Abstract
This paper analyzes the existing credit card anomaly detection classification algorithms, summarizes the parts that can be improved, and proposes an outlier detection classification algorithm based on the unsupervised algorithm and active learning decision trees. Since the 1990s, machine learning technology has been widely used in the field of credit card fraud detection. Among them, the supervised learning expert system used for classification tends to perform better than the unsupervised learning model. The good performance of supervised learning methods requires high learning costs. The training process is usually serial or partially parallel. Therefore, the computing power, time, and manual labeling cost for learning need to be considered as algorithm selection factors. However, manual labeling usually involves the counter or ATM sending information to the back-end large-value transaction authorization department for manual review, and then the employee judges whether it is an abnormal transaction based on experience. This rigorous process will produce a very large time delay. Combining this feature, this article selects the appropriate unsupervised outlier detection method, selects part of the training data, and uses a small part of the more valuable data for annotation learning. Experiments show that this method can improve the accuracy while saving the time cost of training. Compared with the training time, the added time of classification is negligible.
Export citation and abstract BibTeX RIS
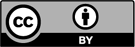
Content from this work may be used under the terms of the Creative Commons Attribution 3.0 licence. Any further distribution of this work must maintain attribution to the author(s) and the title of the work, journal citation and DOI.