Abstract
Classification and identification of yellowing, spotted and normal green leaves were performed by using hyperspectral imaging technology. Firstly, three kinds of support vector machine (SVM) classification models were established under different preprocessing methods. The optimal preprocessing method (normalized+ SG+ first derivative) was obtained by using the pixel as the data set input and the recognition rate of the classification reached 97.4%. However, the amount of data information is large and the detection time is relatively long. In order to further optimize the spectral characteristics and improve the detection efficiency, principal component analysis (PCA) was used for feature selection and feature extraction under this optimal pretreatment method. The experiment shows that the feature extraction method selects the optimal principal component number of 7 in the dimension reduction experiment with the dimension ranging from 3 to 15 and the recognition rate is 97.3% after SVM classification, which is 4.8 percentage points higher than the feature selection method. The method of feature selection speeds up by 22.57s, which is faster than the optimal preprocessing method by 104.07s, which can greatly improve the recognition and detection speed while ensuring the accuracy. The results show that the hyperspectral image technology combined with the support vector machine classification model can achieve the non-destructive rapid detection of green leaf defects under the normalization + SG + first derivative preprocessing method and feature extraction.
Export citation and abstract BibTeX RIS
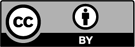
Content from this work may be used under the terms of the Creative Commons Attribution 3.0 licence. Any further distribution of this work must maintain attribution to the author(s) and the title of the work, journal citation and DOI.