Abstract
This paper presents an effective Self-Knowledge Distillation (SKD) framework via Atrous Spatial Pyramid Structure (ASPS), which is able to enhance the performance of the object segmentation network without increasing network parameters. In the framework, a lightweight object segmentation network is constructed to achieve the pixel-level object segmentation efficiently. A SKD learning model, including the SKD representation structure based on ASPS and loss function, is proposed to transfer the knowledge in the ASPS into our object segmentation network and improve its generalization ability. The experimental results confirm that compared with recent typical object segmentation networks, our object segmentation network contains the fewest parameters but achieves better performance. Moreover, the proposed SKD method achieves the best performance-boosting compared with recent SKD methods
Export citation and abstract BibTeX RIS
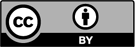
Content from this work may be used under the terms of the Creative Commons Attribution 3.0 licence. Any further distribution of this work must maintain attribution to the author(s) and the title of the work, journal citation and DOI.