Abstract
While topics on Twitter may be categorized according to their predictability and sustainability, some topics have characteristics depending on the time scale. Here we propose a good measure for the transition of sustainability, which we call the information-sharing tendency, and find that the unpredictability on Twitter is provoked by the exposure of Twitter users to external environments, e.g., mass media and other social network services. In addition, it is demonstrated that the numbers of articles and comments on on-line newspapers serve as plausible measures of exposure. From such measures of exposure, the time evolution of tweeting can be described, when the information-sharing tendency is known.
Export citation and abstract BibTeX RIS
Introduction
Twitter is an important medium in modern society, enabling to share and transfer information rapidly. Since there do not require reciprocal relationships, it is believed that intra-sharing or transferring of information on Twitter has unique features. This has raised interest in and a few works on the characteristics of Twitter [1–5]; however, various features of information sharing on Twitter still remain unclear. Specifically, whereas information flow into and its sharing on Twitter occur dynamically, a majority of the existing studies focused mostly on the analysis of correlations between various types of data on Twitter. In recent works, on the other hand, dynamic properties of Twitter such as propagation or diffusion of tweets and the propensity to tweet or retweet on the Twitter network have been studied [6–8]. In particular, it was reported that the topics on Twitter could be categorized into three kinds according to their predictability and sustainability: predictable and sustainable events, predictable but unsustainable events, and unpredictable and unsustainable events [6]. However, not all topics have such a simple property. Namely, some topics, while sustainable at one time, can become unsustainable at other times; predictable events may turn to unpredictable ones due to the occurrence of unexpected incidents related to them. For example, elections are a representative topic displaying all the characteristics associated with sustainable, unsustainable, predictable, and unpredictable events.
In this letter, we present a mathematical model for the Twitter dynamics, focusing on the possible prediction of the time evolution of tweeting. Interpreting "the retweet probability" relevant to sustainability as the tendency to share information and "the tweet probability by external causes" relevant to unpredictability as the degree to be exposed to external environments, e.g., on-line newspapers, we study how the sustainability changes on Twitter and how the exposure to on-line media affects Twitter, particularly in view of information in-flow and intra-flow. The model is then applied to the Twitter data on the 2011 election for the mayor of Seoul in Korea. This indeed demonstrates the possibility of predicting the time evolution of tweeting solely from external data.
Mathematical model
The total number Nt of tweets on Twitter on day t is given by the sum of the numbers Nn and Nr of new tweets and retweets, respectively, on the day:

In general new tweets are driven largely by mass media; retweets depend on the elapsed time as well as the value of the original tweet to retweet [6]. Denoting the motivation of a Twitter user (called a twitterian) to tweet newly as E(t) and the character function measuring variations with the day of the week as χ(t), we write the number of new tweets in the form

where N is the number of twitterians and Pn(t − τ) denotes the probability for a twitterian to tweet newly on day t on the condition that she/he was driven on day τ. Intuitively, we expect Pn(t − τ) to be very short-ranged and take Pn(t − τ) = qδt,τ + (1 − q)δt,τ+1, where δt,τ is the Kronecker delta. Accordingly, the number of new tweets on day t reads

with γ ≡ (1 − q)/q.
The tendency for twitterians to tweet on weekdays is different from that at weekends: While the tendencies on five weekdays are essentially the same, the tendencies on weekends or holidays are reduced to half of those on weekdays [6]. The character function is thus given by

Note that χ(t) accounts for the influence of the day, not of driving to tweet but of actual tweet. Therefore in eq. (2), it appears in the form χ(t) rather than χ(τ).
Among the total tweets on day τ, some will be retweeted at some time or later whereas others never will. With r(τ) denoting the ratio of the former to the total tweets on day τ, the number of retweets on day t obtains the form

where Pr(t − τ) represents the probability for a tweet, sent on day τ, to be retweeted on day t. It is expected that Pr(t − τ) is not so short-ranged as Pn(t − τ). In a recent work, it was reported that 75% of the retweeted tweets are retweeted on the very day of receiving the tweets. Beyond that day, the cumulative distribution of the required time for a tweet to be retweeted follows a linear form in the semi-log plot [1]. This leads to the relation

