Abstract
The usage of the face recognition system is various, one of which is to identify missing people. Cases of missing persons can usually happen to people who do not carry an identity card, such as people with mental disorders. Identity cards have some information, namely name, address, date of birth, and a photo ID of a face. This information has stored at the civil registry office, which is an Indonesian government agency. Therefore to solve this problem, we use a photo ID as a dataset to identify someone's identity. Photo ID as a dataset has age progressing factors in facial recognition systems. Several previous studies have discussed age progressing factors. Previous research used MORPH, CACD, and FGNET datasets. The dataset has several photos of faces consisting of various age levels for the same subject, but it is different from the photo ID that we use. For the training data process, we only use one data, namely a photo ID used to recognize faces at this point. Therefore we use the pre-trained VGGFace2 model, and then, fine-tuning the data during the training process, we use AM-Softmax loss as a loss function. Then the classification is done using SVM. The method we use than compared with the Moustafa method, which also uses a pre-trained model. The results we obtained have a better accuracy performance of 0.9351 compared to the Moustafa method of 0.733
Export citation and abstract BibTeX RIS
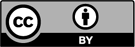
Content from this work may be used under the terms of the Creative Commons Attribution 3.0 licence. Any further distribution of this work must maintain attribution to the author(s) and the title of the work, journal citation and DOI.