Abstract
In the Japanese construction industry, work environment and productivity data of construction projects are collected and analyzed by on-site engineers as individual data based on attendance and performance records. Therefore, productivity data are not uniformly collected and cannot be considered as highly reliable. Therefore, in this study, a prototype of a system is developed that automatically collects data regarding the workers' activities using sensors and analyzes the working environment and productivity of construction projects. Although image analysis is typically employed for capturing workers' activities, it is extremely costly and time-consuming for collecting and discriminating data. In addition, it is not practical because it requires adjusting the system to suit the changing environment of the construction site each time. Accordingly, we have conducted motion-detection using accelerometers, gyro-sensors, and GPS that can be attached to individual workers. We have constructed a hierarchical system that combines a phase to detect large acceleration changes that trigger motion, a phase to discriminate periodic motion, and a phase to classify motion by machine learning. The system classified motions into three productivity types: "direct work," "support," and "delay." As a result of applying this system to the sensing data of workers performing road pavement construction-related works, the system discriminated with an overall accuracy rate of 42%. Furthermore, because of the wide range of behaviors that correspond to each productivity behavior category, a large amount of data must be read from the site adopting the system for obtaining training data to perform final behavior classification by machine learning based on the sensing data. Therefore, a system relying on machine learning may not be able to achieve a higher accuracy; thus, in the near future, we aim to automatically discriminate the nine categories of productivity behaviors shown in previous studies.
Export citation and abstract BibTeX RIS
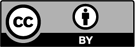
Content from this work may be used under the terms of the Creative Commons Attribution 3.0 licence. Any further distribution of this work must maintain attribution to the author(s) and the title of the work, journal citation and DOI.