Abstract
Diabetic retinopathy is a complication of diabetes that targets the eyes by damaging the retinal blood vessels. Initially it is asymptomatic or causes fluctuating vision problems. As it becomes severe, it affects both the eyes and eventually causes partial or complete vision loss. Primarily occurs when the blood sugar level is unmanageable. Therefore, the person with diabetes mellitus is always at a high risk of acquiring this disease. The early detection can deter the contingency of complete and permanent blindness. Thus, requires an efficient screening system. The present work considers a deep learning methodology specifically a Densely Connected Convolutional Network DenseNet-169, which is applied for the early detection of diabetic retinopathy. It classifies the fundus images based on its severity levels as No DR, Mild, Moderate, Severe and Proliferative DR. The datasets that are taken into consideration are Diabetic Retinopathy Detection 2015 and Aptos 2019 Blindness Detection which are both obtained from Kaggle. The proposed method is accomplished through various steps: Data Collection, Preprocessing, Augmentation and modelling. Our proposed model achieved 90% of accuracy. The Regression model was also employed, manifested up an accuracy of 78%. The main aim of this work is to develop a robust system for detecting DR automatically.
Export citation and abstract BibTeX RIS
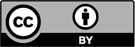
Content from this work may be used under the terms of the Creative Commons Attribution 3.0 licence. Any further distribution of this work must maintain attribution to the author(s) and the title of the work, journal citation and DOI.