Abstract
In this paper, we consider the hybridization problem of the evolutionary-inspired Fish School Search algorithm. The algorithm shows accurate results on solving multi-extremal optimization problems, outperforms most other swarm intelligence algorithms and is computationally inexpensive. But, the accuracy of the algorithm highly depends on the defined iteration count to compute. The accuracy increases with the increase of iteration count, but this also affects computation time required to find the suboptimal solution. In this paper, we propose two hybrid approaches which intend to accelerate the Fish School Search algorithm using either gradient descent or Newton's algorithm. We provide the comparative analysis of the original algorithm and the two hybrid algorithms applied to the Styblinsky-Tang, Eggholder and other test functions for optimization, including multi-extremal and multi-dimensional ones. The obtained results confirm that the proposed hybrid algorithms are more effective, compared to the original population-based algorithm. The results demonstrate the strong advantage of the hybrid algorithm, where the gradient descent is used as its part. Additionally, we provide the solution of the XOR problem using a perceptron with one hidden layer, where the most effective hybrid approach is used to train the network. We visualize the loss function surface near the global extreme point to show the effectiveness of the proposed hybrid algorithm.
Export citation and abstract BibTeX RIS
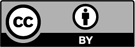
Content from this work may be used under the terms of the Creative Commons Attribution 3.0 licence. Any further distribution of this work must maintain attribution to the author(s) and the title of the work, journal citation and DOI.