Abstract
Real-time monitoring of the aquaculture water state is essential for shrimp aquaculture, and it plays a vital role in the growth of shrimp. The main purpose of this research is to find an effective method to achieve rapid detection and classification with water state images. We obtained a water state image dataset containing four categories in the laboratory, and gained the corresponding Hough datasets through Hough transform, including blue-green and turbid water, reddish brown and turbid water, light green and clear or tawny and clear water, colorless and transparent water. We compare the performance and spatio-temporal complexity of 12 widely used convolutional neural network models in water state image classification tasks, and then optimize the best performing model on the optimizer and hyperparameters. The experimental results show that the InceptionResNet model has the best effect and the InceptionV3 model has the smallest spatio-temporal complexity, while both models realize the task of water state image classification excellently.
Export citation and abstract BibTeX RIS
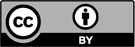
Content from this work may be used under the terms of the Creative Commons Attribution 3.0 licence. Any further distribution of this work must maintain attribution to the author(s) and the title of the work, journal citation and DOI.