Abstract
The accurate prediction of power system load is extremely important for the operation of the power market and the safe operation of the power grid. In order to improve the accuracy of short-term load forecasting of power systems, a combination model based on long short term memory network (LSTM) and light gradient boosting machine (LightGBM) is proposed. The experiment first decomposes historical load data by EMD, uses historical weather data and load data decomposed by EMD to establish LSTM prediction model and LightGBM prediction model respectively, and then these two predicted values are linearly combined to obtain the final predicted value. The electrical load data of the 2016 Electrician Mathematical Contest in Modeling is used as an example to verify. The experimental results show that the LSTM-LightGBM combined model has higher forecasting accuracy and application prospects for power load forecasting than traditional load forecasting methods and standard LSTM and LightGBM load forecasting methods.
Export citation and abstract BibTeX RIS
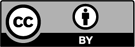
Content from this work may be used under the terms of the Creative Commons Attribution 3.0 licence. Any further distribution of this work must maintain attribution to the author(s) and the title of the work, journal citation and DOI.