Abstract
A novel approach for satellite-based comprehensive national tree cover change assessment was developed and applied in Bangladesh, a country where trees outside of forests play an important role in the national economy and carbon sequestration. Tree cover change area was quantified using the integration of wall-to-wall Landsat-based mapping with a higher spatial resolution sample-based assessment. The total national tree canopy cover area was estimated as 3165 500 ± 186 600 ha in the year 2000, with trees outside forests making up 54% of total canopy cover. Total tree canopy cover increased by 135 700 (± 116 600) ha (4.3%) during the 2000–2014 time interval. Bangladesh exhibits a national tree cover dynamic where net change is rather small, but gross dynamics significant and variable by forest type. Despite the overall gain in tree cover, results revealed the ongoing clearing of natural forests, especially within the Chittagong hill tracts. While forests decreased their tree cover area by 83 600 ha, the trees outside forests (including tree plantations, village woodlots, and agroforestry) increased their canopy area by 219 300 ha. Our results demonstrated method capability to quantify tree canopy cover dynamics within a fine-scale agricultural landscape. Our approach for comprehensive monitoring of tree canopy cover may be recommended for operational implementation in Bangladesh and other countries with significant tree cover outside of forests.
Export citation and abstract BibTeX RIS

Original content from this work may be used under the terms of the Creative Commons Attribution 3.0 licence.
Any further distribution of this work must maintain attribution to the author(s) and the title of the work, journal citation and DOI.
1. Introduction
The natural vegetation of Bangladesh, a South Asian country located largely in the floodplains of the Padma, Jamuna, and Meghna river systems, consists of tropical moist deciduous and semi-evergreen forests, mangroves, and freshwater wetlands (Olson et al 2001). The natural ecosystems of the country are rich in plant and animal diversity. The Chittagong Hill Tracts forests at the border with Myanmar are related to the Indo-Burma biodiversity hotspot, one of the few globally significant areas with high species diversity and endemism (Myers et al 2000). The country is home to a large portion of the Sundarbans, the world's largest contiguous mangrove forest, named a UNESCO World Heritage site in 1997. Most of the natural forest vegetation within the country has long been cleared for agriculture uses. Only 11% of the country is still covered by forests, according to the latest Forest Resources Assessment (FRA) report by the Food and Agriculture Organization of United Nations (FAO 2015), making Bangladesh a forest-poor country. With a population of 156 million people and the forest area below 1.5 million ha, forested land per capita (0.009 ha person−1) is one of the lowest in the world (FAO 2015). Growing demands for timber, fuelwood, as well as for agriculture land expansion, represent tremendous pressure on the remaining forests. Recent research (Reddy et al 2016) showed that more than 39% of the Bangladesh natural forest area has been lost since 1930, with the highest loss rate within the semi-evergreen hill forests of the Chittagong region.
In contrast to the high levels of natural forest conversion, the area of planted trees (including industrial and small-scale tree plantations, village woodlots, and homestead trees) within the country is increasing. Since the 1980s a number of governmental programs, as well as campaigns organized by non-governmental organizations, are focused on the establishment and sustainable management of trees outside forests, which includes support for agroforestry systems, expansion of village woodlots, and tree planting on degraded lands, near houses, and along roads (Zashimuddin 2004). In addition to 'village forests', industrial forest plantations are also expanding in response to high demand for timber. Another important governmental investment, which started with the coastal 'green belt' project in the 1980s, supports mangrove afforestation along the coastlines, especially on newly formed land within coastal estuaries (Biswas and Choudhury 2007). According to the country report to the FAO FRA 2015, the national afforestation area grew from 4500 ha per year in the 2000s to 7300 ha per year in the 2010s. The mangrove plantations grew especially fast; planted mangrove area increased four times from 1990 to 2015. Correspondingly, the living forest biomass within the country increased by 34 million tons from 1990 to 2015 (FAO 2015).
Household and community tree plantations play an important role in timber and non-timber products supply for rural residents, who represent three quarters of the total country population. More than half of national wood production comes from trees outside forests. This proportion is even higher for fuelwood (>70%) and bamboo (>80%) supply (Islam 2004). The production from the village woodlots makes a large contribution to household income and is considered an effective way to reduce poverty in rural communities (Zashimuddin 2004). In addition to provisional functions, tree cover increase within agriculture areas and settlements provides a number of other ecosystem services like water purification, erosion prevention, and biodiversity support. Another important function of trees outside forests is carbon sequestration. Based on the national forest inventory of 2005–2007, 73% of the total aboveground tree biomass in Bangladesh was found within trees outside forests (Schnell et al 2015). Tree plantation expansion plays an important role as a major terrestrial carbon sink. The land use system in Bangladesh (Ahmed et al 1996) and in South Asia as a whole (Patra et al 2013) has been shown to be a carbon sink. Unfortunately, the carbon sink in forests and other ecosystems does not outweigh emissions from fossil fuel combustion, making the region a net source of greenhouse gasses (Patra et al 2013). Forest restoration projects coupled with investments in alternative energy sources and energy conservation technologies are needed to reduce the carbon footprint of South Asian countries.
Timely, operational forest monitoring is a baseline requirement for effective national forest management, national and international reporting. Forest extent and change area are the primary data required for national reporting on greenhouse gas emissions according to the Intergovernmental Panel on Climate Change guidelines (IPCC 2006). Periodic global and regional forest statistics reports, like the FAO FRA, rely on accurate data on forest extent and resources provided by countries. Such data are also required in the context of the international negotiations on Reducing Emissions from Deforestation and Forest Degradation (REDD+). The Bangladesh Forest Department (BFD), a part of the Ministry of Environment and Forests, is the government agency responsible for national forest monitoring. The BFD has conducted forest mapping using remotely sensed data since the mid-1990s. In the 1990s and early 2000s, forest mapping was limited to specific forest areas. The first in situ national forest assessment was performed by the BFD in 2006–2007, consisting of 296 sample plots distributed across the country. Recently, a new national forest inventory was organized with support from the FAO. The inventory data, including aboveground and belowground biomass, are being collected for 1858 clusters of plots across the country. BFD is expected to complete the inventory by 2018. In addition to national forest mapping projects, the international research community has created a number of satellite-based products depicting forest extent and change, as well as other land cover dynamics for Bangladesh over recent decades (Giri and Shrestha 1996, Hansen et al 2013, Reddy et al 2016). The most recent analysis performed by the Indian National Remote Sensing Centre (Reddy et al 2016) mapped forest extent and change from 1975 to 2014 using Landsat, LISS, and AWiFS satellite data. These map products, however, only considered natural forest area and ignored dynamics of trees outside of forest.
