Abstract
Vacuum degree is a crucial factor for the operation of thermoelectric generating set. Existing approaches typically use machine learning algorithms to link the relationship between unit operating data and condenser vacuum degree by focusing on the temporal information within the data, while ignoring the frequency information implied in the historical condenser vacuum degree. To fully use of frequency information and further improve prediction accuracy, we propose a novel condenser vacuum degree prediction model with multi-view information fusion. In specific, the implicit frequency information in the historical vacuum degree sequence is explored via a combination of Variational Mode Decomposition (VMD) and Convolutional Neural Network (CNN). Furthermore, Transformer encoder is used to extract the temporal information from the unit operating data. Finally, the two views information are fused for condenser vacuum degree prediction. Extensive experiments conducted on the real data collected from a power plant demonstrate the superiority of the proposed method over several state-of-the-art methods.
Export citation and abstract BibTeX RIS
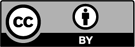
Content from this work may be used under the terms of the Creative Commons Attribution 3.0 licence. Any further distribution of this work must maintain attribution to the author(s) and the title of the work, journal citation and DOI.