Abstract
Cropland expansion and agriculture intensification have been the primary methods to increase food production since the 19th century. The resulting landscape simplification, however, can impede long-term agricultural crop productivity. This paper examines the role of landscape diversification on resilience of corn, soy, and winter wheat production, in terms of crop yield and yield variability, in the state of Kansas. The study uses panel data analysis with county fixed effects and time trends to estimate the relationship between landscape diversity and crop production resilience. Results show that diversity has a significant positive effect on yields after controlling for weather, irrigation, and chemical inputs. We find that the yields of winter wheat increase, on average, by 28% at high levels of landscape diversity, while corn and soy yields increase 7% and 5%, respectively. In addition, we find that increases in landscape diversity are significantly associated with reduced yield variability for winter wheat and corn, and that landscape diversity mitigates the effect of extreme weather conditions on yield. Study findings suggest that within a single, relatively low diversity state, increasing landscape diversity is positively associated with crop production resilience. Future extreme climate conditions may reduce crop yields and yield stability, requiring appropriate policies to ensure food security. Our findings suggest that landscape diversification may be an important tool within a portfolio of approaches to increase crop resilience under highly variable weather conditions.
Export citation and abstract BibTeX RIS

Original content from this work may be used under the terms of the Creative Commons Attribution 4.0 license. Any further distribution of this work must maintain attribution to the author(s) and the title of the work, journal citation and DOI.
1. Introduction
Increasing food production to feed a growing population has been a major concern of agriculture in the last few decades. Efforts to boost production have led to practices of agricultural intensification and landscape simplification. In the US, highly specialized cash crop farming became prominent in the 1950s, resulting in higher yields, but also in substantial changes to landscapes (Hart 1986, Karlen et al 1994). Today, half of all cultivated land worldwide is devoted to growing corn, wheat, rice and other grains (FAO 2020). Landscape simplification, however, can impede crop productivity growth (Burchfield et al 2019a, Renard and Tilman 2019), as land-use intensification is associated with degradation of essential ecosystem functions that allow biological productivity, organizational integrity, and perpetuation of agricultural ecosystems (Swift et al 2004). As a result, modern farming systems optimized to boost crop productivity by agricultural intensification and simplification may hinder long-term crop performance.
Crop diversification is an approach to addressing long-term diminished crop performance. Field-scale studies and experiments (Di Falco and Perrings 2005, Smith et al 2008, Abson et al 2013, Weigel et al 2018, Beillouin et al 2019) have shown that diversification practices result in higher yields than monoculture because biodiversity helps preserve essential ecosystem functions, making food and farming systems more resilient to climate change and enhancing agroecosystem diversity. This gain in resilience may compensate for the negative effects of high-intensity management (Tscharntke et al 2005, Aguilar et al 2015, Gaudin et al 2015).
Yield resilience is attracting widespread interest due to the disruptions that climate change and other environmental and non-environmental perturbations, such as flooding and geopolitical conflict, create in food production (Bullock et al 2017, Seekell et al 2017). Resilience in food production is defined as the capacity to maintain agricultural production despite resource variability and climate change, or the food system's ability to adapt and respond to such perturbations (Altieri et al 2015, Bullock et al 2017, Li et al 2019). Specific perturbations to crop yield include weather events, pest outbreaks, and diseases (Redhead et al 2020). Crop-yield resilience has been measured using quantitative outcomes such as yield stability, yield resistance to stressful growing conditions, recovery time following a shock, and maximum yield potential (Nalley and Barkley 2010, Gaudin et al 2015, Li et al 2019, Renwick et al 2021).
The yield effect of landscape diversification has been extensively explored in the literature, but most research has used field-scale yield data with measures of localized (∼1 km radius or less) land-cover diversity. However, how field-scale results scale up to produce aggregate effects on crop production is not well understood. Theory suggests that land-use diversity may produce heterogeneous services and disservices to crop production across landscapes to produce aggregate effects of land-use diversity that are more than the sum of field-scale expectations (Swift et al 2004, Letourneau et al 2011, Dively et al 2018). Recent landscape-scale studies (Burchfield et al 2019b, Galpern et al 2020, Nelson and Burchfield 2021) that use aggregate yields across areas spanning 10s to 100s of kilometers across, and measures of land-cover diversity across these areas, appear to confirm that increasing landscape diversity is associated with improved crop production even across larger landscape scales. Burchfield et al (2019b) investigate the relationship between crop diversification and yield for county landscapes and found that areas with higher diversity tend to have higher yields. Although their approach implicitly accounted for the effect of ecological processes due to interactions between land-use, the biophysical environment, and human action, their study did not explicitly account for chemical inputs or irrigation volumes and only examined agricultural outcomes in terms of average county yields. Similarly, Galpern et al (2020) find that higher levels of landscape diversity are associated with higher levels of crop production; however they do not explicitly control for environmental conditions or inputs and examine only the effect of landscape complexity on yields.
