Abstract
Most current knowledge base question answering models mainly use RNN and its various derivative versions such as BiLSTM to model the problem, which limits Parallel computing capabilities of the model. In response to this problem, we try to use TransformerEncoder instead of BiLSTM to model and encode the problem, and hope to improve the parallel computing efficiency of the model. At the same time, to solve the problem of insufficient relative position information obtained by using absolute position coding in TransformerEncoder, it is proposed to use relative position coding instead of absolute position coding. According to the experimental results, our model effectively reduces a certain amount of training time and has achieved certain results on the WebQuestions benchmark data set.
Export citation and abstract BibTeX RIS
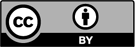
Content from this work may be used under the terms of the Creative Commons Attribution 3.0 licence. Any further distribution of this work must maintain attribution to the author(s) and the title of the work, journal citation and DOI.