Substituting eqs. (2), (5), and (6) into eq. (1), we have the total number of tweets on day t:
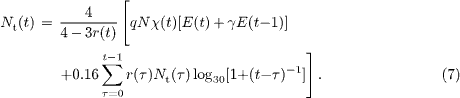
Since r(t) corresponds to the fraction of tweets on day t to be retweeted at some time or later, it measures how much the tweets on day t are worth retweeting, i.e., the value to share or transfer the information. For this reason, we call r the information-sharing tendency. From eqs. (5) and (6), it obtains the form

where fr(t) ≡ Nr(t)/Nt(t). Therefore, we may extract r(t) from the data on the total number Nt(τ) of tweets for 0 ⩽ τ ⩽ t and the number Nr(t) of rewteets. Note that the cumulative distribution corresponding to eq. (6) was obtained for 10 trending keywords [1] and accordingly, the validity of eq. (6) is not guaranteed for small r(t). In particular, when a keyword does not interest twitterians, namely, r(t) is vanishingly small, eq. (8) may yield a negative value of r(t). Accordingly, to remedy this artifact, we set r(t) = 0 whenever we meet a negative value of r(t).
Twitter data and information-sharing tendency
We now apply the model to the Twitter data on the Seoul mayoral election held on 26 October, 2011, in Korea. There were three candidates for the Mayor of Seoul: "PARK Won-Soon", "NA Kyung-won", and "BAE Il-do". In the election, where the total number of votes reached 4066557, "PARK Won-Soon" got 2158476 votes (53.08%), "NA Kyung-won" 1867880 votes (45.93%), and "BAE Il-do" 15408 votes (0.39%). In practice, the election was a confrontation of two candidates, "PARK Won-Soon" and "NA Kyung-won", and we thus focus only on the data for the two candidates. Actual data on the numbers of total tweets and retweets on Twitter and those of articles and comments on the on-line newspapers were obtained from keyword searches for the names "PARK Won-Soon" and "NA Kyung-won" in Korean. Since each retweet has a prefix "RT @", we counted the number of tweets that have the string "RT @" to obtain the number of retweets. From 1 October to 31 October in the year 2011, there occurred 680349 tweets and 523383 retweets for "PARK Won-Soon" while 692497 tweets and 556085 retweets for "NA Kyung-won". In obtaining the numbers of articles and corresponding comments appearing in mass media, we chose two on-line newspapers: "Chosunilbo" which is a conservative Korean press and "Hankyoreh" which is a liberal Korean press. In this manner we keep a balanced position, and present only the sum of the numbers of articles or comments over two on-line newspapers, rather than over each one. From 1 October to 31 October in the year 2011, there appeared 1069 articles and 32172 comments for "PARK Won-Soon" and 716 articles and 20354 comments for "NA Kyung-won".
Since the election day, tc = 26 October, is known in advance, it is expected intuitively that the topics about the election belong to predictable and sustainable events. However, there occur day by day some incidents and events related to the candidates, which should be considered as unpredictable and unsustainable events on short-time scales. Figure 1 shows temporal changes of the numbers of total tweets, new tweets, and retweets for each candidate. Note that there are a few transient increases or decreases in the number of total tweets. It is, however, not conceivable that these variations actually reflect the changes of the degrees of twitterians' concern with the election or with the candidates.
Fig. 1: (Colour on-line) Daily changes of the numbers of new tweets (triangles), retweets (squares) and total tweets (circles) searched by keywords "PARK Won-Soon" (a) and "NA Kyung-won" (b), from 1 October to 31 October in 2011. The vertical dotted lines indicate the election day, tc = 26 October, 2011; lines connecting data points are merely guides to the eye. The scales on the vertical axis are given in units of 10000.
Download figure:
Standard imageFigure 2 reveals that the information-sharing tendency r was maintained essentially constant until the election day while the number of retweets varied largely. In particular, r remained unchanged on 20 October, 2011, despite that, there were a huge number of retweets for the keyword "NA Kyung-won" on that day. From this, it is inferred that the change of the number of retweets is not provoked by the change in the propensity of twitterians to sharing/transferring information but induced by the change in the information flow into Twitter. Namely, the number of retweets reflects the amount of information flowing into Twitter, e.g., the number of articles exposed to twitterians on the Internet, the effects of mass media, and so on. On the other hand, it is interesting that the tendency r(t) reduced abruptly right after the election day (t > tc). Namely, twitterians no more tend to share or transfer information after the election, and the keyword is deprived of its sustainability. This discloses a transition at which a sustainable event, characterized by a strong tendency to share information, becomes an unsustainable one, with a weak tendency. It is also shown in fig. 2 that the fraction fr(t) of the number of retweets to that of total tweets on day t hardly changed not only until the election day but also even after it, in sharp contrast with r(t). Accordingly, unlike r(t), fr(t) is not proper to measure the propensity of twitterians to sharing/transferring information.
