Abstract
The rising demands of more reliable and stable electrical systems attach importance to accurate Remaining Useful Life (RUL) prediction of the lithium-ion batteries. As artificial intelligence and machine learning techniques advance, data-driven methods especially deep learning algorithms have become the rising star in RUL prediction. Recurrent Neural Networks (RNNs) and their variants such as Long Short Term Memory have proven effectiveness in various sequential tasks. However, due to its iterative nature along the time axis, RNNs take much time for information to flow through the network for prediction. Inspired by recent advance brought by Transformer in sequence transduction tasks, we proposed the attention mechanism based Convolutional Neural Network (CNN) with positional encoding to tackle this problem. The attention mechanism enables the network to focus on specific parts of sequences and positional encoding injects position information while utilizing the parallelization merits of CNN on GPUs. Empirical experiments show that the proposed approach is both time effective and accurate in battery RUL prediction.
Export citation and abstract BibTeX RIS
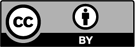
Content from this work may be used under the terms of the Creative Commons Attribution 3.0 licence. Any further distribution of this work must maintain attribution to the author(s) and the title of the work, journal citation and DOI.