Abstract
Future climate projections are valuable datasets to investigate the impacts of future climate changes on natural disasters such as intense precipitation, severe flood, and drought. However, they are too coarse depending on the purpose, and downscaling is required in such a case. There is nowadays a downscaling technique using deep learning such as CNN. Atmospheric information can be used as an input for precipitation downscaling by means of Convolutional Neural Network (CNN). For such precipitation downscaling, the spatial and temporal resolution of the atmospheric information may be important. This study obtained atmospheric information from a coarser reanalysis dataset and a finer reanalysis dataset as input for precipitation downscaling. As a coarser reanalysis dataset, ERA-Interim was selected. As a finer reanalysis dataset, ERA5 was utilized. Then, this study investigated the effect of spatial and temporal resolution of input data on the estimation accuracy of precipitation downscaling by CNN. For simplification, daily average precipitation at a watershed was used as the target data. The results show advantage of the use of a higher resolution as input can improve the model accuracy.
Export citation and abstract BibTeX RIS
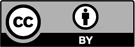
Content from this work may be used under the terms of the Creative Commons Attribution 3.0 licence. Any further distribution of this work must maintain attribution to the author(s) and the title of the work, journal citation and DOI.