The importance of trees outside of forests and the continuing loss of remaining natural forests required the Bangladesh government to establish a comprehensive tree canopy cover monitoring capability. Traditionally, national forest mapping projects did not include trees outside forests in analyses or were limited in reporting about their extent and change. Quantification of the heterogeneous and dynamic cover of planted trees is challenging, especially if performed at the national level. Satellite data provides practical means for national tree canopy cover monitoring. India, as well as a number of other South Asian countries, uses satellite data for national forest assessments (Schnell et al 2015). However, the use of freely available medium spatial resolution data (e.g. Landsat) as the sole input for a comprehensive tree cover monitoring may not be optimal in a highly heterogeneous landscape with fine-scale dynamics, like Bangladesh. An integration of wall-to-wall medium-resolution mapping with higher spatial resolution sample-based assessment, as done in Peru (Potapov et al 2014) may be suitable to estimate area and changes in tree canopy cover with high precision.
In February 2015, the Resources Information Management System (RIMS) Unit of the BFD in collaboration with the Global Land Analysis and Discovery (GLAD) Lab of the University of Maryland started a new project for conducting comprehensive tree cover extent and change mapping in Bangladesh. The initiative was supported by SilvaCarbon, a USA inter-agency program that is working on enhancing the capacity of developing countries for monitoring and managing forest and terrestrial carbon. The overarching project goal was to enhance the RIMS capacity in performing operational tree canopy cover monitoring at national extent. The technical objective was to map and quantify the change in tree cover from 2000 to 2014 and to create a baseline for future bi-annual tree cover monitoring. The tree cover mapping and monitoring system implemented by RIMS Unit and GLAD Lab was based on the integrated use of wall-to-wall Landsat-based mapping and sample-based area estimation using freely available high spatial resolution imagery and Landsat time-series data. Our results demonstrated the capacity of the method to quantify tree canopy cover dynamics within different forest types and outside forests. We believe that similar approach for comprehensive monitoring of tree cover may be implemented within countries having considerable tree cover outside of natural or managed forests.
2. Data and methods
A fine-scale mosaic of settlements, woodlots, orchards, crops, pastures, and aquaculture forms the Bangladesh agricultural landscape. Tree canopy cover is highly fragmented, and changes usually happen at fine scales, from single tree planting/removal to small village woodlot management. Landsat data with a spatial resolution of 30 m is not capable of capturing the full spectrum of variations in canopy cover or detecting small changes (Tyukavina et al 2013). Higher spatial resolution data (1–5 m) are suitable for this task; however, such data are expensive to purchase at a national scale. Another problem is the absence of a national coverage of high-resolution data in the early 2000s. To overcome data limitations, we established a two-stage method for national tree cover monitoring. Wall-to-wall Landsat-based tree cover extent and change maps were created during the first stage. These maps served to stratify Bangladesh in the implementation of a stratified random sampling protocol. The second stage of the analysis consisted of characterizing tree cover area and change based on samples of multi-resolution time-series data.
For the first stage, we used the entire Landsat archive for Bangladesh to derive nationally consistent input time-series data using the method developed by Potapov et al (2012) and implemented at the global (Hansen et al 2013) and national (Potapov et al 2014) scales (figure 1). Using Landsat data for the year 2000, we mapped pixels with greater than 50% tree cover to create a dataset hereafter referred to as the '>50% tree cover extent stratum'. The >50% tree cover extent stratum map guided the change detection for the 2000–2014 time interval. A 'gross tree cover loss stratum' was defined as pixels with greater than 50% tree cover in the year 2000 that lost tree cover from 2000 to 2014 (even if they gained tree cover by the year 2014). A 'gross tree cover gain stratum' was defined as pixels outside the year 2000 '>50% tree cover extent stratum' that increased canopy cover above 50% by the year 2014. The gross tree cover loss and gain stratum maps were produced independently.
Figure 1. National Landsat cloud-free time series data were used as an input for wall-to-wall mapping and reference sample interpretation. Shown here are annual cloud-free national mosaics for the years 2000, 2007, and 2014 in SWIR-NIR-Red band combination.
Download figure:
Standard image High-resolution imageThe second stage consisted of sample-based area estimation of tree canopy cover and dynamics. We selected and analyzed the sample following the 'good practice' guidance (Olofsson et al 2013, Olofsson et al 2014). To increase sampling efficiency and to reduce the uncertainty of the estimate we employed stratified random sampling design using the Landsat-derived wall-to-wall maps as sampling strata. A set of samples consisting of 30 × 30 m Landsat pixels was visually interpreted to estimate fractional (% of pixel area) tree canopy cover, canopy loss and gain, as well as forest type. Sample interpretation was done using high spatial resolution data from Google Earth and Landsat data time-series available from a built-for-purpose web interface (figure 2). Tree canopy cover was defined as crown cover from vegetation above 5 m tall. The result of the sample interpretation allowed us to produce unbiased area estimates of tree canopy cover, gross loss and gain, and net change with known uncertainties, and to disaggregate these areas by forest type. In addition, we utilized sample data to estimate the accuracy of the Landsat-based wall-to-wall strata maps. Detailed description of the national-scale Landsat data processing, wall-to-wall mapping, and sample-based tree canopy cover and change quantification are presented in the Appendix.
