Abstract
Quantile Regression Neural Network (QRNN) is a hybrid method that be developed based on quantile regression (QR) that can model data with non-homogeneous variance and neural network (NN) approach that can capture nonlinear patterns in the data. One example of real data that supposedly have such characteristics is the inflow and outflow of currency, where the inflow is the amount of money flow coming from banks and the public to Bank Indonesia (BI) while outflow is the flow of money out of BI to the banks and community. The data used in this research are inflow and outflow in Yogyakarta as many as 14 notes during January 2003 until December 2016 period. This study aims to forecast inflow and outflow in Yogyakarta using QRNN method and compare the results with individual method, i.e. ARIMAX-GARCH and NN. Based on RMSE and MdAE evaluation criteria, the results show that the best model for inflow of Rp100,000, RP20,000 Rp10,000, and Rp5,000 are ARIMAX, while for the inflow Rp50,000, Rp2,000, Rp1,000, and outflow Rp100,000, Rp50,000, Rp20,000, Rp10,000, Rp5,000, Rp2,000, and Rp1,000 are QRNN. In general, the QRNN method yields better forecasting results and can capture well the effects of calendar variations on the data.
Export citation and abstract BibTeX RIS
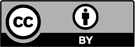
Content from this work may be used under the terms of the Creative Commons Attribution 3.0 licence. Any further distribution of this work must maintain attribution to the author(s) and the title of the work, journal citation and DOI.