Abstract
The fuzzy broad learning system (FBLS) is a novel, neuro-fuzzy model. Different from other neuro-fuzzy models with low efficiency, FBLS can obtain better performance using less computation time. However, the clustering-based fuzzy rule generation approach makes the performance of FBLS limited. Meanwhile, it is unknown how the enhancement layers from FBLS contribute to the model performance, which hinders the further extension of the model structure. To solve these problems, we propose a sparse enhancement fuzzy broad learning system (SEFBLS). It uses only a sparse set of enhancement nodes to replace the original enhancement node groups. To obtain a better representation, the designed principal component-based sparse autoencoder is used for feature reconstruction and information removal. In addition, to explore the optimal model structure and performance, multiple clustering methods (fuzzy and non-fuzzy) are used to improve SEFBLS. The results on 10 UCI classification datasets show that the proposed SEFBLS obtains competitive accuracy using fewer fuzzy rules.
Export citation and abstract BibTeX RIS
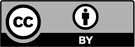
Content from this work may be used under the terms of the Creative Commons Attribution 3.0 licence. Any further distribution of this work must maintain attribution to the author(s) and the title of the work, journal citation and DOI.