Abstract
Neural network is one of flexible nonlinear models that could handle various relationship patterns on data with high accuracy. The data-driven approach is one of the advantages of neural network models in solving complex problems in forecasting. The selection of the best model becomes one of the most important problems in the application of neural network for time series forecasting, which consists of determining the input, the number of neurons in the hidden layer, the activation function, and preprocessing method. This paper focuses on the simulation study to explore how to determine the best architecture of multilayer perceptron for forecasting nonlinear seasonal time series. The data that be generated from seasonal exponential smooth transition autoregressive model are used as a case study. The results show that the inputs and the number of neurons in the hidden layer are two main factors that affect significantly the forecast accuracy. In contrary, the activation function and preprocessing method do not significantly influence the forecast accuracy.
Export citation and abstract BibTeX RIS
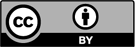
Content from this work may be used under the terms of the Creative Commons Attribution 3.0 licence. Any further distribution of this work must maintain attribution to the author(s) and the title of the work, journal citation and DOI.