Abstract
Rain will significantly lower image quality, which will undoubtedly have an impact on how well outdoor computer vision systems operate, such as autonomous driving. This paper proposes a two-branch deep neural network consisting of an attention guided U-Net and Vision Transformer, which could capture intra-field details and cross-patch relationships to obtain good global and local deraining effects. In order to ensure both branches pose positive effects on the target derain task, we specifically design a patch boot image module to achieve complemented feature fusion, which adaptively selects informative intra-field regions guided by the patch-wise importance map. Moreover, Wasserstein distance between the prediction and reference image is applied as the objective function to pursue better image quality measurement. In comparison to existing algorithms, the suggested method may more effectively remove rain and restore the background details, according to qualitative and quantitative results on the public datasets Rain200L and Rain200H. On the aforementioned datasets, the peaks of the structural similarity (SSIM) and signal-to-noise ratio (PSNR) were 32.55/0.9476 and 26.12/0.8826, respectively.
Export citation and abstract BibTeX RIS
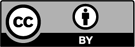
Content from this work may be used under the terms of the Creative Commons Attribution 3.0 licence. Any further distribution of this work must maintain attribution to the author(s) and the title of the work, journal citation and DOI.