Abstract
To improve the detection level of aggregate shape for automated road use, Per-Optuna-LightGBM model for aggregate shape classification is proposed. Collect aggregate images using industrial camera and extract 48 morphological feature parameters. A feature importance analysis method based on Spearman Correlation and Permutation Importance is proposed to remove redundant factors and select the feature parameters of aggregate morphology. Based on cross-validation, an optimized Optuna-LightGBM model is trained based on the constructed dataset. Compared with GS-XGBoost algorithm, the Optuna-LightGBM model can classify the shape of aggregates more accurately and efficiently. The accuracy value of the proposed model is 82.5%, which increased by 4% compared to before optimization. The proposed model can efficiently classify the shape of aggregates which meet the design requirements, also provide a certain foundation for automated classification of aggregate shapes.
Export citation and abstract BibTeX RIS
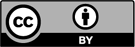
Content from this work may be used under the terms of the Creative Commons Attribution 3.0 licence. Any further distribution of this work must maintain attribution to the author(s) and the title of the work, journal citation and DOI.