Abstract
For modern operation and maintenance systems, they are usually required to monitor multiple types and large quantities of machine's key performance indicators (KPIs) at the same time with limited resources. In this paper, to tackle these problems, we propose a highly compatible time series anomaly detection model based on K-means clustering algorithm with a new Wavelet Feature Distance (WFD). Our work is inspired by some ideas from image processing and signal processing domain. Our model detects abnormalities in the time series datasets which are first clustered by K-means to boost the accuracy. Our experiments show significant accuracy improvements compared with traditional algorithms, and excellent compatibilities and operating efficiencies compared with algorithms based on deep learning.
Export citation and abstract BibTeX RIS
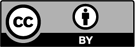
Content from this work may be used under the terms of the Creative Commons Attribution 3.0 licence. Any further distribution of this work must maintain attribution to the author(s) and the title of the work, journal citation and DOI.