Abstract
A timely identification of an anomalous functioning of the energy system of an industrial building would increase the efficiency and the resilience of the energy infrastructure, beside reducing the economic wastage. This work has been inspired by the need of identifying, for a series of supermarket buildings in Switzerland, the failures happening in their heating systems across the years in an unsupervised and easy-to-visualize fashion for the building managers. The lack of any a-priori label differentiating between typical and anomalous behaviors calls for the usage of unsupervised machine learning methods to extract the relevant features to describe the system operations, to reduce the dimension of the feature space, and to cluster together similar patterns of operations. The method is validated on a standard supermarket building, where it successfully discriminates winter and summer operations from periods of refurbishment or malfunctioning of the heating system.
Export citation and abstract BibTeX RIS
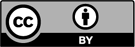
Content from this work may be used under the terms of the Creative Commons Attribution 3.0 licence. Any further distribution of this work must maintain attribution to the author(s) and the title of the work, journal citation and DOI.