While yields are clearly an important metric for understanding agricultural system resilience, examination of additional measures of agricultural system success and persistence are necessary to improve understanding of the connection between aggregate landscape diversity and resilience of agricultural crop production. Similarly, given the undeniable influence of inputs that strongly influence crop production, such as irrigation, fertilizers, and pesticides, models that account for these factors are needed to verify the robustness of findings that landscape diversity has a beneficial influence on crop production at landscape scales and confirm that these findings are not a product of spurious correlation with previously omitted input factors.
At the landscape scale, anecdotal evidence suggests that landscape diversity may enhance crop production resilience (Bullock et al 2017), but the supporting literature is undeveloped. Birthal and Hazrana (2019) and Redhead et al (2020) are among the handful of studies addressing this relationship. For instance, using a panel of district-level data, Birthal and Hazrana (2019) show that crop diversification mitigates harmful effects of climate shocks on agricultural productivity (in terms of value of crop output per unit of land) in India. Specifically, districts with more diverse crop portfolios displayed reduced sensitivity to rainfall deficits when compared with districts with less diverse crop portfolios (Birthal and Hazrana 2019). While this study shows that a diverse crop portfolio can help increase resilience of entire farming operations by spreading risk across several crops, it does not determine if diversity alters the resilience of a single crop. Relatedly, Redhead et al (2020) found that stability of English wheat yields and resistance of yields to a heavy rainfall event were both positively associated with proximity of fields to areas classified as semi-natural habitat. This study suggests that diversity can increase the resilience of a single crop, presumably through provision of ecosystem services. However, the study examines diversity of land-cover in terms of only arable land, semi-natural habitat, and semi-natural grassland so is unable to assess if adding more types of crops on arable land may influence resilience. Overall, additional studies are needed to determine how yield resilience varies across contexts and to determine the robustness of these findings across methodological approaches.
This study examines the relationships between diverse agricultural landscapes and crop production resilience in Kansas. Specifically, we address the following research questions:
- (a)Is higher landscape diversity associated with higher yields within a region with a relatively consistent cropping system structure?
- (b)Is landscape diversity associated with short-term (sub-decadal) yield variability/stability?
- (c)Does landscape diversity moderate the effects of weather conditions on crop yields and yield variability/stability?
We understand crop production resilience to refer to the ability of agricultural systems to maintain high crop yields and reduced yield variability in the face of weather conditions not conducive to crop growth (henceforth called 'adverse weather conditions'). While there are many dimensions to diversity, we focus on the spatial diversity of county landscapes in terms of the number of land-cover types (including both crop and non-crop land-covers) and their relative proportions in a county each year. This diversity of land-cover composition may influence, for example, beneficial insect habitat, spread of crop diseases and pests, or impacts of herbicide drift. Landscape compositional diversity is intimately tied with the complexity of landscape configuration, or the spatial arrangement of land-covers, and prior work has suggested that landscape configuration is also associated with crop yields and ecosystem services that support crop yields (Hass et al 2018, Martin et al 2019). However, the correlations between landscape diversity and landscape configuration are not one-to-one (for example, a low diversity landscape with only two land-cover types may have irregular land-cover patch shapes that wind and meander to create high configurational complexity) and prior work at a county landscape scale has shown that associations between landscape structure and crop yields are stronger for measures of landscape diversity than measures of landscape configuration (Nelson and Burchfield 2021).
By exploiting county-level data from Kansas, this paper makes three contributions. First, we examine crop production resilience at the state scale to remedy the primary shortfall of related national-scale studies (Burchfield et al 2019b, Nelson and Burchfield 2021) which have not adequately considered localized context that could significantly shape agricultural practices and resulting ecological processes. In the United States, farming decisions such as land and water use, crop mix, and input usage depend on local policies and natural resources that may vary widely between states. Second, we account for the use of agronomic inputs (i.e. irrigation water, fertilizer, and pesticides), which are primary determinants of crop yields, using county-level irrigation water use and expenses on fertilizer and pesticides (herbicides and insecticides). Third, this study examines crop production resilience in terms of two outcomes, average county-annual yields and yield variability, and explicitly examines how landscape diversity alters the response of these two outcomes to weather conditions (resistance). Improving our understanding of the benefits of landscape diversity on crop yields, yield variability, and resistance of crop production to weather impacts is essential to motivate and assist farmers in diversifying agricultural production (Chavas and Kim 2010, Bowman and Zilberman 2013). In addition, grasping the impacts of diversification at a broad landscape scale may provide relevant information for regional agricultural planning.