Fig. 2: (Colour on-line) Daily changes of the information-sharing tendency r (triangles), the fraction fr of the number of retweets to total tweets (squares), and the number Nr of retweets (circles) for keywords "PARK Won-Soon" (a) and "NA Kyung-won" (b). The vertical dotted lines indicate the election day tc; lines connecting data points are merely guides to the eye. The scales on the right vertical axis are given in units of 10000.
Download figure:
Standard imageFurther, it is of interest that r(t) for "PARK Won-Soon" grew again on 31 October, whereas r(t) for "NA Kyung-won" remained to be zero at that time. This indicates that while the keyword "NA Kyung-won" just as an ordinary citizen did not interest twitterians at all, the keyword "PARK Won-Soon" as the mayor-elected did.
External data and time evolution of tweeting
It is likely that articles on newspapers serve as a good source for information on Twitter: The higher the number of articles to be exposed to twitterians, the larger the amount of information to flow into Twitter. Accordingly, we presume that the number of articles is a reasonable candidate for the motivation E(t) to tweet newly. In addition, the number of people who comment on the articles perhaps serves as a measure for the motivation to tweet newly, and therefore the number of comments provides another candidate for E(t). Since the information-sharing tendency r(t) is invariant under the transformation ,
,
and
, eq. (7) is also invariant under the transformation, giving freedom of the overall normalization. Here we choose the normalization of E(t), which is appropriate for the sum of Nt(t) during October, namely, which leads to the sum of simulation data for Nt(t) equal to the sum of the actual data. The resulting normalization constants are listed in table 1. Figure 3 shows that the general trends of temporal variations in the numbers of articles and comments, denoted by E, indeed remained roughly parallel with respect to those in the numbers of new tweets, Nn. In contrast to r(t) which measures the information-sharing tendency, E(t) reflects the change by unpredictable incidents and events.
Fig. 3: (Colour on-line) Numbers E(t) of articles (triangles) and comments (squares) with appropriate normalization, together with the numbers Nn(t) of new tweets (circles) for keywords "PARK Won-Soon" (a) and "NA Kyung-won" (b). The vertical dotted lines indicate the election day; lines connecting data points are merely guides to the eye. The scales on the left and right vertical axes are given in units of 0.001 and 10000, respectively.
Download figure:
Standard imageTable 1:. Normalization constant for motivation E(t).
PARK Won-Soon | NA Kyung-won | |
---|---|---|
Articles | 3.37×10−5 | 5.16×10−5 |
Comments | 1.11×10−6 | 1.87×10−6 |
In order to probe whether the numbers of articles and comments play the role of E(t), we substitute each of them (with appropriate normalization) for E(t) in eq. (7), and compute the number of total tweets Nt(t), which are plotted in fig. 4. In obtaining these model results, we have used the information-sharing tendency r(τ) for 0 ⩽ τ ⩽ t, extracted from the real data through eq. (8). Figure 4, which displays the model results in comparison with the real data, confirms that both the numbers (of articles and of comments) serve as good measures for E(t). This has significant implications and prospects with regard to the possibility of prediction: When a time series of the information-sharing tendency r(t) is given, we can predict the corresponding time series of the numbers of tweets and retweets from the external data. In principle, to obtain the information-sharing tendency r(t) in eq. (8), we need to know the numbers of tweets and retweets in advance. Note, however, that the information-sharing tendency reflects the intra-structure of Twitter and is expected not to vary much for similar topics. Accordingly, for r(t), we may use one obtained from past data for similar topics or one obtained possibly by intuition.
Fig. 4: (Colour on-line) Numbers Nt(t) of total tweets, obtained from the model with the numbers of articles (triangles) and of comments (squares) chosen as E(t), in comparison with real data (circles) for keywords "PARK Won-Soon" (a) and "NA Kyung-won" (b). In obtaining the model results, we have used r(t) in fig. 2 and parameters q = 0.9 (and thus γ = 1/9) and N = 4000000, which is roughly the number of the Korean users of Twitter. The vertical dotted lines indicate the election day; lines connecting data points are merely guides to the eye. The scales on the vertical axis are given in units of 10000.
Download figure:
Standard imageAs an instance, we suppose that r(t) takes the simple form