Figure 2. Reference Landsat data example (24° 27' 57.15'' N; 90° 3' 11.25'' E). Image subsets represent cloud-free annual Landsat data composites centered at the sample pixel (outlined in yellow). The graph shows annual tree canopy cover (green), annual minimal normalized difference vegetation index (NDVI) value (white) and annual maximal shortwave infrared band reflectance (red). In this example (forest plantation), tree cover loss was detected twice, in the year 2002 and 2012. The tree cover has not yet recovered by the year 2014.
Download figure:
Standard image High-resolution imageThe RIMS Unit and the GLAD Lab performed the project jointly. The input Landsat data were processed by the GLAD team and consistent time-series data were shared with RIMS along with the image characterization software. A set of training sessions was organized by the GLAD team to train and port the method to the RIMS Unit, with a focus on image interpretation and national-scale mapping. The RIMS Unit, using high-performance desktop servers provided by SilvaCarbon, performed national Landsat-based tree cover extent and change strata mapping. A sample-based assessment was performed by the RIMS Unit with support from the GLAD Lab. The training and infrastructure will enable RIMS to continue national tree cover monitoring in the future using annual Landsat data supplied by the GLAD Lab.
3. Results
3.1. Tree canopy cover for the year 2000
The sample-based estimate of the total area covered by tree crowns in the year 2000 (with 95% confidence interval) was 3165 500 ± 186 600 ha. The total tree canopy cover in 2000 equaled to 21% of the country area (almost 23% if only land area is considered).
The total tree canopy cover area was disaggregated by forest type using reference information collected for each sample pixel (table 1). Tree canopy cover within forests (which includes hill, sal, swamp forests, and mangroves) was estimated at 1464 000 ha and made up 9.8% of the country area. The area of natural sal and swamp forests was very small, hence the low precision of the estimate. More than a half of total tree canopy cover (54%) within the country was from trees outside forests.
Table 1. Tree canopy cover area within Bangladesh in the year 2000 by forest type estimated from the sample.
Forest type | Estimated area, thousand ha | 95% confidence interval, ± thousand ha | Proportion of the total tree cover area, % |
---|---|---|---|
Hill forests | 937.9 | 153.1 | 29.6 |
Mangroves and mangrove plantations | 503.0 | 92.6 | 15.9 |
Sal forests | 16.6 | 18.9 | 0.5 |
Swamp forests | 6.6 | 13.0 | 0.2 |
Trees outside forests | 1701.4 | 174.6 | 53.8 |
Total tree canopy cover | 3165.5 | 186.6 | 100.0 |
The wall-to-wall >50% tree cover extent stratum for the year 2000 provides an overview of tree canopy cover distribution within the country (figure 3). The large patches of natural forests remaining within Bangladesh include the Sundarbans mangroves and Chittagong Hill Tracts forests. In the rest of the country, natural and planted tree cover exists within the fine-scale mosaic of agricultural lands and settlements. The sample reference data were used to assess the quality of our Landsat-based >50% tree cover extent stratum map. The reference data were converted into two binary categories of 'tree cover' and 'treeless' pixels using a 50% tree cover threshold to enable comparison with the Landsat-based map. The Overall accuracy of the classification was 90.3% (standard error (SE) 0.9%), with User's accuracy of 88.3% (SE 1.6%), and Producer's accuracy of 67.1% (SE 2.4%). Validation results revealed that that the binary Landsat-scale map tends to omit tree cover area. Nevertheless, the Landsat-scale wall-to-wall strata map allowed us to implement stratified sampling design which produced the unbiased national tree cover area estimate with relatively small uncertainty (± 5.9% of the total estimated area with the 95% confidence).
Figure 3. The year 2000 >50% tree cover extent stratum and gross tree cover loss and gain 2000–2014 strata mapped using the Landsat data.
Download figure:
Standard image High-resolution image3.2. Tree cover dynamics from 2000 to 2014
The total gross tree cover loss area estimated using sample-based assessment is 272 600 (± 64 900) thousand ha (table 2). The gross loss represents 8.6% of the year 2000 tree canopy cover. Of the total gross tree cover loss area, 17.9% (48 700 ± 18 800 ha) restored tree cover by 2014. These areas represent tree rotation within plantations, shifting cultivation and agroforestry systems, as well as tree plantation establishment after clearing of natural forests. The gross tree cover gain, measured outside areas covered with trees in the year 2000, is estimated to be 359 600 (± 95 000) ha, or 11.4% of the year 2000 tree cover. The net tree cover change is estimated at +135 700 (± 116 600) ha and represents an overall increase of the tree canopy cover within the country by 4.3% during the 2000–2014 time interval. The 95% confidence interval for the national net tree canopy cover change estimate is quite large (86% of the estimate). Such high relative uncertainty was expected in the highly heterogeneous landscape of Bangladesh, where change (both loss and gain) is pervasive and individual change patches very small. The resulting net change area is small compared to the country land area.
The observed tree covers dynamic (table 2, figures 4 and 5) results in different net change area between forest types. Gross tree cover loss is mostly found within hill forests (which include natural forest stands and shifting cultivation areas), contributing 56.5% to the total loss. Hill forests have low tree recovery rate and small tree gain area. As a result, hill forests experience net tree cover loss (−80 800 ± 61 400 ha, or 8.6% of their year 2000 area). Trees outside forests (village forests, orchards, tree plantations) are characterized by strong net tree cover increase. Their area increased by 219 300 ± 98 200 ha (12.9% increase compared to their year 2000 area). Mangroves do not experience net tree cover change, with loss and gain area balancing each other. Tree loss and gain within mangrove forests are spatially separated: while some patches of mangroves were flooded or cleared for agriculture, new plantations were established on newly formed land in the river estuary. Sal forests do not experience significant tree cover area changes. No changes are detected in swamp forests, mostly due to their small size, which precludes correct estimation using national-scale sampling.
Table 2. Sample-based estimates of tree canopy cover change area in different forest types of Bangladesh from 2000–2014. Sample-based results include 95% confidence interval (shown in parentheses).