2. Method
2.1. Data
Annual county-level data on winter wheat, corn, and soy crop yields, land-cover, and weather for Kansas were obtained from Nelson and Burchfield (2021) for the period of 2008–2018. The average county area is approximately 2029 square kilometers suggesting county landscapes in Kansas are generally in the range of 10s of kilometers across. The extracted weather variables include growing degree days (GDDs), stress degree days (SDDs), and total seasonal precipitation (TP) for each county-crop-year. GDDs, SDDs, and TP are crop-specific measures during the growing season, where GDDs are the accumulated daily degrees (in Celsius) within a temperature range in which a crop's growth rate increases (Miller et al 2001), SDDs are the total accumulated degrees Celsius above a crop's maximum GDD temperature threshold (Lobell et al 2013, Roberts et al 2017), and TP is the sum of precipitation (in millimeters) during a crop's growing season. Growing season duration was defined using the spatially explicit, crop-specific planting and harvesting dates provided by Sacks et al (2010). These measures were calculated using county average daily precipitation and temperature computed from daily gridded (4 km) weather estimates from the Parameter-Elevation Regressions on Independent Slope Model (PRISM Climate Group 2004). The crop-specific (but not cultivar specific) weather metrics vary across both space (counties) and time although variations are stronger across years than across space (figure S1).
Our measure of landscape diversity, the Simpson Diversity Index (SIDI), was extracted from the same dataset for each county-year and has been used to examine relationships between landscape diversity and crop yields in prior national studies (Birthal and Hazrana 2019, Burchfield et al 2019b, Nelson and Burchfield 2021). SIDI is a metric of both the abundance and evenness of land-cover categories that measures the probability that two randomly selected pixels belong to different land-cover categories (McGarigal 1995) and was computed using 30 m spatial resolution annual land-cover information from the USDA Cropland Data Layer (USDA NASS CDL 2018) As a measure of spatial diversity of land-cover composition SIDI does not directly account for temporal diversity resulting from crop rotations or cover cropping, for within-field diversity due to intercropping, or for genetic diversity. More information on SIDI and its use in this study can be found in the supporting information.
The Great Plains region of the United States records relatively high rates of potential evapotranspiration, or potential water loss, due to the semi-arid nature of the region (Chen et al 2019). As a result, irrigation is a common practice for most crops, with corn and soybeans being the main irrigated crops in the region. To control for the heterogeneous impact of irrigation on Kansas crop yields, this study included total annual water volume used for irrigation (henceforth 'irrigation') by county in acre-feet (KSDA, 2020). Irrigation water use data is reported by individual irrigators and reviewed by the Kansas Department of Agriculture. Note that this data does not distinguish between irrigation water applied to different crops, providing a simple measure of total irrigation water used in each county in each year. As irrigation volumes vary by orders of magnitude across counties (figure S2) additional models in which observations with irrigation values in excess of the 95th percentile were dropped and models employing a log transformation of irrigation values were run to test robustness of model findings to bias from data skewness.
A myriad of additional factors may affect crop production as agriculture is an input intensive sector (McArthur and McCord 2017). In recent years farmers have relied heavily on fertilizer and pesticides use to increase yields (Davis et al 2012). Data from the Kansas Farm Management Association (KFMA) was used to control for farm fertilizer expenses and combined herbicide and insecticide expenses (henceforth 'pesticide') (KFMA 2020). Expenses in each year were adjusted using the national average of the producer price index for pesticides or fertilizers published by the U.S. Bureau of Labor Statistics (n.d.) using 2008 as the index base year. Irrigation, fertilizer expenses, and pesticide expenses were normalized using total acres in each county, and each predictor variable was standardized to zero mean and standard deviation of one (z-scores) prior to modeling to compare the effects in each predictor on yield and yield variability.
