Abstract
Linear-kernel Support Vector Machine (SVM) classification algorithm needs to constantly calculate the similarity between the data to be tested and the sample during classification. If samples in the dataset are linearly indivisible, the accuracy of the algorithm will get worse. The feature weight calculation based on category information in Multi-layer Perceptron (MLP) algorithm is not accurate enough to express the relationship between features and categories, i.e. it cannot distinguish categories precisely when the features with the same category frequency. In order to improve the performance of MLP and linear-kernel SVM algorithms in classification, an improved function: the Base function, which is based on clustering, is proposed in this paper. Before running the classification algorithm, a series of possible centers is predicted, after which the Base function is used for data clustering to increase the dimension of data, and then samples with more features are passed to classification models. In the high-position Gaussian data space, the Base function can obviously improve the spatial distribution of samples. Experimental comparison in the thesis also proved that the Base function can improve the performance of MLP or SVM in classification effectively. The proposed feature-enhanced MLP is superior to the baseline with 32.6% accuracy promotion on the Gaussian dataset.
Export citation and abstract BibTeX RIS
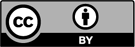
Content from this work may be used under the terms of the Creative Commons Attribution 3.0 licence. Any further distribution of this work must maintain attribution to the author(s) and the title of the work, journal citation and DOI.