Abstract
There are many kinds of inverse kinematics solutions for robots. Deep reinforcement learning can make the robot spend a short time to find the optimal inverse kinematics solution. Aiming at the problem of sparse rewards in the process of deep reinforcement learning, this paper proposes an improved PPO algorithm. Firstly, built a simulation environment for the operation of the robotic arm. Secondly, use a convolutional neural network to process the data read by the camera of the robotic arm, obtaining a network about Actor and Critic. Thirdly, based on the principle of inverse kinematics of the robotic arm and the reward mechanism in deep reinforcement learning, design a hierarchical reward function containing motion accuracy to promote the convergence of the PPO algorithm. Finally, compare the improved PPO algorithm with the traditional PPO algorithm. The results show that the improved PPO algorithm has improved both the convergence speed and the operating accuracy.
Export citation and abstract BibTeX RIS
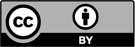
Content from this work may be used under the terms of the Creative Commons Attribution 3.0 licence. Any further distribution of this work must maintain attribution to the author(s) and the title of the work, journal citation and DOI.