2.2. Econometric model specification
Our models use Kansas county-level panel data to test whether landscape diversity is associated with improved yield resilience, in terms of annual yield levels and yield variability, for winter wheat, corn, and soy. Panel data models allow us to exploit the change within counties over time to control for unobserved time-invariant heterogeneity and may reduce the risk of confounding from unobserved variables (Blanc and Schlenker 2017). For yield models the dependent variable is specified as log of yield for county
and year
. We run three separate models for corn, soy, and winter wheat and use log-yield instead of raw yields to constrain possible predicted yields to positive values (Schlenker and Roberts 2009, Roberts et al
2017). The main explanatory variables are
and the three weather variables
(Roberts et al
2017). Control variables include irrigation for county
in year
, pesticide expenses for county
in year
, and fertilizer expenses for county
in year
. The model takes the form
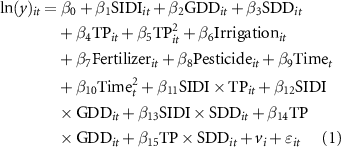
where is a quadratic time trend,
is county fixed effects, and
denotes random errors. A squared term is included for TP to account for non-linearities in the relationship between precipitation and crop yields (Rosenzweig et al
2014, Burchfield et al
2019a, 2019b). The time trend accounts for changes in yields over time that are consistent across the state of Kansas, such as yield improvements due to technological advances (Schlenker and Roberts 2009), and the county fixed effects capture unobserved heterogeneity across counties due to factors such as soil quality. In addition, we include interaction terms between diversity and weather variables, and between TP and other weather variables. The diversity-weather interaction terms (SIDI-TP, SIDI-GDD, SIDI-SDD) indicate how the effect of diversity on yields varies with changing weather conditions. Similarly, the interaction between TP and temperature variables (TP-GDD, TP-SDD) accounts for the conjoint effect of temperature and precipitation on crop yields, such as the combined high temperature and low precipitation conditions experienced during drought.
The relationship between landscape diversity and yield variability was also tested (equation (2)). Yield variability is measured by the county level coefficient of variation (CV) of yield over a three year moving window calculated as the CV of log yield, in which
is the temporal standard deviation of yields and
is mean crop yield. High values of CV indicate high yield variability or low yield stability.
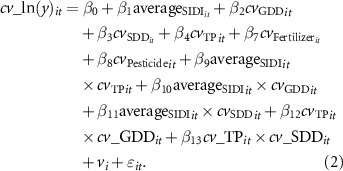
For the yield variability models we employ the three year average of our landscape diversity index, calculated as , to determine if higher levels of landscape diversity are associated with reduced crop yield variability. All other explanatory and control variables are included as coefficients of variation (e.g. variability in SDD over the three year window). Because the CV of yield may also include consistent yield growth that may be desirable to producers, we include a dummy variable to account for counties where yields consistently increase over each three year period. Hence, coefficient estimates for explanatory variables apply only to detrimental yield variability conditions without consistent growth.
Given the relatively short period covered by the dataset, our model emphasizes variability in systems that are substantively similar rather than the influence of systemic changes over longer periods of time. Therefore, the influence of short-term weather fluctuations and input use variability (rather than variability due to long-term changes in climactic conditions, management techniques or technology advances) on the relationship between landscape diversity and yield variability are more readily examined. This quantification of yield variability using three year coverage is consistent with previous literature (Redhead et al 2020) that has examined short term yield stability related to the ability of farming practices to compensate for environmental fluctuations. We also test the sensitivity of these yield variability models to length of the temporal window by running models with a two year, four year, and eleven year (cross-section) window.
All models were estimated using ordinary least squares (OLS) regession in STATA 16, and standard errors are clustered at the county level. Model sensitivity was examined by testing models with and without interaction effects and models with and without fertilizer, pesticide, and irrigation inputs. Coefficient estimates were highly consistent across model formulations, and models with interaction effects and agronomic input controls generally showed improved Akaike information criterion and adjusted R2 values.
3. Results
In Kansas the average value of the SIDI diversity index across all crops and counties was approximately 0.71, with a standard deviation of 0.1 and range from 0.36 to 0.87, over the period 2008–2018 (table 1). The average irrigation water volume by crop ranges from 21 943 to 32 042 acre-feet per county per year, and annual average fertilizer and combined herbicide and insecticide expenses for counties were approximately $65 000 and $40 000, respectively (table 1). Figure 1 shows the variation in landscape diversity in Kansas from 2008 to 2018 and suggests that landscape diversity in Kansas varies significantly across space and moderately over time (see the SI for more information).
Figure 1. Variations in landscape diversity as measured by SIDI (standardized) for Kansas counties from 2008 to 2018. Counties for which neither corn, soy, or wheat were grown are shown in gray.
Download figure:
Standard image High-resolution imageTable 1. Summary statistics for counties in which a crop was grown, for the time period 2008–2018. (Values are calculated over both space and time. Yield and water volume values in SI units are provided in parentheses).