The year 2000 tree canopy cover, thousand ha | Gross tree canopy cover loss, thousand ha | Tree canopy cover gain within areas of loss, thousand ha | Gross tree canopy cover gain, thousand ha | Net tree canopy cover change, 2000–2014, thousand ha | |
---|---|---|---|---|---|
Hill forests | 937.9 (153.1) | 153.9 (51.5) | 35.8 (18.2) | 37.3 (28.0) | −80.8 (61.4) |
Mangroves | 503 (92.6) | 33.4 (7.2) | 0.6 (0.8) | 30.5 (18.2) | −2.3 (19.6) |
Sal forests | 16.6 (18.9) | 0.5 (1.0) | 0 (0) | 0 (0) | −0.5 (1.0) |
Swamp forests | 6.6 (13.0) | 0 (0) | 0 (0) | 0 (0) | 0 (0) |
Trees outside forest | 1701.4 (174.6) | 84.8 (40.2) | 12.2 (5.0) | 291.9 (89.4) | 219.3 (98.2) |
Total | 3165.5 (186.6) | 272.6 (64.9) | 48.7 (18.8) | 359.6 (95.0) | 135.7 (116.6) |
Figure 4. Tree canopy cover area for the years 2000 and 2014 per forests category. 'Forests' include hill, sal, and swamp natural and managed forests. Mangrove plantations are considered a part of the mangroves forest type.
Download figure:
Standard image High-resolution imageFigure 5. Gross tree canopy cover loss and gain 2000–2014 for different categories of forests. 'Rotation' stands for tree cover gain after the loss event and includes gain of trees outside forests on the place of natural forests. 'Forests' include hill, sal, and swamp natural and managed forests. Mangrove plantations are considered a part of the mangroves forest type.
Download figure:
Standard image High-resolution imageMap accuracy metrics showed that both Landsat-based gross tree cover loss and gain strata maps have high omission rates (table 3). Overall accuracy is high, however, because the change area is very small (less than 2% of the country land area). The main reason for the loss and gain omission was the small scale of change events, which in most cases did not affect an entire Landsat pixel. Sample-based analyses that employ high spatial resolution satellite images as reference data are possibly the only way to accurately estimate tree canopy cover loss and gain within highly heterogeneous forest landscapes characterized by small-scale change dynamics as those within Bangladesh.
Table 3. Map accuracy metrics for the 2000–2014 gross tree canopy cover loss and gain strata maps.
User's accuracy (SE) | Producer's accuracy (SE) | Overall accuracy (SE) | |
---|---|---|---|
Gross tree cover loss map | 67.5 (2.4) | 41.7 (5.8) | 98.4 (0.3) |
Gross tree cover gain map | 63.8 (2.6) | 20.2 (3.6) | 97.8 (0.4) |
Annual gross tree canopy cover loss area was quantified using the sample pixels where interpreters identified the date of the disturbance event. Only a handful of tree cover loss samples were found in the 'core no tree cover loss' stratum (18 samples, with 12 of them having tree cover loss proportion below 50% of the sample area). As such, they were not deemed representative of the overall trend. We decided to analyze annual change dynamics using only samples that were mapped as tree cover loss ('core' and 'peripheral tree cover loss' strata) and 1 pixel buffer around such areas ('peripheral no loss' stratum). The annual gross tree cover loss proportion estimates were smoothed using a 3 year rolling average filter to remove the annual area estimation inconsistency due to a small number of samples available for each year (figure 6). The tree cover loss area almost doubled from 2001 to 2006. Observed increase in the annual change dynamics, however, may be affected by unequal availability of the high resolution data which are biased towards more recent years. The annual tree cover loss area was relatively stable during the 2006–2014 interval.
Figure 6. Annual percent of the total gross tree canopy cover loss area. The percent estimated only within areas mapped as tree cover loss and 1 pixel buffer around such areas. The annual percent estimates were smoothed using a 3 year rolling average filter, and the uncertainties were propagated.
Download figure:
Standard image High-resolution image4. Discussion
The presented method of national tree cover assessment based on the integration of wall-to-wall medium-resolution mapping and sample-based analysis allowed us to quantify tree cover area and change fast and with sufficiently small uncertainty. We compared our sample-based estimates with the official national forest area for the year 2000 that was reported by the BFD to FAO FRA (FAO 2014) (table 4, figure 7). The sample-based estimate of tree canopy cover within forests (including hill, sal, swamp, and mangroves forests) is almost the same compared to the FAO report. The BFD reported 1468 000 ha of forest area while we estimated 1464 000 ha of tree canopy cover within forests and mangroves, which is 0.3% smaller compared to the national report. However, the difference in tree cover estimation within forests for the year 2014/2015 is high. Based on our net tree cover change results, we estimated the year 2014 tree cover area within forests as 1380 000 ha. The BFD forest area estimate for the year 2015 is 1429 000 ha, which is 3.4% higher compared to our result.
Table 4. The year 2000 national forest tree cover area estimates from this study (sample-based assessment) and from the national statics reported to the FAO FRA (FAO 2014). The area is shown in thousand ha. Sample-based results include 95% confidence interval (shown in parentheses).
Sample-based estimate | FAO FRA national report | ||
---|---|---|---|
Hill, swamp, and sal forests | 961.1 (± 154.8) | Forest | 1468 |
Mangroves forests and plantations | 503.0 (± 92.6) | ||
... from which mangroves | 476 | ||
Trees outside forests | 1701.4 (± 174.6) | Other wooded lands | 279 |
Other land with tree cover | 934 | ||
Total | 3165.5 (± 186.6) | Total | 2681 |
Figure 7. Comparison of the national tree canopy cover estimates by forest type for the year 2000 with the year 2000 national forest reporting (FAO 2014). For the current product ('RIMS Tree Canopy Cover Assessment'), F stands for forests (including hill, sal, and swamp forests and forest plantations); M stands for mangroves and mangrove plantations, and TOF stands for trees outside forests. For the FAO FRA report, F stands for forests (excluding mangroves), M stands for mangroves and mangrove plantations, W for other wooded areas, and R stands for other land with tree cover.
