Abstract
The key technical challenge of 6D target attitude estimation from RGB-D image is to make full use of 2d and 3D image point cloud data. Previous traditional target pose estimation methods either extract information from RGB images and depth, respectively, or use expensive post-processing steps that limit their performance in highly cluttered scenarios and real-time applications. Therefore, in this paper, a method is proposed to estimate the 6D attitude of a group of known objects from RGB-D images. The network used is a heterogeneous architecture, which processes two data from different dimensions separately, and uses a new dense fusion network to extract pixel-level dense feature embedding, and estimate the target position and attitude from it. In addition, an end-to-end iterative attitude optimization method is integrated to further improve attitude estimation, while real-time visualization of point clouds and rendering of bounding boxes are realized. Experimental results show that this method is effective on open source data sets.
Export citation and abstract BibTeX RIS
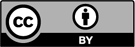
Content from this work may be used under the terms of the Creative Commons Attribution 3.0 licence. Any further distribution of this work must maintain attribution to the author(s) and the title of the work, journal citation and DOI.