Wheat | Corn | Soy | |||||||
---|---|---|---|---|---|---|---|---|---|
Variables | Mean | Standard deviation | n | Mean | Standard deviation | n | Mean | Standard deviation | n |
Yield (bu/acre) | 41 (∼2752 kg ha−1) | 10 (∼671 kg ha−1) | 962 | 121 (∼7610 kg ha−1) | 39 (∼2453 kg ha−1) | 786 | 37 (∼2483 kg ha−1) | 12 (∼805 kg ha−1) | 723 |
SIDI | 0.71 | 0.12 | 962 | 0.72 | 0.11 | 786 | 0.71 | 0.10 | 723 |
Water volume (acre-feet) | 31 995 (∼39.5 × 106 m3) | 56 571 (∼69.8 × 106 m3) | 959 | 32 042 (∼39.5 × 106 m3) | 56 542 (∼69.7 × 106 m3) | 784 | 21 942 (∼27.1 × 106 m3) | 45 908 (∼56.6 × 106 m3) | 720 |
GDD (°C) | 5620 | 391 | 962 | 3302 | 166 | 786 | 2992 | 133 | 723 |
SDD (°C) | 194 | 95 | 962 | 316 | 147 | 786 | 289 | 128 | 723 |
TP (mm) | 627 | 215 | 962 | 642 | 211 | 786 | 593 | 177 | 723 |
Fertilizer expenses ($) | 49 508 | 28 477 | 552 | 49 968 | 28 272 | 474 | 48 617 | 27 470 | 498 |
Pesticide expenses ($) | 42 696 | 261 834 | 552 | 42 200 | 23 676 | 474 | 40 516 | 23 909 | 498 |
3.1. Yield and diversity
Figure 2 presents the coefficient estimates of the yield models using fixed-effect regression with crop yield as the dependent variable over the period 2008–2018 as shown in equation (1). (Full model results are shown in table S1.) The estimates indicate that increases in landscape diversity within counties result in, on average, higher yields for all three crops. An increase of one standard deviation in landscape diversity is associated with, ceteris paribus, an average increase in corn and soy yields of approximately 7% and 5% respectively, while wheat yield increases reach 28% (robust standard errors indicate a range of about ±3% for each crop). This suggests that Kansas wheat is more sensitive to within-county landscape diversity changes than corn and soy. In addition, the effect of diversity on corn yields is stronger when SDDs are high, as indicated by the interaction term between SIDI and SDD (SIDI × SDD). The effect of increasing within-county diversity on corn yields is 7.9% higher when SDDs increase by one standard deviation, suggesting that landscape diversity mitigates the negative effect of high temperatures on corn yields (figure 3).
Figure 2. Coefficient estimates of the yield models for corn, soy, and wheat. Points indicate the mean estimated effect and bars indicate the 95% confidence interval. Variables are standardized using z-scores, therefore effects on log(yield) can be interpreted as approximately a percent change in the actual yield associated with a one standard deviation increase in the variable of interest. (The true coefficient effects are calculated as (exp(coefficient) − 1) × 100.)
Download figure:
Standard image High-resolution imageFigure 3. Estimated log(yield) values for corn given significant interaction effect between SDD and SIDI when all other predictor variables are held at average values (zero). Coefficient estimates are shown as colored points and robust standard errors are shown as vertical colored bars. The increased slope when SDD = 1 suggests and increase in the effect of SIDI on corn yields when SDD are high.
Download figure:
Standard image High-resolution imageCoefficient estimates for weather control variables suggest that higher values for GDDs are associated with higher yields while increased SDDs lead to lower yields, consistent with previous literature (Schlenker and Roberts 2009, Burchfield et al 2019b). The results also suggest that TP has a positive and nonlinear effect on yields, as indicated by the significance of the quadratic term added to the model (Fishman 2016 Schlenker and Roberts 2009). The significant interaction term between TP and SDD for wheat (figure S6) suggests that due to the prevalence of dryland (unirrigated) wheat production in Kansas (KFMA 2020) increased precipitation had a stronger positive impact on wheat yields in places with higher SDDs. This indicates that precipitation mitigates the negative impact of extreme heat on wheat yields, and conversely, that combined extreme heat and low precipitation (drought conditions) are associated with lower yields. In contrast, the interaction effect between TP and SDD for corn (figure S7) suggests that the positive effect of precipitation on corn yields is reduced in strength when SDDs increase. This may be due, in part, to the use of irrigation to offset precipitation shortfalls during drought conditions and in areas with lower annual precipitation (Islam 2012).
