Abstract
Answer selection is the most important module of question answering system. Mining the semantic relevance between questions and answers is the key point of answer selection. However, the answer texts often contain some colloquial expressions and redundant information in some fields. In these cases, some traditional methods of mining text features are complex and the performance improvement is limited. In this paper we investigate using deep learning to enhance the computability of semantics and eliminate the semantic gap for the answer selection module of non-factual question answering system. By integrating different networks to mine the association semantic between questions and answers, a novel multi-layer answer selection model is proposed. The shallow semantic relevance between questions and answers is mined by adding the attention mechanism to CNN, and then the features are input into Bi-LSTM model for further mining to obtain more efficient semantic representation. The experimental results show that compared with other models, our method can achieve the better accuracy in the task of answer selection.
Export citation and abstract BibTeX RIS
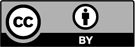
Content from this work may be used under the terms of the Creative Commons Attribution 3.0 licence. Any further distribution of this work must maintain attribution to the author(s) and the title of the work, journal citation and DOI.