Download figure:
Standard image High-resolution imageThe highest disagreement between our tree cover area estimate and the official report is for the trees outside forests. For the year 2000, our sample-based estimate of canopy cover from trees outside forests is 28.7% higher comparing to the official estimate that includes wooded areas and other land with tree cover. The high disagreement was observed when comparing the change in tree cover within wooded and other lands. For the year 2000, our sample-based estimate reported 484 000 ha more tree cover compared to the national statistics. However, for the year 2015, the area covered by trees outside forests reported by BFD is 778 000 ha higher compared to our estimate for the year 2014. Observed differences in estimation of tree canopy cover outside forest areas illustrate the need for a consistent, statistically valid, periodic sample-based assessment that are based on standard set of definitions and employs high spatial resolution satellite data. The integration of wall-to-wall mapping and sample assessment presented here may be beneficial to improve the spatial and temporal consistency of the national tree canopy cover and change reporting.
The quality of a satellite-based wall-to-wall national tree cover and change mapping depends on data availability, consistency, and their suitability to map land cover dynamics. As it was shown earlier (Hansen et al 2013, Potapov et al 2012, Potapov et al 2014), spatially and temporally consistent Landsat input data is the key to large-scale tree cover extent and change mapping. A Landsat-based wall-to-wall mapping method was implemented in Bangladesh resulting in the national tree cover extent and change strata maps. Landsat's spatial resolution, however, is not optimal for mapping small-scale changes within heterogeneous landscapes. The low accuracy of our Landsat-based products is a consequence of this limitation. The high omission rates of tree cover extent and change strata maps were due to the landscape heterogeneity and the fine scale of change events that in most cases did not affect an entire Landsat pixel. Tree cover gain was mostly found within trees outside forest (81% of total gain area) where it was pervasive and usually located on the boundaries between woodlots and croplands, near houses, and along roads. Landsat data was not suitable to detect such small change events. Using wall-to-wall high spatial resolution data (<5 m per pixel) will better suit national change mapping. However, using such data will require considerable effort in data preparation (especially if data from different sensors are used), and substantial investments to purchase a complete national data coverage.
The availability of high spatial resolution image time series is the most important factor to consider for national sample analysis. Bangladesh stands out as a country having excellent high-resolution data coverage available through the Google Earth platform, especially during late years (2010–2014) of our analysis. Some problems, however, hamper image interpretation. The high-resolution data availability is inconsistent year to year. Unlike Landsat data, there is no systematically acquired high spatial resolution image archive. Along this line, good data availability was found for recent years while images for the early years of our analysis (2000–2004) were rare. Out of 1000 samples used to estimate tree canopy cover for the year 2000, 540 lacked high-resolution data earlier than 2006. The interpretation of canopy cover proportion within sample was done using the earliest high-resolution image available on Google Earth. For samples which lack circa 2000 images, interpreted tree canopy cover proportion may be incorrect due to changes that may have happened between the year 2000 and the date of the earliest image.
The lack of high resolution data in the early years of analysis may also affect gross tree cover loss detection. Out of 1506 samples used to estimate gross tree cover loss area, 48% do not have high resolution data before the year 2006. We assume that an image analyst may have missed some of the earlier change events given that most of the high-resolution images were from later years. The lack of high resolution data is especially critical for detection of small-scale sub-pixel disturbances that may not be consistently detected using Landsat data. Small-scale tree cover disturbance and recovery events are very common in Bangladesh as most of the tree cover represents trees outside of forests that are found within a fine-scale heterogeneous rural landscapes. Out of all samples where gross tree cover loss was detected, 36% were interpreted as having sub-pixel disturbances that affected less than 75% of the pixel area. For tree cover gain pixels, this proportion of sub-pixel recovery events was 61%. High spatial resolution data are the only means for consistent interpretation of such sub-pixel change events. Our annual change detection results (figure 6), even restricted to the areas where Landsat indicated possibility of tree cover change, may be affected by inconsistent detection of small-scale disturbances during early years of the analyzed time interval. When all samples are considered, including samples from the 'core no tree cover loss' stratum, the estimated average annual gross tree cover loss area is twice higher during the 2008–2014 interval (22 500 ha yr−1) compared to the 2001–2007 interval (10 200 ha yr−1). The differences is primarily related to the loss area estimated within 'core no tree cover loss' stratum. Tree cover change within this stratum is usually sub-pixel. Out of the 18 samples within this stratum where change were detected, 12 have change fraction below 50% of the pixel area. Such change events may only be detected if consistent high spatial resolution data is available for every sample and every year.
In addition to the unequal annual image availability, not all of the high-resolution imagery was collected during the growing season, which posed problems with interpretation of deciduous tree cover extent and change. The interpretation of time-series of images collected by different sensors and at different off-nadir angles and in different seasons was challenging due to the inconsistent representation of the same land cover type in such images. Finally, high-resolution data on Google Earth is not always perfectly co-registered, compromising small-scale change detection.
Using data specifically collected for validation instead of available on Google Earth may be beneficial for the operational national reporting. The experience of Ministry of Environment of Peru (MINAM) that implemented similar forest monitoring protocol developed by the GLAD team (Potapov et al 2014) proves this point. For the stratified sample assessment, MINAM purchased 30 RapidEye images that cover only 0.5% of the country area. Yet, these data were sufficient to estimate the national area of tree canopy cover and change with low uncertainty (standard error of 6.6% of the estimated change area). A similar approach based on two-stage cluster sampling may be recommended for Bangladesh. The high-resolution images may be collected for the sample units (e.g. blocks of pixels) allocated using a historical tree cover change map as a stratifier. Repeated coverage of high-resolution data for these samples every one or two years will be sufficient to estimate national tree cover change area. Improvements of the commercial image distribution systems, like availability of data purchase for small areas, or 'image renting', will be beneficial for developing countries that have limited funds for large-scale data purchase.