Irrigation volumes are associated with increased yields for corn and soy, but reduced yields of wheat. The strong positive impact of irrigation on corn, and to a lesser extent soy, is expected due to the high water requirements for these crops and large proportion of these crops that are cultivated using irrigation in Kansas Lamn et al 2007, KFMA 2020). Reported irrigated acres planted were 19% for corn, 7% for soy and 1% for wheat in 2017 (Kansas Department of Agriculture 2017). The negative effect for wheat is unexpected but may reflect differences in irrigation demand based on the growing season of winter wheat, which is planted in the fall and harvested in the following spring (Lamn et al 2007), or reduced water use efficiency under full irrigation in continuous wheat cropping systems (Araya et al 2017). Note that effects for irrigation lose strength when extremely high values in the tail of the distribution are removed or when irrigation values are log-transformed (table S2) suggesting some bias in our coefficient estimates due to data skew. Specifically, only corn retains a significant effect for irrigation when the top 5% of irrigation values are removed from the dataset. While the estimates for the irrigation control value are not highly reliable, they do not significantly impact estimates of other coefficients in our models (table S2).
Fertilizers and pesticide inputs were accounted for in the model, but significant effects were only found for soy. While pesticide expenses are associated with higher soy yields, fertilizer expenses are associated with lower soy yields. The lack of consistent, significant effects may be due to the expense proxy not fully capturing the actual quantities of fertilizer applied, or the inability of excessive use of inputs to overcome the negative impact of poor growing conditions or pest outbreaks on yields. Notably, models that did not include the input control variables resulted in similar coefficient estimates for other variables (table S3), suggesting model robustness to changes in these variables.
3.2. Yield variability and diversity
Figure 4 shows the estimates for yield variability models that calculate the CV of yield using a three year moving window. The results indicate that, within a county, higher levels of average landscape diversity are associated, on average, with lower yield variability (higher yield stability) for all crops, however the effect is only significant for wheat (table S4). Estimates for variables representing variation in weather suggest that increased variability in TP is associated with higher yield variability for soy and wheat but does not significantly affect corn. Variability in GDDs is associated with higher yield variability for corn and soy, while high fluctuations in SDDs are associated with reduced corn and soy yield variability and increased wheat yield variability, mirroring differences between irrigated and non-irrigated crop production observed in the yield models. The reduction in yield variability associated with higher SDD variability for corn and soy is counterintuitive yet may be the result of reliance on irrigation to counteract adverse growing conditions in areas of the state where more extreme SDD values are experienced (figure S8), providing yield stability by partially disassociating yields from weather conditions (Burchfield and Nelson 2021).
Figure 4. Coefficient estimates of the yield variability (three year) models for corn, soybean, and wheat. Points indicate the mean estimated effect and bars indicate the 95% confidence interval.
Download figure:
Standard image High-resolution imageHigher variability in irrigation volumes is associated with increased yield variation for both corn and wheat, but with reduced yield variation for soy. This unexpected finding may be related to differences in where soy is grown in the state (figure S4). Model robustness checks in which irrigation and input control variables were removed (table S5) suggest that the inclusion of the irrigation variability control variable does not significantly affect our findings for our primary variable of interest (average SIDI). Fertilizer and pesticide variability control variables do not show significant effects in our main yield variability model; however removal of these controls does alter coefficient estimates (table S5), suggesting that variability in chemical inputs is correlated with landscape diversity. This may be the result of ecosystem services provided by higher landscape diversity that alter input requirements and use.
Interaction terms suggest that for wheat, the positive effect of higher landscape diversity on yield variability is slightly weaker when there is greater variability in precipitation (table S4, figure S9). In other words, while higher landscape diversity may be associated with reduced yield variability for wheat, it does not reduce the effect of precipitation variability on wheat yield variability. Models run without inclusion of the interaction effects (table S6) show no significant changes in other coefficient estimates.
Yield variability models run with a 2 year or 4 year moving window, or as an 11 year window cross-sectional model (table S7) suggest that selection of the temporal frame does alter model results, however some overall trends are consistent. Weather variability control variables tend to have a stronger association with yield variability when shorter temporal frames are considered, suggesting that extreme fluctuations in weather are associated with in-season or in-year impacts to yields that recover quickly in the following year. Similarly, more significant associations between average diversity and yield variability are observed for shorter temporal frames. In addition, in all versions of the model significant associations between average diversity and yield variability indicate that higher levels of landscape diversity are associated with reduced yield variability.
4. Discussion
The world's major agricultural regions, including the United States, have experienced recent yield losses due to climate variability and extreme weather events (Lobell et al 2011, Ray et al 2015, Renard and Tilman 2019) with yield variability estimated to explain more than 30% of global crop yields and more than 60% of the yield variability in some parts of the world (Ray et al 2015). In the face of increasing climate variability improving yield resilience to climate conditions has been discussed as a necessary component of tackling world food demand challenges (Ray et al 2015, Renard and Tilman 2019). Development of diversified agricultural landscapes have been widely called for as a way to reduce the use of synthetic inputs and increase ecosystem health while ensuring economic and environmental sustainability (Hertel et al 2021, Smith et al 2008, Viguier et al 2021).
