Abstract
The CO2 water-alternating-gas flooding (CO2-WAG) is a key technology to improve the oil recovery of low permeability reservoirs. The effect of CO2 flooding to enhance the oil recovery is affected by geological conditions and production systems. The effect of CO2 flooding parameters on the enhanced recovery factor should be clarified to optimize the production system. In this paper, the machine learning algorithms are used to carry out the study and establish a set of procedures for optimizing CO2 flooding parameters based on the artificial neural network (ANN) and the particle swarm optimization (PSO) algorithm. Firstly, large amounts of basic data are generated by the Monte Carlo sampling method. Then, the recovery factor by the water flooding and the CO2-WAG and the enhanced recovery factor by CO2-WAG in different models are calculated in the reservoir numerical simulator. Moreover, the machine learning method is used to establish a neural network model, and analysis of the sensitivity of parameters of the enhanced oil recovery (EOR) is carried out by combining with the Sobol method. Finally, the neural network model and the particle swarm algorithm are combined to optimize the parameters of CO2-WAG flooding. The results show that the established model has a good prediction accuracy (97.6%), thus it could be used to predict the enhanced recovery factor by CO2-WAG, and it is applicable in potential evaluation of enhancing the oil recovery and optimization for parameters in the CO2-WAG well group.
Export citation and abstract BibTeX RIS
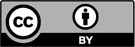
Content from this work may be used under the terms of the Creative Commons Attribution 3.0 licence. Any further distribution of this work must maintain attribution to the author(s) and the title of the work, journal citation and DOI.