Detecting tree cover and change in Bangladesh was challenging even though high-resolution time-series were available for most of the years. Repeated forest disturbance and regrowth observed within timber plantations (see figure 2) and shifting cultivation areas complicates image interpretation. Small-scale changes due to removal or growth of a few trees within sampled area posed specific challenges (figure 8; table 5). The tree canopy change detection model at the Landsat scale was not capable to detect partial clearing of tree cover within sample block (loss samples 1 and 2). Urban areas expansion (loss sample 3, located within Dhaka) caused the reduction in sparse tree canopy cover that was intermixed with rural housing units hampering change interpretation. Selective tree removal within tree plantations (loss sample 4) is particularly challenging to interpret, especially if tree canopy cover have been restored quickly after the disturbance. The tree cover gain interpretation may be even more challenging if it happened within a small portion of the sample block. None of the gain samples 1–3 has an indication of tree cover expansion from the Landsat-based tree cover change strata map. These samples represent a partial and gradual expansion of planted tree cover within the Landsat pixel. The gain sample 4 shows the case of tree canopy expansion as a consequence of rural settlement growth. It also demonstrates that the gain of tree canopy by 25% within one Landsat pixels may be attributed to the growth of only a few trees. Another interpretation issue was related to the separation of trees and shrubs within areas of shifting cultivation and dry forest (e.g. the distinction between tree regeneration and shrub encroachment). In many cases, the quality of interpretation depended on analyst knowledge of local land cover types and management practices.
Table 5. Locations and interpretation results for selected sample blocks shown in the figure 8.
Tree cover loss examples | |||
---|---|---|---|
Sample # | Longitude (decimal degrees) | Latitude (decimal degrees) | Tree cover loss, % |
1 | 92.085375 | 22.707875 | 50 |
2 | 92.419875 | 25.022625 | 10 |
3 | 90.417625 | 23.864375 | 25 |
4 | 91.822875 | 23.130875 | 25 |
Tree cover gain examples | |||
Sample # | Longitude (decimal degrees) | Latitude (decimal degrees) | Tree cover gain, % |
1 | 89.528375 | 25.122125 | 50 |
2 | 90.071125 | 24.742125 | 10 |
3 | 91.607625 | 24.460875 | 25 |
4 | 90.292875 | 23.885625 | 25 |
Figure 8. Examples of high-resolution data available from Google Earth and temporal profiles of the tree canopy cover derived from the annual Landsat data time-series for selected tree cover loss and gain samples. Sampled Landsat pixel (30 × 30 meters) outlined in yellow. Sample location and interpretation result shown in table 5.
Download figure:
Standard image High-resolution imageWhile the crowd-source solutions were proposed and implemented for the sample-based analyses (Fritz et al 2009), such approaches may not be suitable in our specific case. Using multiple interpretations from non-specialists may impede the output as incorrect interpretations may prevail. In contrast, our sample interpretation approach was based on finding the consensus between experts that have deep knowledge of image interpretation and the regional geography. We suppose that the consensus approach is faster, more reliable, and less prone to errors compared to the data collection using crowdsourcing.
Our overarching goal was to establish the capacity of the RIMS unit for operational tree canopy cover monitoring. The outputs of this project provide baseline national tree cover and change area estimates with known uncertainties. In addition, this project helped RIMS to establish computing infrastructure and analyst capacity for satellite data processing. In the future, RIMS is going to implement bi-annual tree cover change area estimate update. For each bi-annual update, the RIMS unit will prepare the wall-to-wall tree cover change maps using Landsat metrics provided by the GLAD team. Change detection results will be used to create tree cover change strata for sample allocation. Resulting samples will be visually interpreted using subsets of Landsat 16 days image composites and high-resolution images in Google Earth, similarly to the process performed for the 2000–2014 change assessment. Such approach will allow RIMS to provide consistent bi-annual estimates of tree cover change with known uncertainties.
Appendix: Technical details on national tree canopy cover and change quantification
A.1. National wall-to-wall mapping and change detection
A.1.1. Landsat data processing
We used the entire Landsat archive for Bangladesh provided by the United States Geological Survey National Center for Earth Resources Observation and Science (USGS EROS). Overall, the Landsat archive for Bangladesh contains 7225 terrain corrected (processing level 1 T) images from the year 1999 to 2014. We processed each image separately following the established automated procedure (Potapov et al 2012). First, image data collected by different Landsat sensors (Landsat Thematic Mapper, Enhanced Thematic Mapper+, and Operational Land Imager) were transformed to the top-of-atmosphere spectral reflectance using sensor-specific equations (Chander et al 2009). The unitless reflectance values with the range from 0 to 1 were scaled to range from 1 to 40 000 to ensure consistency between sensors. Second, we applied a set of pre-defined decision tree models that produce a per-pixel likelihood of observation contamination by clouds, haze, or cloud shadows. Decision tree models were developed for the global forest cover change project (Hansen et al 2013) and performed well for the national data processing. The per-pixel observation quality was recorded as a separate image layer. Observations (pixels) with high likelihoods of cloud or cloud shadow contamination were excluded from the further processing. Third, we implemented a relative reflectance normalization using the global MODIS-derived surface reflectance normalization target (Hansen et al 2013). Normalization allowed us to reduce effects of atmospheric scattering and surface anisotropy, and to create spatially and temporally consistent inputs that are suitable for classification model implementation at the national scale (Potapov et al 2012, Potapov et al 2014). On average, each land pixel within Bangladesh has 113 cloud/shadow-free Landsat observations from the year 2000 to 2014, or 7.6 observations per year.
