Abstract
Many statistical methods are very sensitive to data containing outliers and heavy tails, and simply eliminating these data often does not achieve the desired results. We usually need to do some data transformation to make it approximately follow a normal distribution. But not all data can be transformed into a normal distribution, and then we can only adjust the shape of its data distribution to make its shape close to a normal distribution. The kurtosis of the distribution can better reflect the peakedness or flatness of the distribution. So in this paper, I propose a nonfunctional data transformation approach to improve the efficiency of statistical methods by continuously adjusting the kurtosis of the data while maintaining the distribution of the data. I apply the transformed data to SVM classification, and the numerical results show that the transformed data by my method performs significantly better than the untransformed data, as well as better than other comparable methods.
Export citation and abstract BibTeX RIS
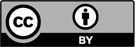
Content from this work may be used under the terms of the Creative Commons Attribution 3.0 licence. Any further distribution of this work must maintain attribution to the author(s) and the title of the work, journal citation and DOI.