In this study, we estimated the effects of landscape diversity on crop yield resilience by examining the relationships between landscape diversity and yield and yield variability in the state of Kansas. Our estimates show that landscape diversification has significant effects on aggregate county-level crop yields; winter wheat yields increase by 28% in highly diversified counties and corn and soy yields increase by 7% and 5%, respectively. Notably our models indicate that even after controlling for agricultural inputs, and when examining only a single state with limited contextual variation, in comparison to similar national models (Burchfield et al 2019b, Merlos and Hijmans 2020, Nelson and Burchfield 2021), landscape diversification is associated with increased crop yield and yield variability. The average level (∼0.7) and standard deviation (∼0.1) of SIDI in Kansas are very similar to those observed nationally (Nelson and Burchfield 2021) suggesting that Kansas may be a reasonable proxy for many other US agricultural systems. As significant associations between landscape diversity and yield resilience are observed within Kansas it seems likely that these same trends apply across many US agricultural systems. Because our analysis is constrained to the state of Kansas our findings also provide some evidence that landscape diversity-yield trends are not simply the product of radically different cropping systems (e.g. Central Valley v. Corn Belt) that are contained within national scope analyses (Nelson and Burchfield 2021), but truly reflect the aggregate impact of diversity of land-cover on average crop yields. These findings further strengthen the mounting evidence that landscape diversity can positively impact aggregate yields for individual crops and point to the reproducibility and robustness of this relationship. Moreover, we find that not only are higher levels of landscape diversity associated with improved yields, and in some cases improved yield stability, these higher levels of landscape diversity can also mitigate the impacts of adverse weather conditions on crop yields, increasing yield resistance to environmental fluctuations.
On average, Kansas crop yields were shown to be sensitive to landscape diversification, indicating that increases in spatial land-cover diversity within counties may improve yield outcomes. Consistent with previous literature (Smith et al 2008, Burchfield et al 2019b, Nelson and Burchfield 2021) our results suggest that landscape diversification has a stronger impact on winter wheat than corn, while soy yields are less responsive to diversification. These variable relationships may be the product of differences in factors including crop management, such as more intensive use of chemical inputs and irrigation for corn and soy, that might mask the positive effects of landscape diversity; use and type of crop rotations and associated soil quality benefits; length and timing of growing seasons; differences in growing locations; or sensitivity to pests and diseases such as wheat leaf rust. However, we note that while our models add to the evidence that landscape diversity relationships with yield resilience vary by crop, they do not elucidate the specific mechanisms behind these variations.
Our main yield variability model suggests that increasing average diversity is associated with reduced yield variation of wheat yields while our alternate yield variability models suggest that this relationship also exists for corn, and to a lesser extent, soy. Diversification has been promoted to improve agricultural production stability (Gaudin et al 2015, Renard and Tilman 2019, Hertel et al 2021). However, this effect is more clearly associated with 'insurance' or 'portfolio' processes (Birthal and Hazrana 2019, Renard and Tilman 2019) and may not be realized for a single crop type. Our results, however, suggest that spatial diversity may provide a stabilizing effect on individual crop yields. Like Redhead et al (2020) our models indicate that this stabilizing effect is most apparent when considering short time periods and suggest that landscape diversity may reduce the impact of short-term extreme weather fluctuations when management strategies cannot fully compensate for impacts on yields.
Several limitations of our approach should be noted. Agronomic input data approximated via fertilizer and herbicide/insecticide expenses are available at the county-year level, but breakdowns of these inputs and irrigation volumes used by crops are not available. While these input data provide a more thorough analysis of the cropping system, they are also subject to a large degree of uncertainty. Fertilizer and pesticide expenses are an indirect estimate of input use which may vary over time and by location based on local differences in pricing. Relatedly, the irrigation volumes used are subject to inaccuracies in self-reporting of irrigation water use. This study also did not consider other agronomic aspects such as the potential to adopt different cultivars and potential conservation practices.
