Abstract
Naïve Bayes Imputation (NBI) is used to fill in missing values by replacing the attribute information according to the probability estimate. The NBI process divides the whole data into two sub-sets is the complete data and data containing missing data. Complete data is used for the imputation process at the lost value. The process is repeated for each missing attribute to generate complete data for classification. This research applies NBI for imputation and preprocessing as preparation of classification process. The trial of this study used NBI for imputation compared to using the mean and mode to predict the missing data. The data used for imputation is full train of complete data as a whole to predict the missing value so as to represent the entire data. The results of this study prove that imputation with NBI produces the right imputation with higher accuracy than other imputations. NBI with single imputation and multiple imputation results in better performance because of the right features. This study aims to calculate the effect of missing values on Naïve Bayes Imputation Algorithm is based on a probalistic model using mixed data. Empirically shows that the interaction between several methods of imputation and supervised classification results in differences in the performance of classification for the same imputation method.
Export citation and abstract BibTeX RIS
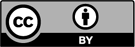
Content from this work may be used under the terms of the Creative Commons Attribution 3.0 licence. Any further distribution of this work must maintain attribution to the author(s) and the title of the work, journal citation and DOI.