where
rb and ra are constants and η(t)'s are independent random numbers distributed uniformly in the range , i.e.,
and
. In numerical simulations, taking the standard deviation of the actual data for r(t) as σ, we control directly the standard deviation of the distribution rather than its range. Specifically, we use the parameters as follows: While ra is taken to be zero for both "PARK Won-Soon" and "NA Kyung-won", the average value of r(t) over 26 days until the election day (t ⩽ tc) is assigned to rb, i.e.,
rPARKb = 0.911 and rNAb = 0.952. In a similar manner, σ is taken to be the mean standard deviation of r(t) during 26 days (t ⩽ tc), i.e., σPARK = 0.065 and σNA = 0.056. The total numbers of tweets, obtained in this manner from the model, are plotted in fig. 5, where real data are also shown for comparison. It is observed that the overall dynamic behavior, predicted by the model, agrees reasonably well with the behavior of the real data.
Fig. 5: (Colour on-line) Numbers Nt(t) of total tweets, obtained from the model with the numbers of articles (triangles) and of comments (squares) chosen as E(t), in comparison with real data (circles) for keywords "PARK Won-Soon" (a) and "NA Kyung-won" (b). In obtaining the model results, we have used the simplified form of r(t) in eq. (9) and parameters q = 0.9, N = 4000000, ra = 0, rPARKb = 0.911, σPARK = 0.065, rNAb = 0.952, and σNA = 0.056. Data have been averaged over 10000 independent runs and error bars have been estimated by the standard deviations. The vertical dotted lines indicate the election day; lines connecting data points are merely guides to the eye. The scales on the vertical axis are given in units of 10000.
Download figure:
Standard imageSummary
In summary, we have presented a mathematical model for the Twitter dynamics, applied to the Twitter data on the Seoul mayoral election. Revealed is the occurrence of a transition on the election day, at which a sustainable event became unsustainable. This transition, characterized by a sharp drop in the information-sharing tendency, is not captured by the simple fraction of the number of retweets to that of total tweets. In addition, we have carried out simulations, to probe the time evolution of the number of total tweets, and with the help of the information-sharing tendency extracted from the real data, demonstrated that the number of articles or comments may be adopted as the motivation for tweeting. It has also been found that unpredictable and unsustainable events occurring incidentally day by day hardly affect the information-sharing tendency, merely changing the twitterians' motivation to tweet newly. In other words, they do not affect the degree of intra-sharing or transferring of information on Twitter but disturb the amount of information flow into Twitter. Finally, with a simplified form of the information-sharing tendency, the model can predict the dynamic behavior of Twitter solely from the external data, which has many potential applications. More precise modeling of the information-sharing tendency, including the probability distribution of the requirement time for a tweet about a non-trending keyword to be retweeted, and corresponding applications are left for further study.
Acknowledgments
This work was supported by the National Research Foundation of Korea through the Basic Science Research Program (Grant Nos. 2009-0080791 and 2011-0012331).