Ultimately, our analyses suggest that the agronomic input variables used in our models had small and inconsistent effects on crop yields. Correlations between these control variables do not indicate multicollinearity that might influence our estimates for these variables (table S8). In addition, while inclusion of these input variables in our models resulted in equivalent or improved model fit (table S9), they had a limited impact on estimates of the effect of landscape diversity on crop production resilience in terms of either gross yields or yield variability. As agronomic inputs are clearly important for crop production (Oerke 2005, Kang et al 2009), this finding may reflect a lack of consistent variability in these factors within counties over time, while strong spatial variation in input use was absorbed by our use of county-level fixed effects. Alternatively, changing input use within counties over years may have had little impact on crop yields due to oversaturation, where input use is already at or exceeding optimal levels and further application provides little to no added benefit (Mózner et al 2012, Burchfield and Nelson 2021). Relatedly, intra-year dynamics related to input use, such as applying pesticides because of an ongoing outbreak which is decreasing yields, may mask the influence of inputs on yields observed at a county-annual basis. While we did not find significant effects for agronomic inputs, controlling for these factors are important to avoid parameter bias, particularly for broad-scope analyses where management practices and cropping system structures vary more widely.
A limitation of use of SIDI as a measure of agricultural landscape diversity is that, while related, the diversity metric does not explicitly account for spatial configuration of land-covers, which may influence disease spread and pest habitat (Martin et al
2019, Redhead et al
2020, Nelson and Burchfield 2021). However, given that landscape compositional diversity, which SIDI provides a measure of, has been shown to be more strongly associated with crop yields than landscape configuration our findings likely capture much of the expected effect of overall landscape complexity (Nelson and Burchfield 2021). SIDI also does not account for possible agricultural producer changes to their land-use during the growing season which may impact observed annual land-cover datasets, and hence, calculated landscape diversity.In this study we assume that observed land-cover changes are primarily driven by changes in land-use from year to year. However, cover cropping, double cropping, and mid-season cultivation changes with the potential to alter crop yields (or that occur in response to expected crop yields) and alter land-cover patterns mid-year can and do occur with regularity (Beal Cohen et al
2019, Bowles et al
2020, Marini et al
2020, Garland et al
2021), yet are not currently considered in existing metrics of spatial landscape diversity. Moreover, year-to-year temporal diversity via common practices such as crop rotations are not directly considered in spatial landscape diversity metrics. These temporal diversity aspects are both clearly linked with spatial landscape diversity and crop yields, therefore how temporal aspects of land-cover change influence assumptions about landscape diversity is a critical area for future research.
5. Conclusion
With the projected ten billion global population by 2050, the demand for food production is projected to increase approximately by 70% (Popp et al 2013). While crop productivity may be increased by application of fertilizer along with other factors such as improved water and soil management, there are limits to what input-intensive management practices can provide (Redhead et al 2020). In a changing climate agricultural diversification may play an important role in boosting crop resilience by supporting pest management, pollination services, and soil and nutrient management through the provision of ecosystem services and altered management practices (Claflin et al 2017, Dainese et al 2019, Crossley et al 2021, Hertel et al 2021).
This study highlights the importance of landscape diversity for crop production resilience. Significant effects of landscape diversification on crop yields were demonstrated, even after accounting for weather, agricultural inputs, and irrigation. In addition, increases in landscape diversity had a significant effect on yield variability, and a moderating effect on adverse weather conditions, indicating a significant effect of landscape diversification on yield resilience with the potential to significantly impact crop production in Kansas. Although these results do not identify the mechanisms through which the observed effects occur, they may arise from a combination of ecosystem service provisioning across landscapes (landscape services) or altered management practices (Crossley et al 2021). Specifically, these effects may be because diversification induces high agroecological benefits resulting from improved soil conditions, decreased soil erosion, and increased nutrient circulation, which improves yield, and yield stability under changing climatic conditions (Kremen and Miles 2012). Effects may also arise from changes to pest and natural predator habitats, reduction of pest and disease spread, or agricultural producer land management changes associated with landscape diversification (Smith and Gross 2007, Meehan and Gratton 2016).
Regardless, the findings suggest that even within a relatively low diversity state with many similarities with agricultural production regions in the United States and across the world, increasing landscape diversity is positively associated with crop production resilience. Because agricultural systems within the state of Kansas are substantively similar (relative to those in national studies) it follows that this positive association is the result of some causal mechanism, likely ecosystem services or management strategies, that is active in diverse landscapes. Overall, we provide further evidence that landscape diversification may become an important part of a suite of policy tools for increasing crop resilience under changing climate conditions.
Acknowledgments
The authors gratefully acknowledge Dr Emily Burchfield for sharing data used in this study. This work was partially funded by USDA National Institute of Food and Agriculture Grant No. 2020-67019-31157. Any opinions, findings, conclusions, or recommendations expressed in this publication are those of the authors and do not necessarily reflect the view of the US. Department of Agriculture.
Data availability statement
The data that support the findings of this study are available upon reasonable request from the authors.
Supplementary data (1.3 MB PDF)