To simplify reflectance time-series analysis, we transformed the normalized reflectance data from individual Landsat images into a set of 16 days image composites. The start/end date of each composite was selected consistently with standard 16 days MODIS Level 3 G products (Huete et al 1999). Four reflectance bands (red, near infrared, and two shortwave infrared bands), the emissive thermal infrared band, and the observation quality layer were used for compositing. The compositing was performed per-pixel, with a data format consistent with the global forest cover change product (Hansen et al 2013). During compositing, an observation with the highest quality (according to the observation quality layer) for each pixel was retained as the output composite value. The 16 days Landsat input data served as a spatially and temporally consistent input to analyze time-series and facilitate fast extraction of spectral properties of land cover for any specific time and location. This data format also allowed us to reduce input data volume that has to be processed by the RIMS Unit. While source national Landsat archive data volume totaled 5 Terabytes (TB), including 2 TB of source imagery and 3 TB of normalized reflectance data, the data volume for 1999–2014 16 days composites was just over 1 TB.
A.1.2. The year 2000 >50% tree cover extent stratum
To create input data for the year 2000 tree cover extent mapping, we employed Landsat data collected from 1999 to 2001. Three years of observations allowed us to create a consistent, cloud-free national dataset, which would be impossible to obtain using a single year of observations. Multi-temporal metrics were derived from the input 16 days time-series data from the three-year time interval. Metrics are statistical derivatives of time-sequential input data that provide a generalized feature space while retaining salient phenological features (Hansen et al 2005) To calculate metric values for each pixel, spectral data from cloud-free observations were ranked based on: (i) band reflectance value; (ii) normalized difference vegetation index (NDVI, Tucker 1979); (iii) normalized difference water index (NDWI, Xiao et al 2004); (iv) and thermal infrared band values. From each of these ranks, a set of statistics were recorded, including: (i) minimum, 10th, 25th, 50th, 75th, 90th percentile, and maximum values; (ii) averages between these percentiles (e.g. from minimum to 10th percentile, from 10th to 25th percentile, etc.). The output values were calculated for the reflective bands, NDVI, and NDWI. In addition to multi-temporal spectral metrics, we used topographical data (elevation, slope, and aspect) derived from the void-filled seamless Shuttle Radar Topography Mission digital elevation model (http://srtm.csi.cgiar.org).
We employed a supervised classification decision trees algorithm to create the >50% tree cover extent stratum (Breiman et al 1984). Decision tree classifiers are based on an analyst-defined training set. At the Landsat spatial resolution, we interpreted training data for two classes: (1) greater than or equal to 50% tree crown cover, and (2) less than 50% canopy cover. We used the year 2000 Landsat cloud-free image composite and the high spatial resolution data available from Google Earth to interpret training sites. To prevent overfitting of the model to the training data, we implemented a decision tree ensemble model known as 'bagging', or bootstrap aggregation (Breiman 1996). The output map was created by applying an ensemble of seven trees, each based on a 20% random sample of pixels from the training population, and calculating the median class likelihood from the tree outputs. The training set was created using the iterative 'active learning' procedure (Tuia et al 2009). After creating an initial set of training, the analyst assessed classification results, correctly labeled examples of classification errors, and added them to the training set. The process was repeated until the quality of the >50% tree cover extent stratum map was sufficient as judged by the image analyst. The final training set consisted of more than 640 000 pixels.
A.1.3. Tree cover loss and gain 2000–2014 strata
Multi-temporal spectral metrics that served as inputs for the tree cover change mapping were derived using the approach developed for the global forest monitoring (Hansen et al 2013). 16 days cloud-free observations time-series for the years 1999 to 2014 were converted into a set of metrics that represent spectral reflectance change in time. Spectral reflectance, NDVI, NDWI indices values and thermal infrared band values were ranked, and the statistics were calculated using the same approach as the one presented in section A.1.2. To highlight the reflectance change within time-series, additional metrics were calculated including: (i) slope of linear regression between reflectance/index value and observation date; (ii) maximum reflectance/index value difference between consecutive 16 days composites; (iii) reflectance/index value gain/drop amplitude; and (iv) earliest and latest cloud-free observations within time-series. Topographic metrics were also used as input features.
Gross tree cover loss and gain strata mapping were performed independently, using separate supervised classification models (bagged decision trees). Both models were based on analyst-defined training sets created iteratively. Training pixels were interpreted using national mosaics of selected metrics, including the year 2000 and 2014 image composites, maximum reflectance value composite, and slope of linear regression for selected spectral bands. In total, more than 200 000 training pixels were used for the loss classification and almost 400 000 for the gain classification. Due to the ambiguity of small-scale tree canopy cover change interpretation at Landsat scale, only relatively large, (at least nine Landsat pixels) unambiguous areas of tree cover change were used as training data. Thus, we expected that both classification models might have low sensitivity to small-scale, sub-Landsat-pixel change events. After classification, we removed change polygons consisting of a single pixel. Single pixels of loss and gain mostly represented noise as was judged by the image interpretation analysts.
A.2. Sample-based assessment
We quantify national tree cover extent for the year 2000 and change from 2000–2014 using the sample data. Tree cover for the year 2000, gross tree cover loss and gain areas were estimated separately, using independently selected and analyzed samples.
A.2.1. Sample allocation and response design
To estimate tree cover for the year 2000, we allocated a random sample of 1000 30 × 30 m pixels using the >50% tree cover extent stratum map (see section A.1.2). To account for the pixels with an intermediate proportion of canopy cover often located at the boundaries of areas with dense tree cover, we created the following sampling strata from the map:
- 1.'core tree cover areas', including >50% tree cover stratum pixels that do not have any treeless neighbors;
- 2.'core treeless areas', including pixels outside >50% tree cover stratum that do not have any tree cover stratum neighbors;
- 3.'periphery tree cover areas', including >50% tree cover stratum pixels that have treeless neighbors;
- 4.'periphery treeless areas', including pixels outside >50% tree cover stratum that have neighboring tree cover stratum pixels.
