in toxic emissions induced by specific regions and countries.
To understand the change in toxic emissions in more detail, emissions discharge methods (e.g. air emissions, water discharge) are also key factors in addition to supply and demand-side effects. Future research should attempt to provide a more comprehensive evaluation of toxic emissions changes based on the type of discharge method.
Acknowledgments
We thank Sangwon Suh and Yasushi Kondo for their constructive comments about this work. We are also grateful to the editor and two anonymous referees for their helpful comments and suggestions. All errors are our own.
Conflict of interest
The authors have no conflicts of interest to declare.
Financial support
This research was funded by the Grant-in-Aid for Specially Promoted Research [26000001 B], Grant-in-Aid for Scientific Research (A) [26241031], Grant-in-Aid for Young Scientists (B) [17K12858], Grant-in-Aid for Research Activity Start-up [15H06554] from the Ministry of Education, Culture, Sports, Science and Technology. S.K. and S.M. were supported by a research grant [No. 27811] for the Tsubasa project at Kyushu University.