Abstract
Malaria is a very serious disease that caused by the transmitted of parasites through the bites of infected Anopheles mosquito. Malaria death cases can be reduced and prevented through early diagnosis and prompt treatment. A fast and easy-to-use method, with high performance is required to differentiate malaria from non-malarial fevers. Manual examination of blood smears is currently the gold standard, but it is time-consuming, labour-intensive, requires skilled microscopists and the sensitivity of the method depends heavily on the skills of the microscopist. Currently, microscopy-based diagnosis remains the most widely used approach for malaria diagnosis. The development of automated malaria detection techniques is still a field of interest. Automated detection is faster and high accuracy compared to the traditional technique using microscopy. This paper presents an exhaustive review of these studies and suggests a direction for future developments of the malaria detection techniques. This paper analysis of three popular computational approaches which is k-mean clustering, neural network, and morphological approach was presented. Based on overall performance, many research proposed based on the morphological approach in order to detect malaria.
Export citation and abstract BibTeX RIS
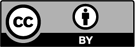
Content from this work may be used under the terms of the Creative Commons Attribution 3.0 licence. Any further distribution of this work must maintain attribution to the author(s) and the title of the work, journal citation and DOI.