The number of sampled pixels in each stratum was between proportional and equal sampling allocations (table A1, A). Sampled pixels were interpreted using Landsat data for the year 2000 and high-resolution data from Google Earth. We recorded percent tree canopy cover per sample pixel in 5% increments, as well as forest type (for pixels with trees). The RIMS Unit adopted a simplified forest type system following the national forest assessment 2006–2007 and the country report to the FAO FRA (FAO 2014). The following forest types were considered:
- Hill forests: tropical evergreen and semi-evergreen forests in Chittagong Hill Tracts and Sylhet region. Hill forests include slash-and-burn agriculture mosaic areas, and naturally regrown trees matching height criterion.
- Sal forests: moist deciduous forests dominated by Shorea robusta. Since nearly all sal forests are managed, this forest type may include mature tree plantations as well.
- Swamp forests: freshwater wetland forests, mostly located in Sylhet region.
- Mangroves: tidal saltwater forests on the coast and within river estuary. Mangrove plantations were included in the same category.
- Trees outside forests: all planted trees, including industrial timber plantations, agroforestry, village woodlots, orchards, and other plantation types (except mangroves).
The sample-based analysis of gross tree cover loss and gain was performed similarly to the analysis of tree cover. The sampling design was based on the Landsat-based gross tree cover loss and gain strata. For each change type, we selected strata that represent 'core' areas of change and no change, and 'periphery' areas that include boundary pixels. Initially, two independent samples of 1000 pixels were randomly selected from these strata for loss and gain map validation. After initial sample-based result assessment, additional sample pixels were selected to increase the precision of the national-scale change area estimate. The final sampling design (including additional sample pixels) is presented in table A1, B and C. To interpret each sample pixel, the following information was provided to the analyst: (i) annual cloud-free Landsat image composites; (ii) temporal profiles of annual minimum NDVI, maximum annual shortwave infrared reflectance, and annual tree canopy cover estimated from Landsat time-series data using the global canopy cover model (Hansen et al 2013); and (iii) Google Earth high-resolution imagery time-series (figure 2). The following information was collected for each sample pixel for gross tree cover loss analysis:
- Percent tree cover loss, with 5% increment;
- The year of the loss (in the case of multiple loss events, only the first date was recorded);
- Forest type for the year 2000 (before the loss event);
- Percent tree cover restoration by the year 2014 (with 5% increments);
- Forest type for the year 2014 (in the case of gain event).
For the gross tree cover gain analysis, we recorded the following information for each sample pixel:
- Percent tree cover gain, with 5% increment;
- Forest type for the year 2014 (in the case of gain event).
The year of tree cover gain was not recorded as tree cover gain consists of a continuous process, unlike tree cover loss, making assignation of a particular year difficult even when using high spatial resolution data.
Sample interpretation was done by the RIMS Unit and the GLAD Lab teams sequentially. The objective of this procedure was to interpret each sample by aggregating all available information on land cover and land use management practices for each particular area. For each interpretation round, only samples where interpreters disagreed were reviewed. The most complicated cases were discussed during the joint workshop. The process was completed when all interpretation experts from both teams agreed on each sample interpretation result.
Table A1. Stratified sampling design to estimate tree canopy cover for the year 2000 and tree cover change, 2000–2014. Samples A, B and C are independent from each other.
Stratum | Area, thousand ha | Count, pixels | % total area | Sample size (pixels) |
---|---|---|---|---|
A. Tree cover 2000 | ||||
Core tree cover areas | 1337.2 | 18 820 603 | 8.9 | 200 |
Periphery tree cover areas | 1324.3 | 18 726 681 | 8.8 | 200 |
Core treeless areas | 10639.5 | 150 956 982 | 71.2 | 400 |
Periphery treeless areas | 1666.7 | 23 605 677 | 11.1 | 200 |
Total sample pixels | 1000 | |||
B. Gross tree cover loss | ||||
Core loss | 34.2 | 480 602 | 0.2 | 128 |
Periphery loss | 161.3 | 2 272 793 | 1.1 | 275 |
Core no loss | 14476.8 | 205 192 754 | 96.7 | 824 |
Periphery no loss | 295.4 | 4 163 794 | 2.0 | 279 |
Total sample pixels | 1506 | |||
C. Gross tree cover gain | ||||
Core gain | 17.5 | 245 952 | 0.1 | 122 |
Periphery gain | 98.1 | 1 383 985 | 0.7 | 273 |
Core no gain | 14627.6 | 207 311 191 | 97.7 | 821 |
Periphery no gain | 224.5 | 3 168 815 | 1.5 | 270 |
Total sample pixels | 1486 |
A.2.2. Sample-based area estimation
The sample-based area estimation was performed following Stehman et al (2014). Equation 1 was used to estimate the area of tree cover in the year 2000, and tree cover loss and gain in 2000–2014 from the sample interpretation results. The same equation was used to estimate tree cover and change for each forest type, and by the year of disturbance.
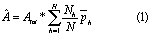
Atot—total country area;
H—number of sampling strata;
N—total number of pixels in the country;
nh—sample size (number of sampled pixels) in stratum h;
Nh—total number of pixels in stratum h;
, mean proportion of tree cover 2000/tree cover loss 2000–2014/tree cover gain 2000–2014 in stratum h, where pu is the proportion of a sampled pixel covered with tree cover in the year 2000, or having experienced tree cover loss or gain in 2000–2014 (pu ranges from 0.0 to 1.0 with 0.05 (5%) increment).
Equation 2 was used to estimate the standard error of the sample-based estimate of the year 2000 tree cover, and 2000–2014 tree cover loss and gain. The 95% confidence interval was obtained by multiplying standard error by 1.96.
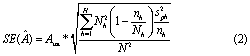
, sample variance for stratum h.
Net tree cover change area was derived from the sample-based estimates of gross loss and gain areas for each forest type separately and for the entire tree cover area. To estimate net tree cover change, we subtracted gross tree cover loss from the area of gross tree cover gain and tree cover restoration within gross loss areas (equation 3). The standard error was estimated using the error propagation approach (equation 4).

Cn—net tree cover change;
Gg—gross tree cover gain;
Gl—gain of tree cover within areas of tree cover loss;
Lg—gross tree cover loss.

SE — standard error.