Abstract
The depth accurately estimated from the RGB image can be used in applications such as 3D reconstruction and scene display. In depth estimation, Convolutional Neural Network (CNN) plays an important role. However, most of the existing CNN-based depth estimation methods often do not take full advantage of the space and channel information in the local receptive field, resulting in lower-resolution depth maps. Based on the extant Encoder-Decoder network architecture, a simple and effective attention module is introduced to learn the discerning feature and the semantic feature in this paper. First, the intermediate feature map is generated by the encoder, and then the attention module is used to generate the attention maps along the two dimensions of the space and channel of the feature map. Finally, the refined output generated by multiplying the attention maps to intermediate feature maps is input to the subsequent neural network for further work. Our works show that the suggest approach has obtained good achievements on NYU Depth v2.
Export citation and abstract BibTeX RIS
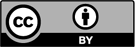
Content from this work may be used under the terms of the Creative Commons Attribution 3.0 licence. Any further distribution of this work must maintain attribution to the author(s) and the title of the work, journal citation and DOI.