Abstract
Small object detection is a challenging research direction in the field of computer vision, due to the low resolution and restricted information of small objects. At present, the general detectors only use appearance features to classify and locate objects, but they are prone to failure under the interference of background noise. On the other hand, the detector based on deep neural network has excellent performance on large scale, but it is difficult to extract enough information of small objects. This paper proposes a feature enhancement network (FENet), which contains two modules. The Residual feature enhancement (RFE) module combines residual learning and sub-pixel convolution to improve the resolution of input small objects and remove image noise. The Attention Feature Pyramid (AFP) module integrates the feature pyramid and attention mechanism, which can extract context information and filter redundant context information. At the same time, considering the imbalance of the contribution of large and small objects to the loss function during the training process, a feedback-driven function is introduced to solve the problem of uneven loss under multiple scales. Experimental results show that compared with the existing small object detection methods, our method has better performance.
Export citation and abstract BibTeX RIS
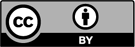
Content from this work may be used under the terms of the Creative Commons Attribution 3.0 licence. Any further distribution of this work must maintain attribution to the author(s) and the title of the work, journal citation and DOI.